Two-Stage Deep Anomaly Detection With Heterogeneous Time Series Data
IEEE ACCESS(2022)
摘要
We introduce a data-driven anomaly detection framework using a manufacturing dataset collected from a factory assembly line. Given heterogeneous time series data consisting of operation cycle signals and sensor signals, we aim at discovering abnormal events. Motivated by our empirical findings that conventional single-stage benchmark approaches may not exhibit satisfactory performance under our challenging circumstances, we propose a two-stage deep anomaly detection (T-DAD) framework in which two different unsupervised learning models are adopted depending on types of signals. In Stage I, we select anomaly candidates by using a model trained by operation cycle signals; in Stage II, we finally detect abnormal events out of the candidates by using another model, which is suitable for taking advantage of temporal continuity, trained by sensor signals. A distinguishable feature of our framework is that operation cycle signals are exploited first to find likely anomalous points, whereas sensor signals are leveraged to filter out unlikely anomalous points afterward. Our experiments comprehensively demonstrate the superiority over single-stage benchmark approaches, the model-agnostic property, and the robustness to difficult situations.
更多查看译文
关键词
Anomaly detection, Time series analysis, Manufacturing, Hidden Markov models, Autoregressive processes, Benchmark testing, Long short term memory, Anomaly detection, operation cycle, sensor, time series data, two-stage detection
AI 理解论文
溯源树
样例
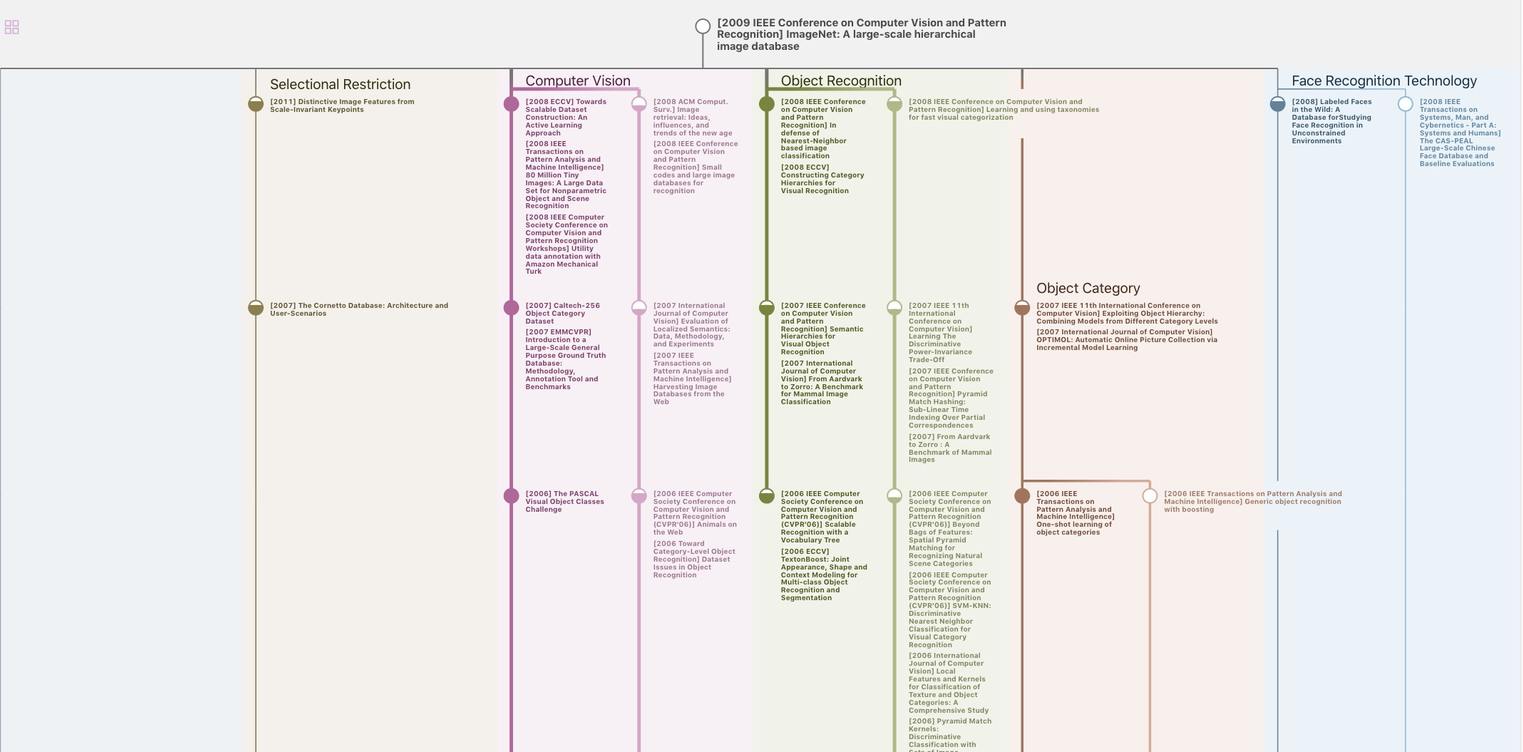
生成溯源树,研究论文发展脉络
Chat Paper
正在生成论文摘要