PatClArC: Using Pattern Concept Activation Vectors for Noise-Robust Model Debugging
arxiv(2022)
摘要
State-of-the-art machine learning models are commonly (pre-)trained on large benchmark datasets. These often contain biases, artifacts, or errors that have remained unnoticed in the data collection process and therefore fail in representing the real world truthfully. This can cause models trained on these datasets to learn undesired behavior based upon spurious correlations, e.g., the existence of a copyright tag in an image. Concept Activation Vectors (CAV) have been proposed as a tool to model known concepts in latent space and have been used for concept sensitivity testing and model correction. Specifically, class artifact compensation (ClArC) corrects models using CAVs to represent data artifacts in feature space linearly. Modeling CAVs with filters of linear models, however, causes a significant influence of the noise portion within the data, as recent work proposes the unsuitability of linear model filters to find the signal direction in the input, which can be avoided by instead using patterns. In this paper we propose Pattern Concept Activation Vectors (PCAV) for noise-robust concept representations in latent space. We demonstrate that pattern-based artifact modeling has beneficial effects on the application of CAVs as a means to remove influence of confounding features from models via the ClArC framework.
更多查看译文
关键词
model debugging,pattern concept activation vectors,noise-robust
AI 理解论文
溯源树
样例
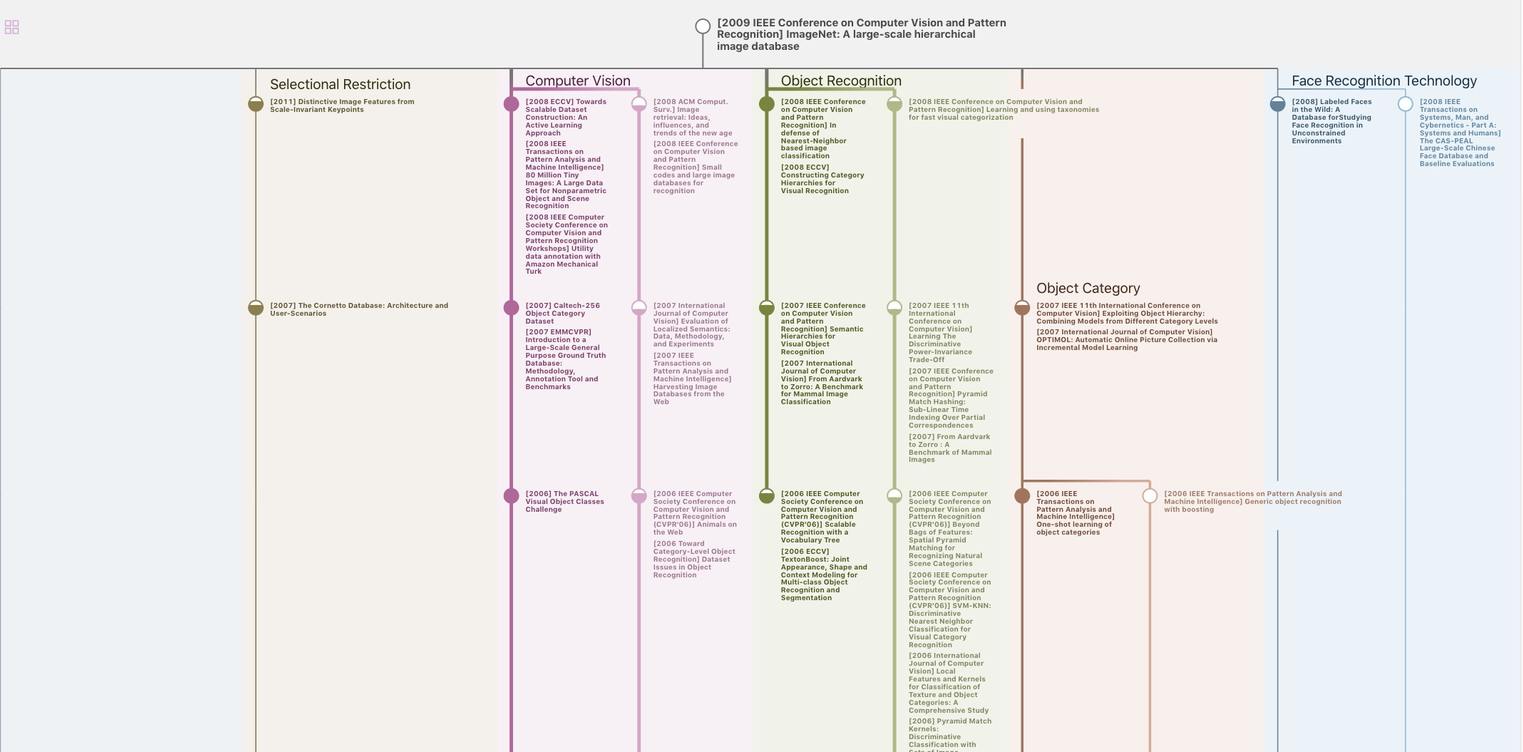
生成溯源树,研究论文发展脉络
Chat Paper
正在生成论文摘要