Missing Mass Estimation from Sticky Channels.
International Symposium on Information Theory (ISIT)(2022)
摘要
Distribution estimation under error-prone or non-ideal sampling modelled as "sticky" channels have been studied recently motivated by applications such as DNA computing. Missing mass, the sum of probabilities of missing letters, is an important quantity that plays a crucial role in distribution estimation, particularly in the large alphabet regime. In this work, we consider the problem of estimation of missing mass, which has been well-studied under independent and identically distributed (i.i.d) sampling, in the case when sampling is "sticky". Precisely, we consider the scenario where each sample from an unknown distribution gets repeated a geometrically-distributed number of times. We characterise the minimax rate of Mean Squared Error (MSE) of estimating missing mass from such sticky sampling channels. An upper bound on the minimax rate is obtained by bounding the risk of a modified Good-Turing estimator. We derive a matching lower bound on the minimax rate by extending the Le Cam method.
更多查看译文
关键词
estimation,mass
AI 理解论文
溯源树
样例
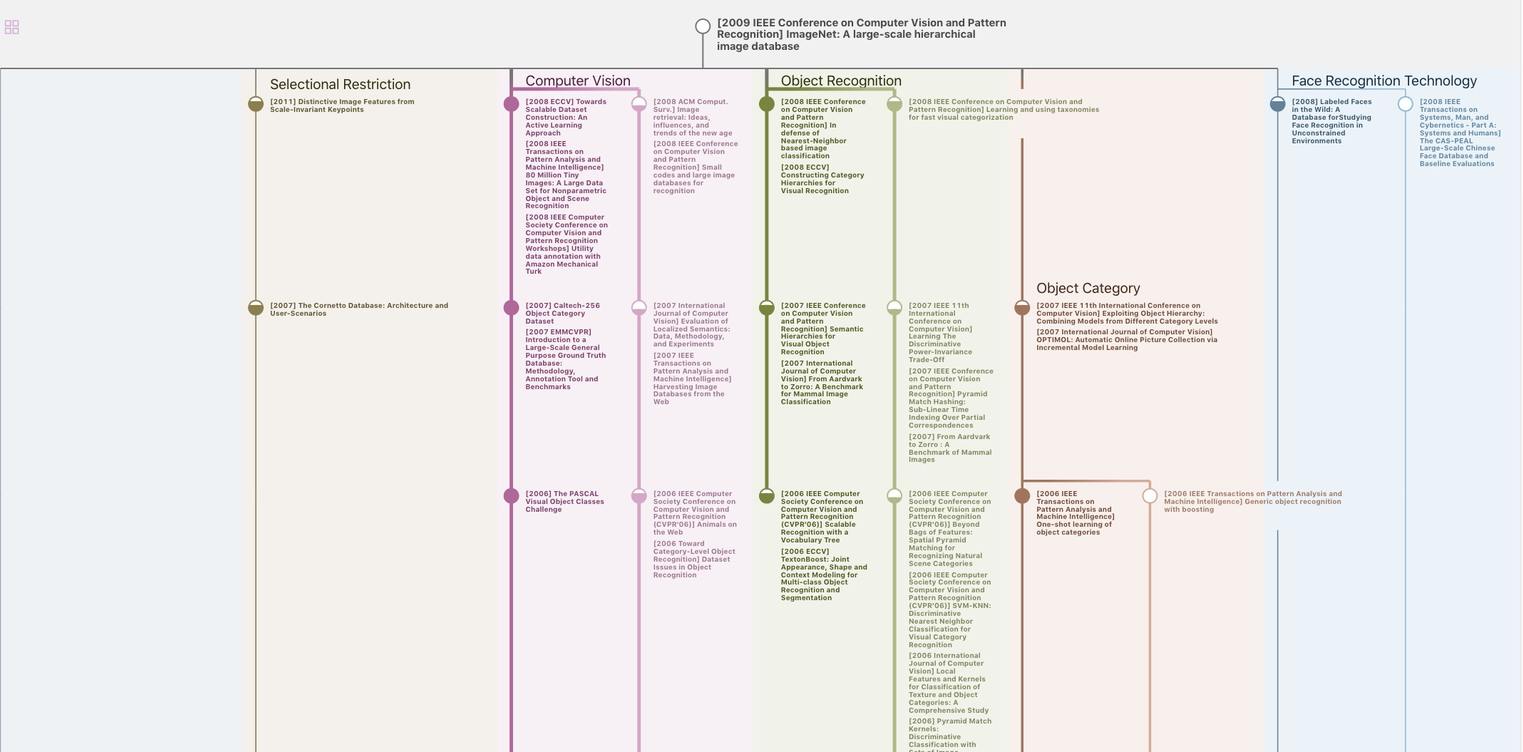
生成溯源树,研究论文发展脉络
Chat Paper
正在生成论文摘要