Maximizing Audio Event Detection Model Performance on Small Datasets Through Knowledge Transfer, Data Augmentation, and Pretraining: an Ablation Study.
IEEE International Conference on Acoustics, Speech, and Signal Processing (ICASSP)(2022)
摘要
An Xception model reaches state-of-the-art (SOTA) accuracy on the ESC-50 dataset for audio event detection through knowledge transfer from ImageNet weights, pretraining on AudioSet, and an on-the-fly data augmentation pipeline. This paper presents an ablation study that analyzes which components contribute to the boost in performance and training time. A smaller Xception model is also presented which nears SOTA performance with almost a third of the parameters.
更多查看译文
关键词
Audio Event Detection,Data Augmentation,Knowledge Transfer,Ablation Study
AI 理解论文
溯源树
样例
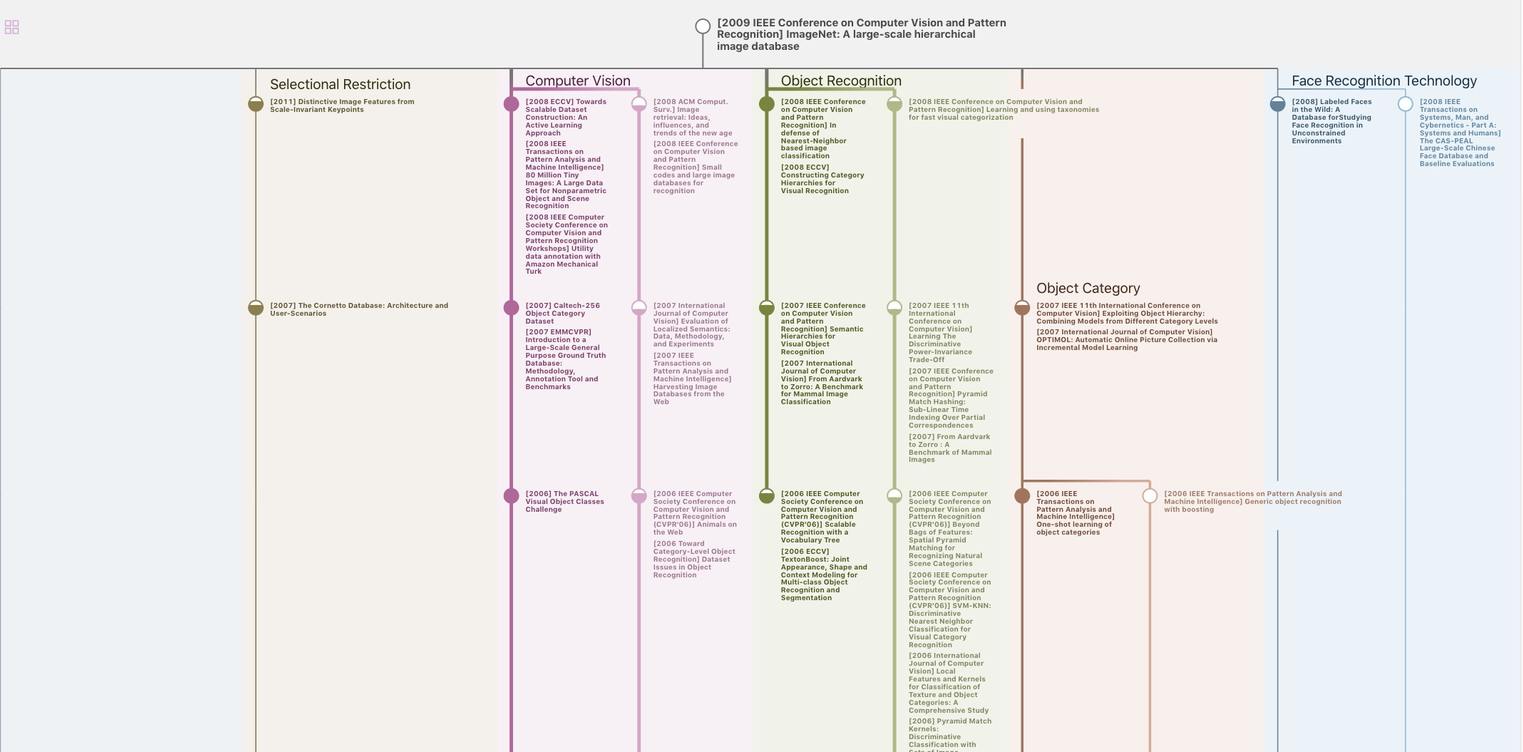
生成溯源树,研究论文发展脉络
Chat Paper
正在生成论文摘要