Statistics in the Service of Science: Don’t Let the Tail Wag the Dog
user-5dd528d2530c701191bf1b49(2022)
摘要
Statistical modeling is generally meant to describe patterns in data in service of the broader scientific goal of developing theories to explain those patterns. Statistical models support meaningful inferences when models are built so as to align parameters of the model with potential causal mechanisms and how they manifest in data. When statistical models are instead based on assumptions chosen by default, attempts to draw inferences can be uninformative or even paradoxical—in essence, the tail is trying to wag the dog. These issues are illustrated by van Doorn et al. ( this issue ) in the context of using Bayes Factors to identify effects and interactions in linear mixed models. We show that the problems identified in their applications (along with other problems identified here) can be circumvented by using priors over inherently meaningful units instead of default priors on standardized scales. This case study illustrates how researchers must directly engage with a number of substantive issues in order to support meaningful inferences, of which we highlight two: The first is the problem of coordination , which requires a researcher to specify how the theoretical constructs postulated by a model are functionally related to observable variables. The second is the problem of generalization , which requires a researcher to consider how a model may represent theoretical constructs shared across similar but non-identical situations, along with the fact that model comparison metrics like Bayes Factors do not directly address this form of generalization. For statistical modeling to serve the goals of science, models cannot be based on default assumptions, but should instead be based on an understanding of their coordination function and on how they represent causal mechanisms that may be expected to generalize to other related scenarios.
更多查看译文
关键词
Mixed models, Default Bayes Factors, Standardized effect sizes, Coordination function, Generalizability, Statistics
AI 理解论文
溯源树
样例
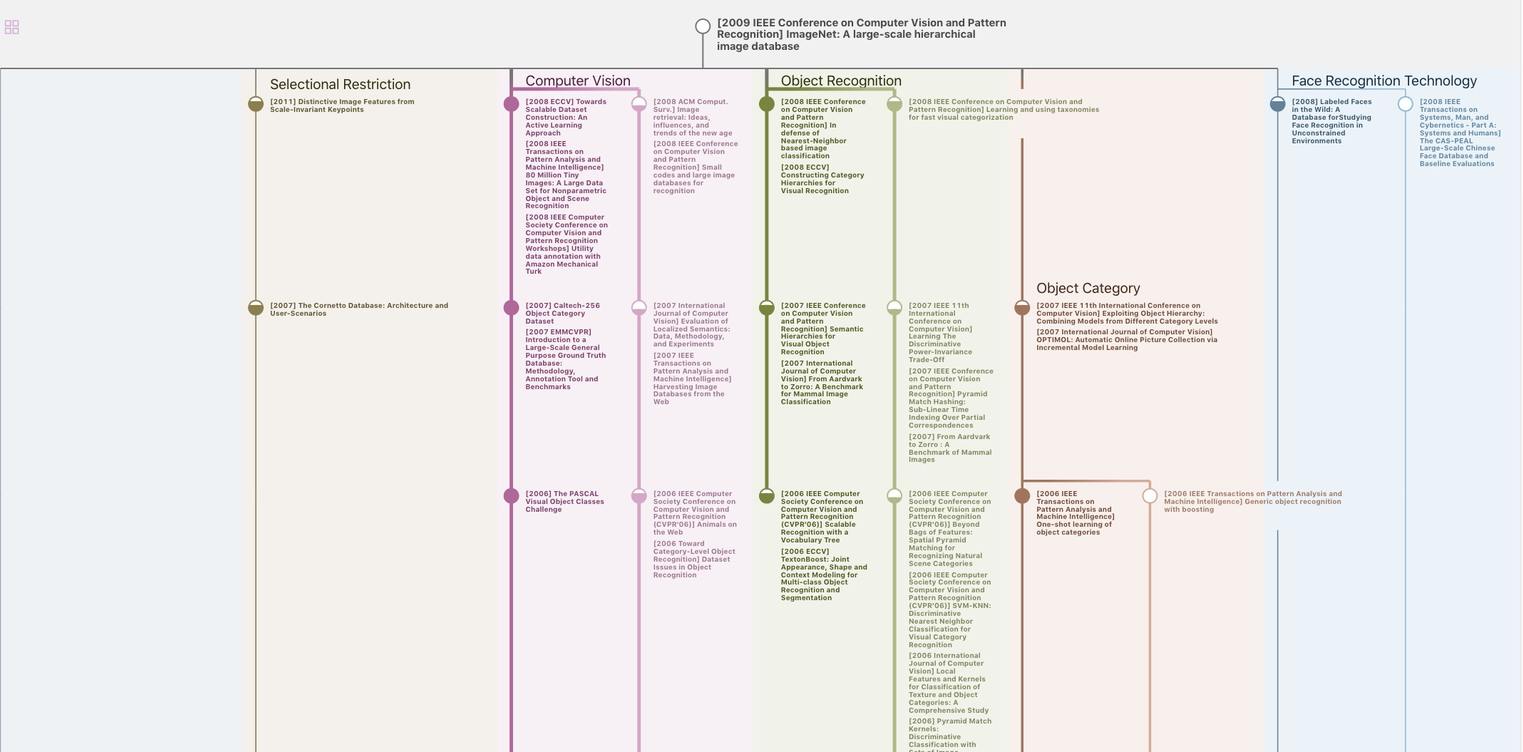
生成溯源树,研究论文发展脉络
Chat Paper
正在生成论文摘要