Auto-Lambda: Disentangling Dynamic Task Relationships
arxiv(2022)
摘要
Understanding the structure of multiple related tasks allows for multi-task learning to improve the generalisation ability of one or all of them. However, it usually requires training each pairwise combination of tasks together in order to capture task relationships, at an extremely high computational cost. In this work, we learn task relationships via an automated weighting framework, named Auto-Lambda. Unlike previous methods where task relationships are assumed to be fixed, Auto-Lambda is a gradient-based meta learning framework which explores continuous, dynamic task relationships via task-specific weightings, and can optimise any choice of combination of tasks through the formulation of a meta-loss; where the validation loss automatically influences task weightings throughout training. We apply the proposed framework to both multi-task and auxiliary learning problems in computer vision and robotics, and show that Auto-Lambda achieves state-of-the-art performance, even when compared to optimisation strategies designed specifically for each problem and data domain. Finally, we observe that Auto-Lambda can discover interesting learning behaviors, leading to new insights in multi-task learning. Code is available at https://github.com/lorenmt/auto-lambda.
更多查看译文
关键词
task,dynamic,auto-lambda
AI 理解论文
溯源树
样例
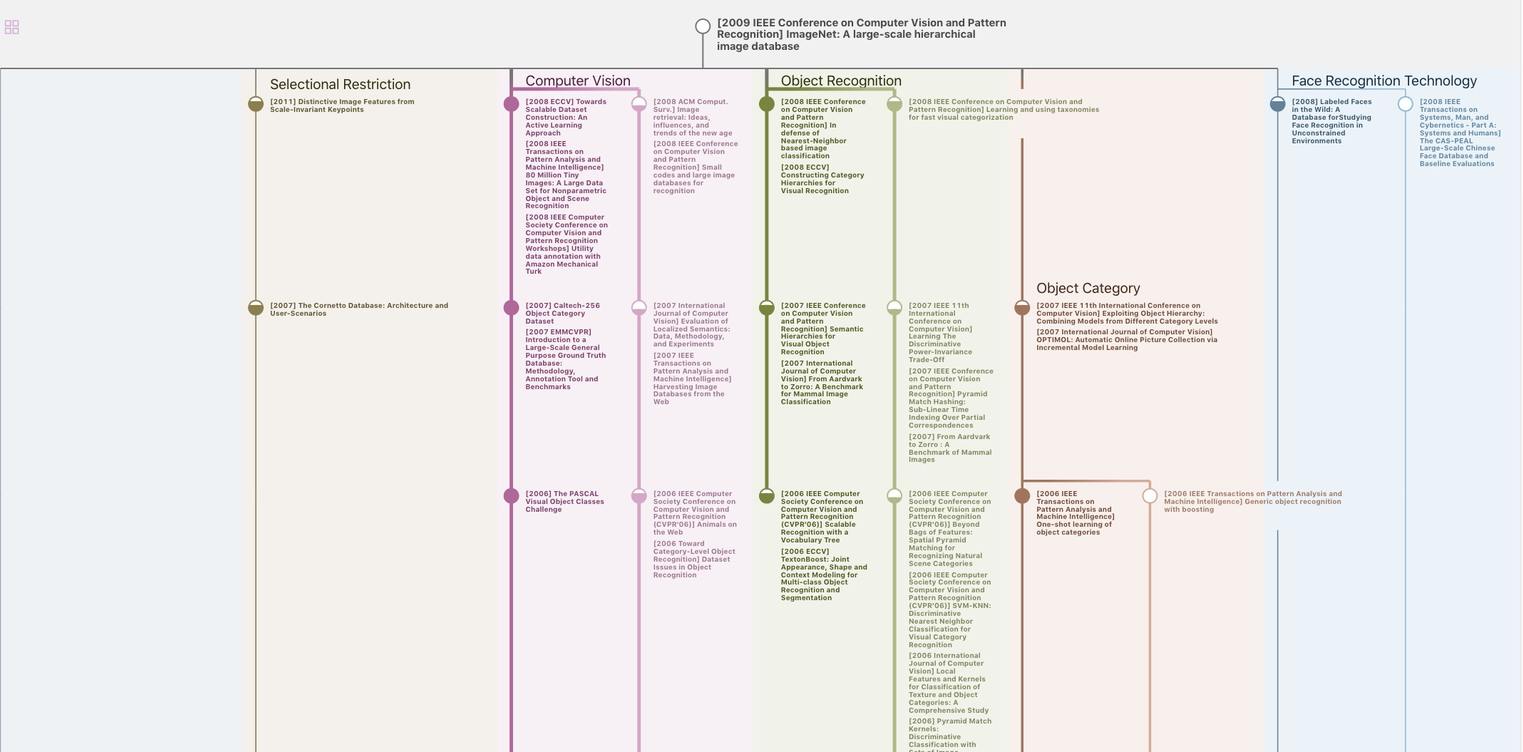
生成溯源树,研究论文发展脉络
Chat Paper
正在生成论文摘要