Unsupervised Bayesian classification for models with scalar and functional covariates
JOURNAL OF THE ROYAL STATISTICAL SOCIETY SERIES C-APPLIED STATISTICS(2024)
摘要
We consider unsupervised classification by means of a latent multinomial variable which categorizes a scalar response into one of the L components of a mixture model which incorporates scalar and functional covariates. This process can be thought as a hierarchical model with the first level modelling a scalar response according to a mixture of parametric distributions and the second level modelling the mixture probabilities by means of a generalized linear model with functional and scalar covariates. The traditional approach of treating functional covariates as vectors not only suffers from the curse of dimensionality, since functional covariates can be measured at very small intervals leading to a highly parametrized model, but also does not take into account the nature of the data. We use basis expansions to reduce the dimensionality and a Bayesian approach for estimating the parameters while providing predictions of the latent classification vector. The method is motivated by two data examples that are not easily handled by existing methods. The first example concerns identifying placebo responders on a clinical trial (normal mixture model) and the other predicting illness for milking cows (zero-inflated mixture of the Poisson model).
更多查看译文
关键词
functional covariates,latent vector,unsupervised clustering,variable selection,variational inference
AI 理解论文
溯源树
样例
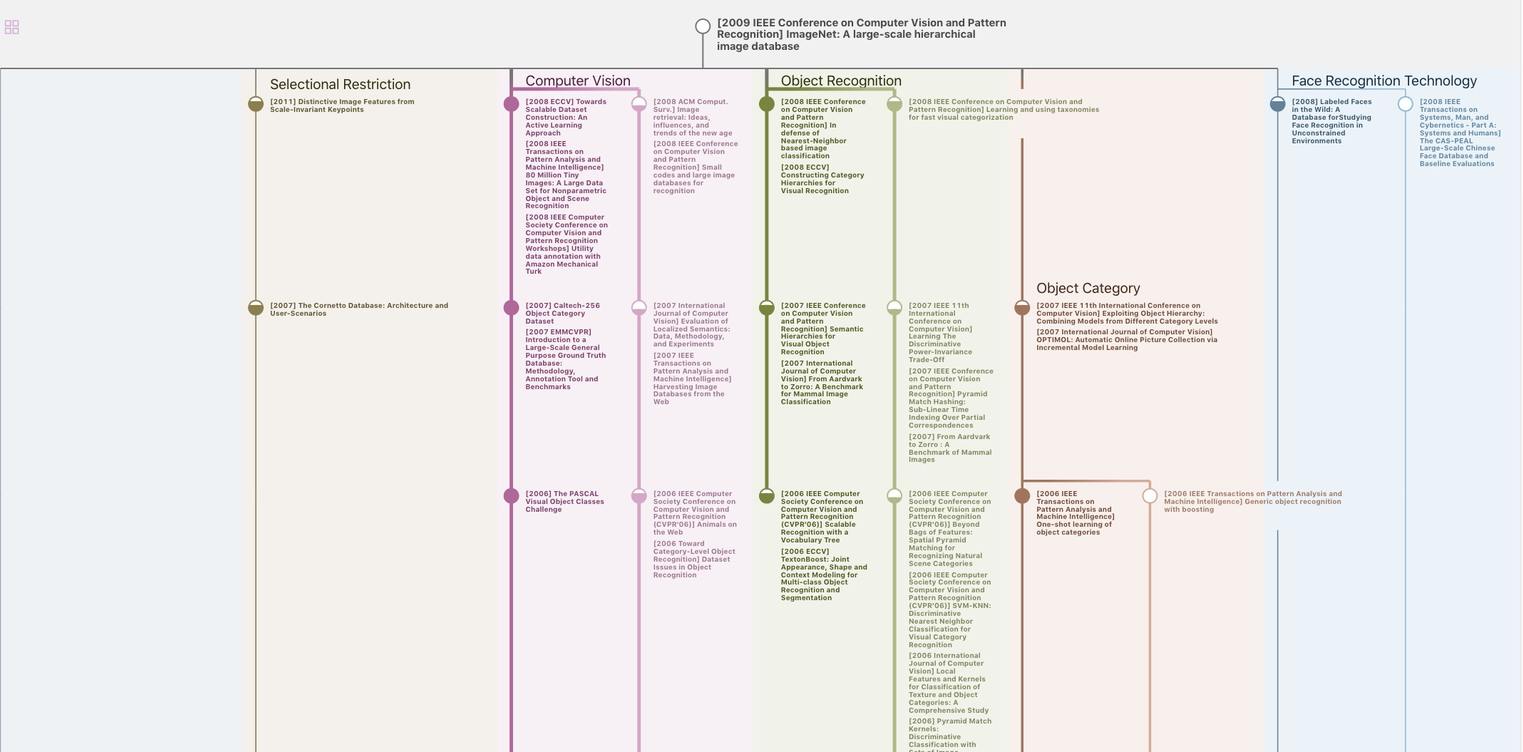
生成溯源树,研究论文发展脉络
Chat Paper
正在生成论文摘要