Facilitating MR-Guided Adaptive Proton Therapy in Children Using Deep Learning-Based Synthetic CT
INTERNATIONAL JOURNAL OF PARTICLE THERAPY(2022)
摘要
Purpose: To determine whether self-attention cycle-generative adversarial networks (cycle-GANs), a novel deep-learning method, can generate accurate synthetic computed tomography (sCT) to facilitate adaptive proton therapy in children with brain tumors. Materials and Methods: Both CT and T1-weighted magnetic resonance imaging (MRI) of 125 children (ages 1-20 years) with brain tumors were included in the training dataset. A model introducing a self-attention mechanism into the conventional cycle-GAN was created to enhance tissue interfaces and reduce noise. The test dataset consisted of 7 patients (ages 2-14 years) who underwent adaptive planning because of changes in anatomy discovered on MRI during proton therapy. The MRI during proton therapy-based sCT was compared with replanning CT (ground truth). Results: The Hounsfield unit-mean absolute error was significantly reduced with self-attention cycle-GAN, as compared with conventional cycle-GAN (65.3 +/- 13.9 versus 88.9 +/- 19.3, P < .01). The average 3-dimensional gamma passing rates (2%/2 mm criteria) for the original plan on the anatomy of the day and for the adapted plan were high (97.6% +/- 1.2% and 98.9 +/- 0.9%, respectively) when using sCT generated by self-attention cycle-GAN. The mean absolute differences in clinical target volume (CTV) receiving 95% of the prescription dose and 80% distal falloff along the beam axis were 1.1% +/- 0.8% and 1.1 +/- 0.9 mm, respectively. Areas of greatest dose difference were distal to the CTV and corresponded to shifts in distal falloff. Plan adaptation was appropriately triggered in all test patients when using sCT. Conclusion: The novel cycle-GAN model with self-attention outperforms conventional cycle-GAN for children with brain tumors. Encouraging dosimetric results suggest that sCT generation can be used to identify patients who would benefit from adaptive replanning.
更多查看译文
关键词
synthetic CT,adaptive proton therapy,cycle GAN,deep learning
AI 理解论文
溯源树
样例
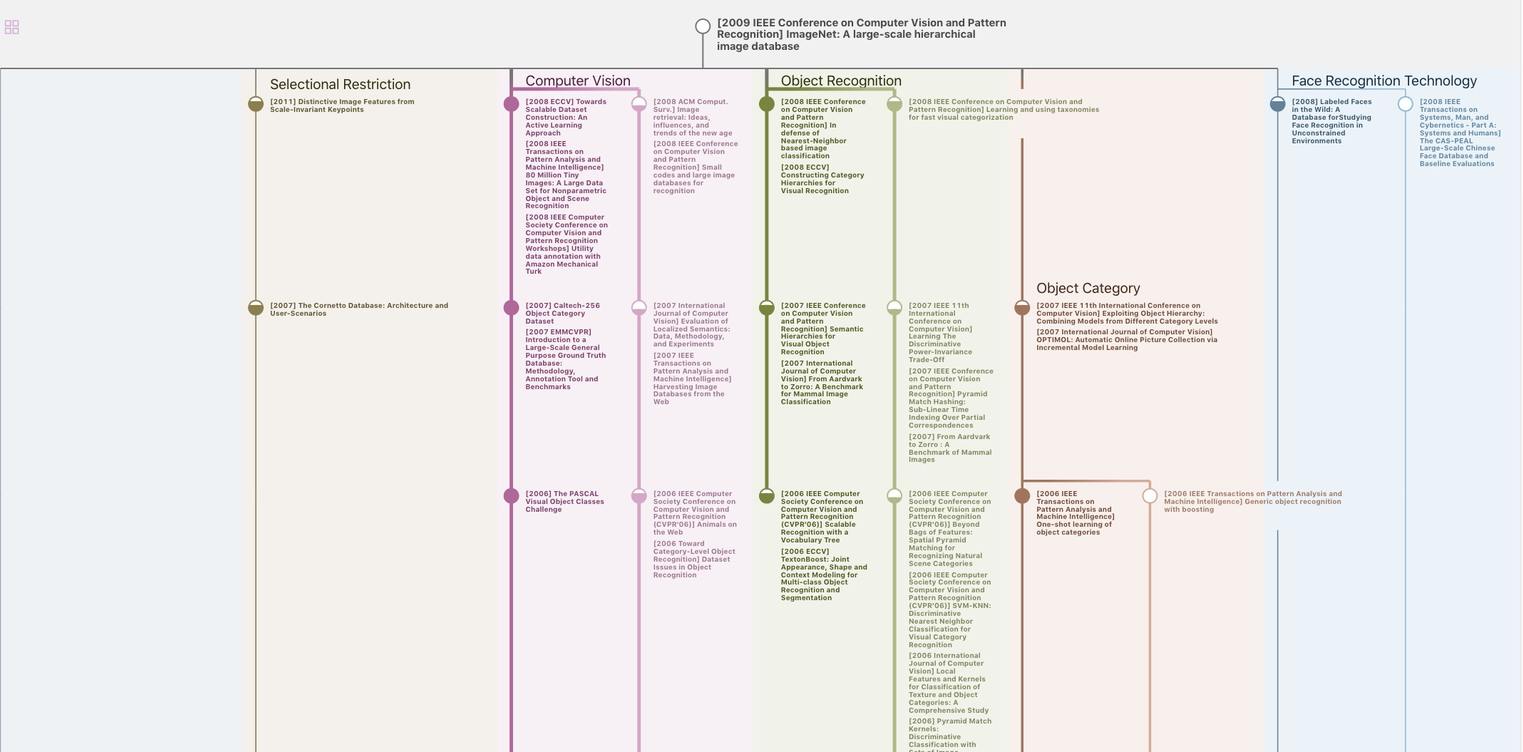
生成溯源树,研究论文发展脉络
Chat Paper
正在生成论文摘要