DURableVS: Data-efficient Unsupervised Recalibrating Visual Servoing via online learning in a structured generative model
IEEE International Conference on Robotics and Automation(2022)
摘要
Visual servoing enables robotic systems to perform accurate closed-loop control, which is required in many applications. However, existing methods require either precise calibration of the robot kinematic model and cameras or use neural architectures that require large amounts of data to train. In this work, we present a method for unsupervised learning of visual servoing that does not require any prior calibration and is extremely data-efficient. Our key insight is that visual servoing does not depend on identifying the veridical kinematic and camera parameters, but instead only on an accurate generative model of image feature observations from the joint positions of the robot. We demonstrate that with our model architecture and learning algorithm, we can consistently learn accurate models from less than 50 training samples (which amounts to less than 1 min of unsupervised data collection), and that such data-efficient learning is not possible with standard neural architectures. Further, we show that by using the generative model in the loop and learning online, we can enable a robotic system to recover from calibration errors and to detect and quickly adapt to possibly unexpected changes in the robot-camera system (e.g. bumped camera, new objects).
更多查看译文
关键词
standard neural architectures,robot-camera system,data-efficient unsupervised recalibrating visual servoing,online learning,structured generative model,closed-loop control,robot kinematic model,unsupervised learning,veridical kinematic camera,model architecture,unsupervised data collection,data-efficient learning,DURableVS,image feature observation
AI 理解论文
溯源树
样例
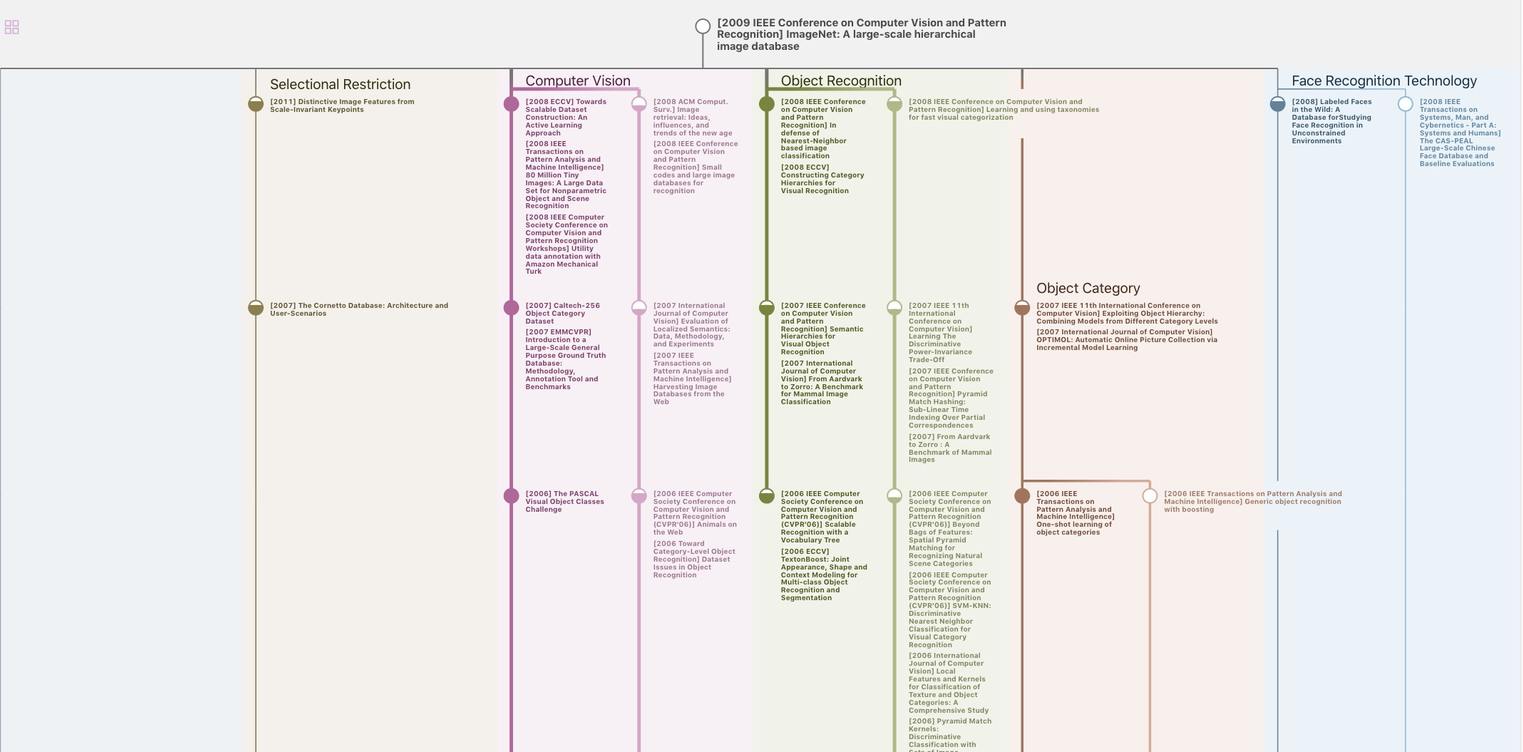
生成溯源树,研究论文发展脉络
Chat Paper
正在生成论文摘要