Self-Supervised Representation Learning for Speech Using Visual Grounding and Masked Language Modeling
arxiv(2022)
摘要
In this paper, we describe our submissions to the ZeroSpeech 2021 Challenge and SUPERB benchmark. Our submissions are based on the recently proposed FaST-VGS model, which is a Transformer-based model that learns to associate raw speech waveforms with semantically related images, all without the use of any transcriptions of the speech. Additionally, we introduce a novel extension of this model, FaST-VGS+, which is learned in a multi-task fashion with a masked language modeling objective in addition to the visual grounding objective. On ZeroSpeech 2021, we show that our models perform competitively on the ABX task, outperform all other concurrent submissions on the Syntactic and Semantic tasks, and nearly match the best system on the Lexical task. On the SUPERB benchmark, we show that our models also achieve strong performance, in some cases even outperforming the popular wav2vec2.0 model.
更多查看译文
关键词
speech,learning,representation,grounding,self-supervised
AI 理解论文
溯源树
样例
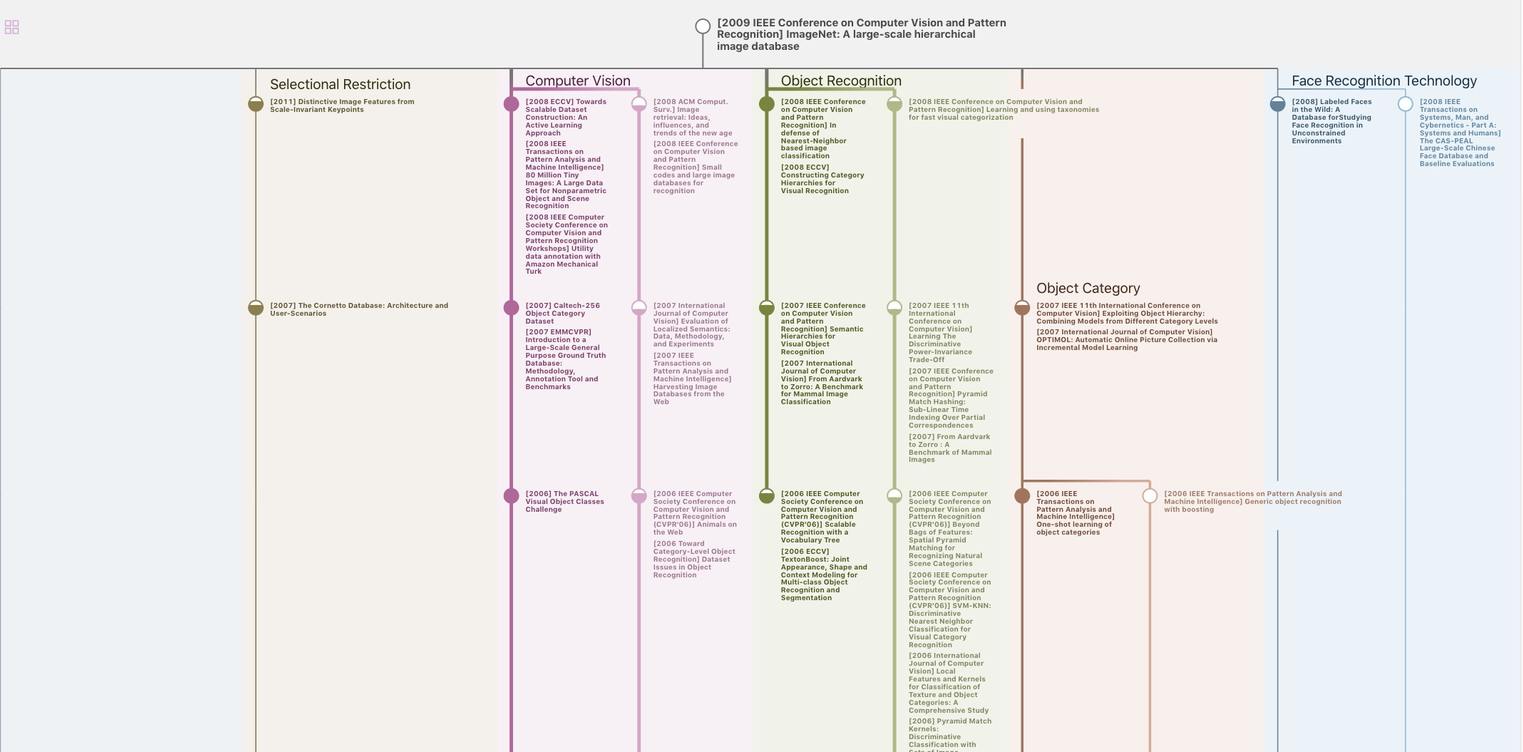
生成溯源树,研究论文发展脉络
Chat Paper
正在生成论文摘要