A new perspective on classification: Optimally allocating limited resources to uncertain tasks
DECISION SUPPORT SYSTEMS(2024)
摘要
A central problem in business concerns the optimal allocation of limited resources to a set of available tasks, where the payoff of these tasks is inherently uncertain. Typically, such problems are solved using a classification framework, where task outcomes are predicted given a set of characteristics. Then, resources are allocated to the tasks predicted to be the most likely to succeed. We argue, however, that using classification to address task uncertainty is inherently suboptimal as it does not take into account the available capacity. We present a novel solution that directly optimizes the assignment's expected profit given limited, stochastic capacity. This is achieved by optimizing a specific instance of the net discounted cumulative gain, a commonly used class of metrics in learning to rank. We demonstrate that our new method achieves higher expected profit and expected precision compared to a classification approach for a wide variety of application areas.
更多查看译文
关键词
Machine learning,Optimal resource allocation,Classification,Learning to rank
AI 理解论文
溯源树
样例
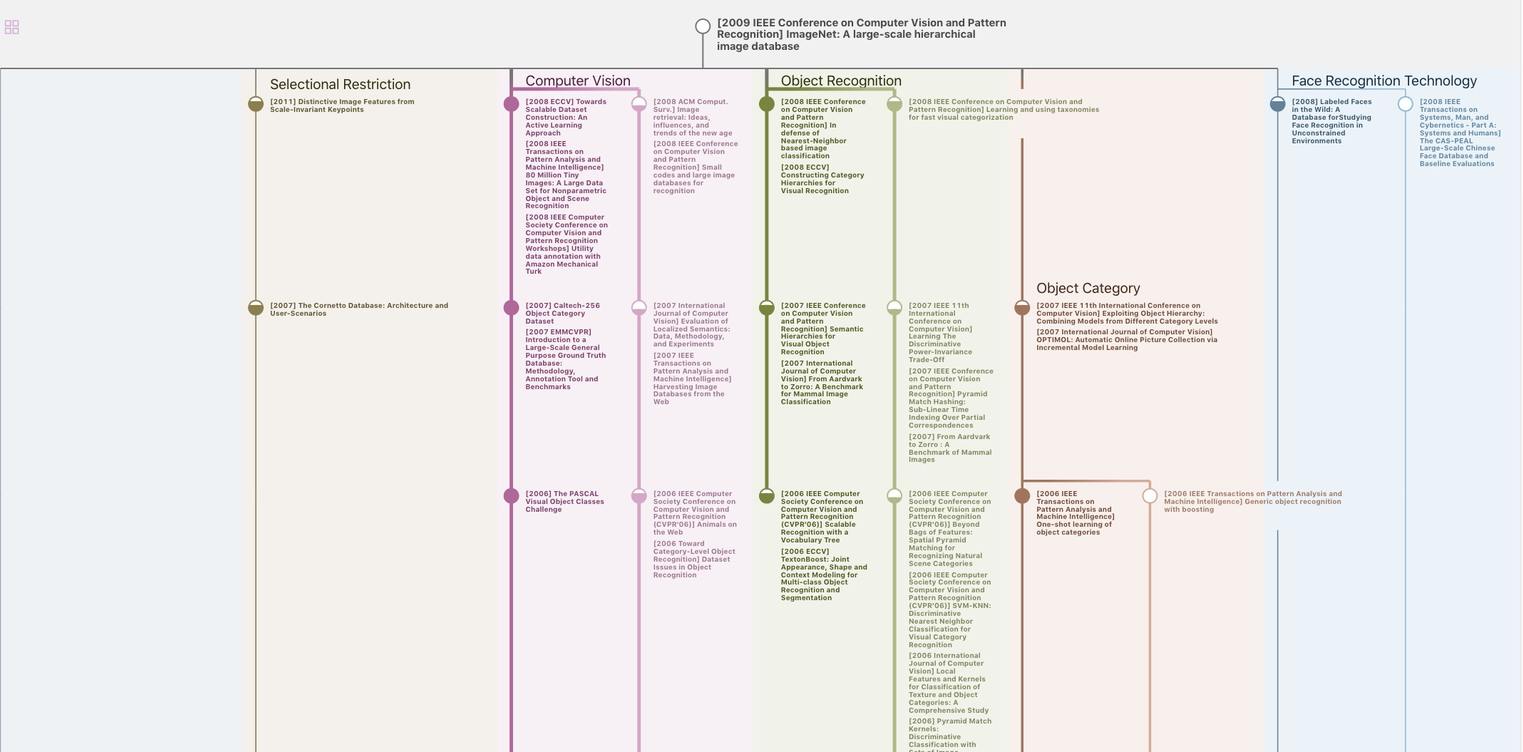
生成溯源树,研究论文发展脉络
Chat Paper
正在生成论文摘要