Non-Linear Spectral Dimensionality Reduction Under Uncertainty
arxiv(2022)
摘要
In this paper, we consider the problem of non-linear dimensionality reduction under uncertainty, both from a theoretical and algorithmic perspectives. Since real-world data usually contain measurements with uncertainties and artifacts, the input space in the proposed framework consists of probability distributions to model the uncertainties associated with each sample. We propose a new dimensionality reduction framework, called NGEU, which leverages uncertainty information and directly extends several traditional approaches, e.g., KPCA, MDA/KMFA, to receive as inputs the probability distributions instead of the original data. We show that the proposed NGEU formulation exhibits a global closed-form solution, and we analyze, based on the Rademacher complexity, how the underlying uncertainties theoretically affect the generalization ability of the framework. Empirical results on different datasets show the effectiveness of the proposed framework.
更多查看译文
关键词
uncertainty,non-linear
AI 理解论文
溯源树
样例
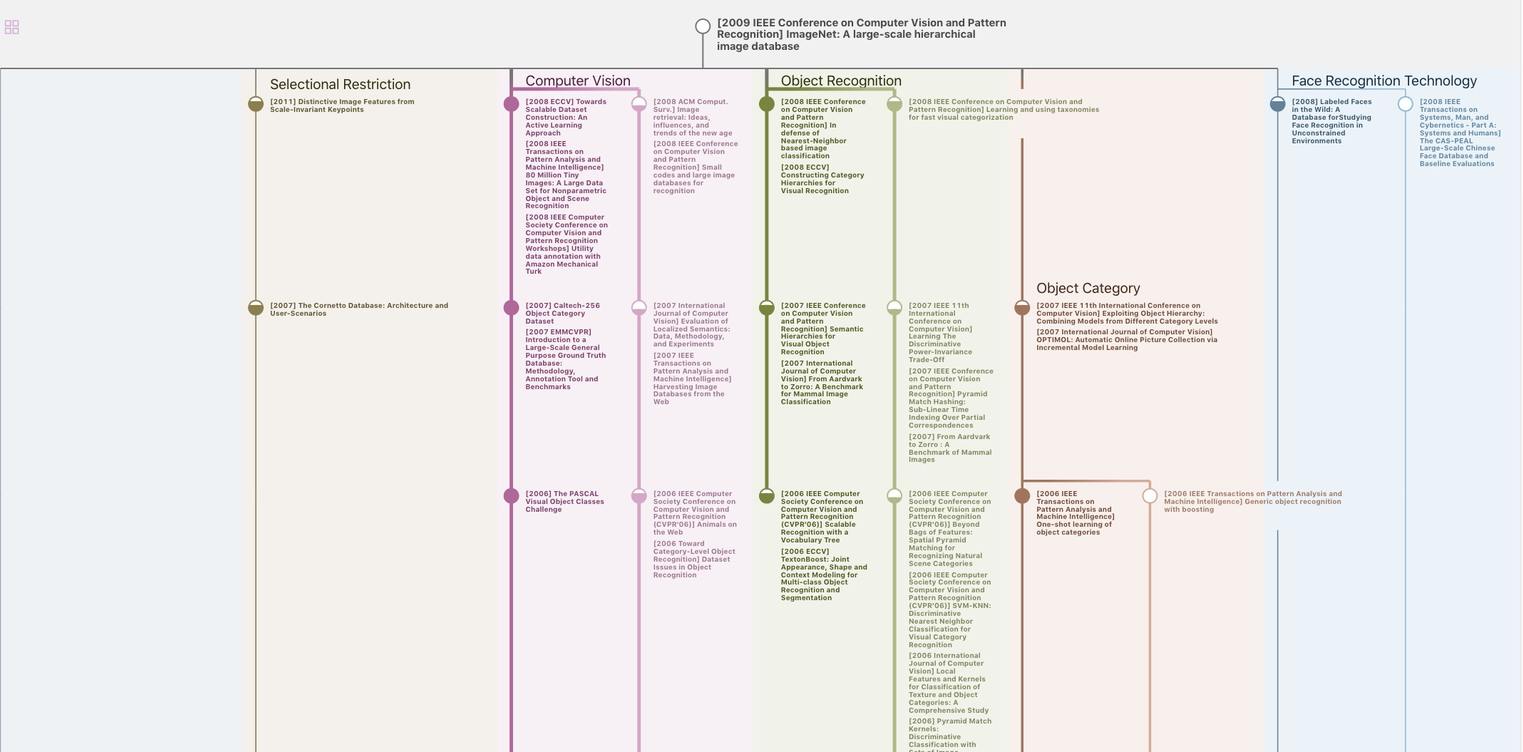
生成溯源树,研究论文发展脉络
Chat Paper
正在生成论文摘要