PINs: Progressive Implicit Networks for Multi-Scale Neural Representations.
International Conference on Machine Learning(2022)
摘要
Multi-layer perceptrons (MLP) have proven to be effective scene encoders when combined with higher-dimensional projections of the input, commonly referred to as positional encoding. However, scenes with a wide frequency spectrum remain a challenge: choosing high frequencies for positional encoding introduces noise in low structure areas, while low frequencies results in poor fitting of detailed regions. To address this, we propose a progressive positional encoding, exposing a hierarchical MLP structure to incremental sets of frequency encodings. Our model accurately reconstructs scenes with wide frequency bands and learns a scene representation at progressive level of detail without explicit per-level supervision. The architecture is modular: each level encodes a continuous implicit representation that can be leveraged separately for its respective resolution, meaning a smaller network for coarser reconstructions. Experiments on several 2D and 3D datasets shows improvements in reconstruction accuracy, representational capacity and training speed compared to baselines.
更多查看译文
关键词
progressive implicit networks,multi-scale
AI 理解论文
溯源树
样例
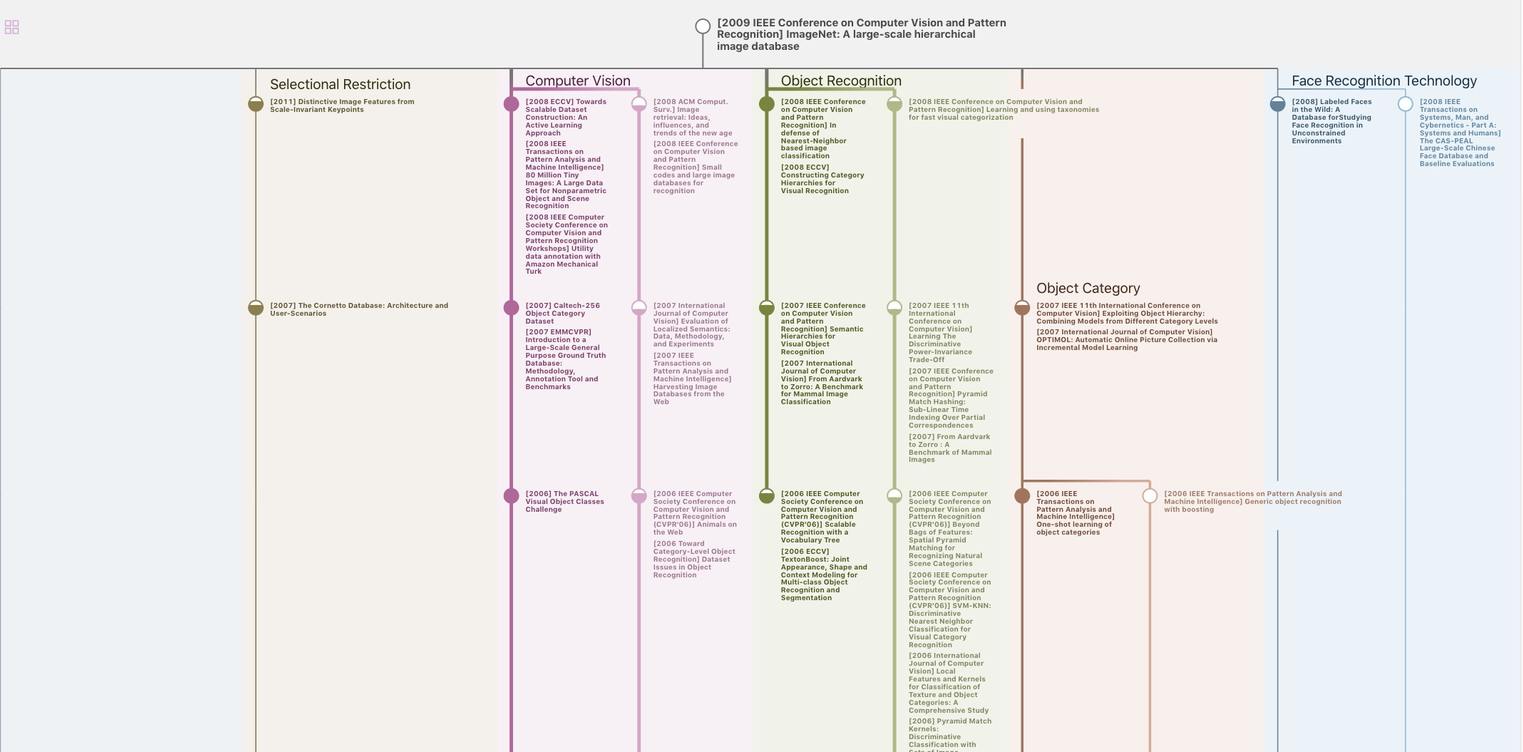
生成溯源树,研究论文发展脉络
Chat Paper
正在生成论文摘要