A Compressed Sensing Based Least Squares Approach to Semi-supervised Local Cluster Extraction
arxiv(2023)
摘要
A least squares semi-supervised local clustering algorithm based on the idea of compressed sensing is proposed to extract clusters from a graph with known adjacency matrix. The algorithm is based on a two-stage approach similar to the one proposed by Lai and Mckenzie (SIAM J Math Data Sci 2:368–395, 2020). However, under a weaker assumption and with less computational complexity, our algorithms are shown to be able to find a desired cluster with high probability. The “one cluster at a time" feature of our method distinguishes it from other global clustering methods. Numerical experiments are conducted on the synthetic data such as stochastic block model and real data such as MNIST, political blogs network, AT &T and YaleB human faces data sets to demonstrate the effectiveness and efficiency of our algorithms.
更多查看译文
关键词
Graph clustering, Semi-supervised clustering, Local clustering, Compressed sensing, Least squares, Graph Laplacian, 68Q25, 68R10, 68U05, 94A12
AI 理解论文
溯源树
样例
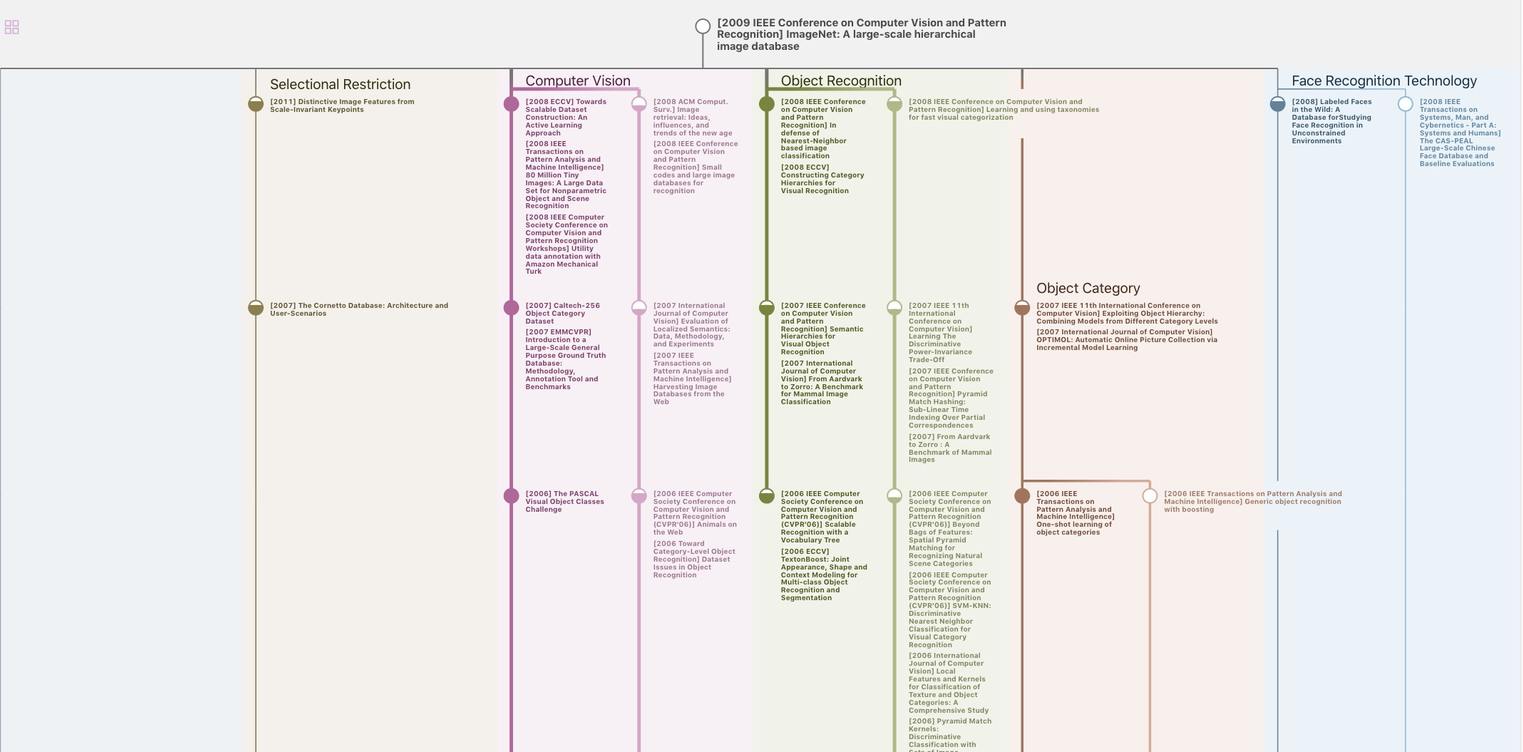
生成溯源树,研究论文发展脉络
Chat Paper
正在生成论文摘要