Deep Learning for Autism Diagnosis and Facial Analysis in Children
FRONTIERS IN COMPUTATIONAL NEUROSCIENCE(2022)
摘要
In this paper, we introduce a deep learning model to classify children as either healthy or potentially having autism with 94.6% accuracy using Deep Learning. Patients with autism struggle with social skills, repetitive behaviors, and communication, both verbal and non-verbal. Although the disease is considered to be genetic, the highest rates of accurate diagnosis occur when the child is tested on behavioral characteristics and facial features. Patients have a common pattern of distinct facial deformities, allowing researchers to analyze only an image of the child to determine if the child has the disease. While there are other techniques and models used for facial analysis and autism classification on their own, our proposal bridges these two ideas allowing classification in a cheaper, more efficient method. Our deep learning model uses MobileNet and two dense layers to perform feature extraction and image classification. The model is trained and tested using 3,014 images, evenly split between children with autism and children without it; 90% of the data is used for training and 10% is used for testing. Based on our accuracy, we propose that the diagnosis of autism can be done effectively using only a picture. Additionally, there may be other diseases that are similarly diagnosable.
更多查看译文
关键词
deep learning, autism, children, diagnosis, facial image analysis
AI 理解论文
溯源树
样例
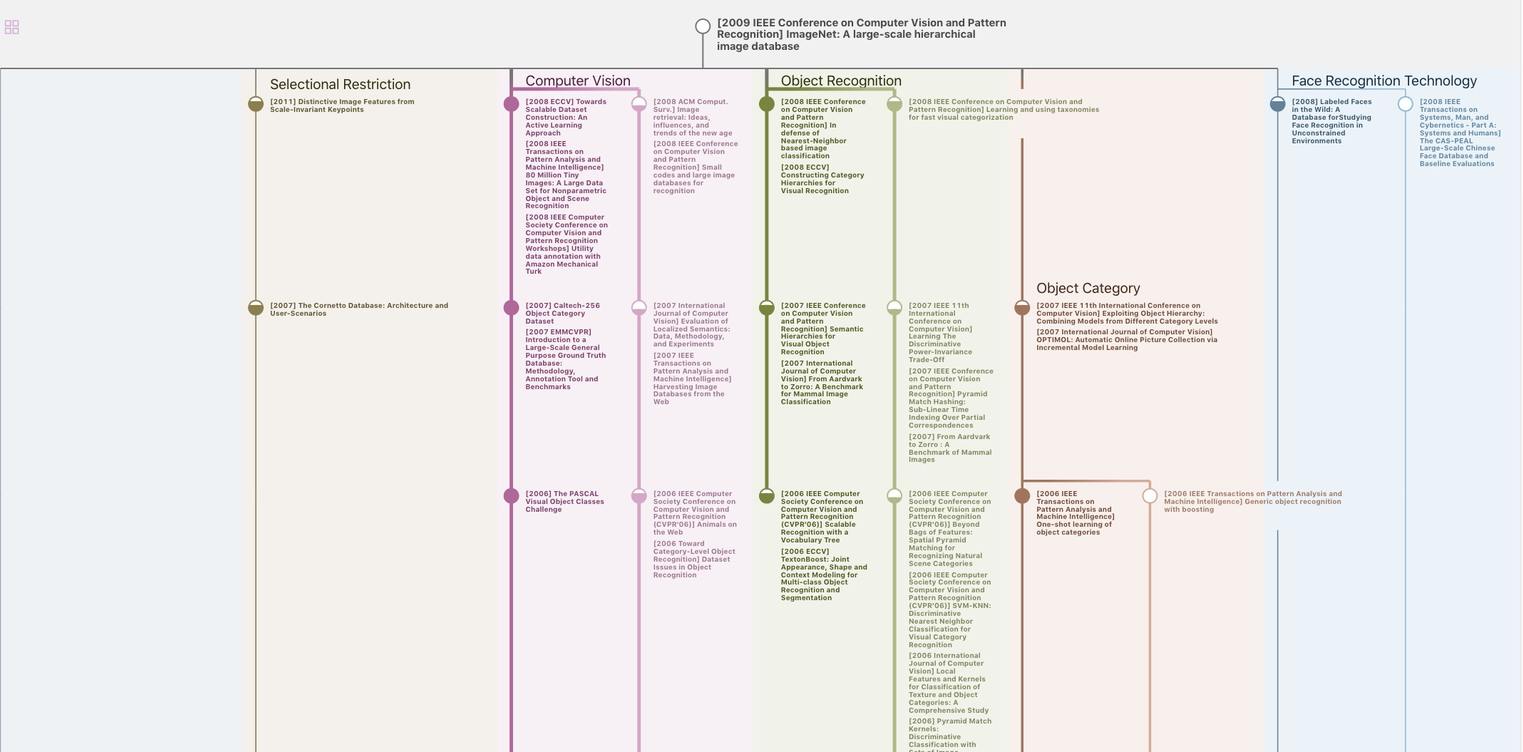
生成溯源树,研究论文发展脉络
Chat Paper
正在生成论文摘要