BAM: Bayes with Adaptive Memory
International Conference on Learning Representations (ICLR)(2022)
摘要
Online learning via Bayes' theorem allows new data to be continuously integrated into an agent's current beliefs. However, a naive application of Bayesian methods in non-stationary environments leads to slow adaptation and results in state estimates that may converge confidently to the wrong parameter value. A common solution when learning in changing environments is to discard/downweight past data; however, this simple mechanism of "forgetting" fails to account for the fact that many real-world environments involve revisiting similar states. We propose a new framework, Bayes with Adaptive Memory (BAM), that takes advantage of past experience by allowing the agent to choose which past observations to remember and which to forget. We demonstrate that BAM generalizes many popular Bayesian update rules for non-stationary environments. Through a variety of experiments, we demonstrate the ability of BAM to continuously adapt in an ever-changing world.
更多查看译文
关键词
Bayesian learning,online learning
AI 理解论文
溯源树
样例
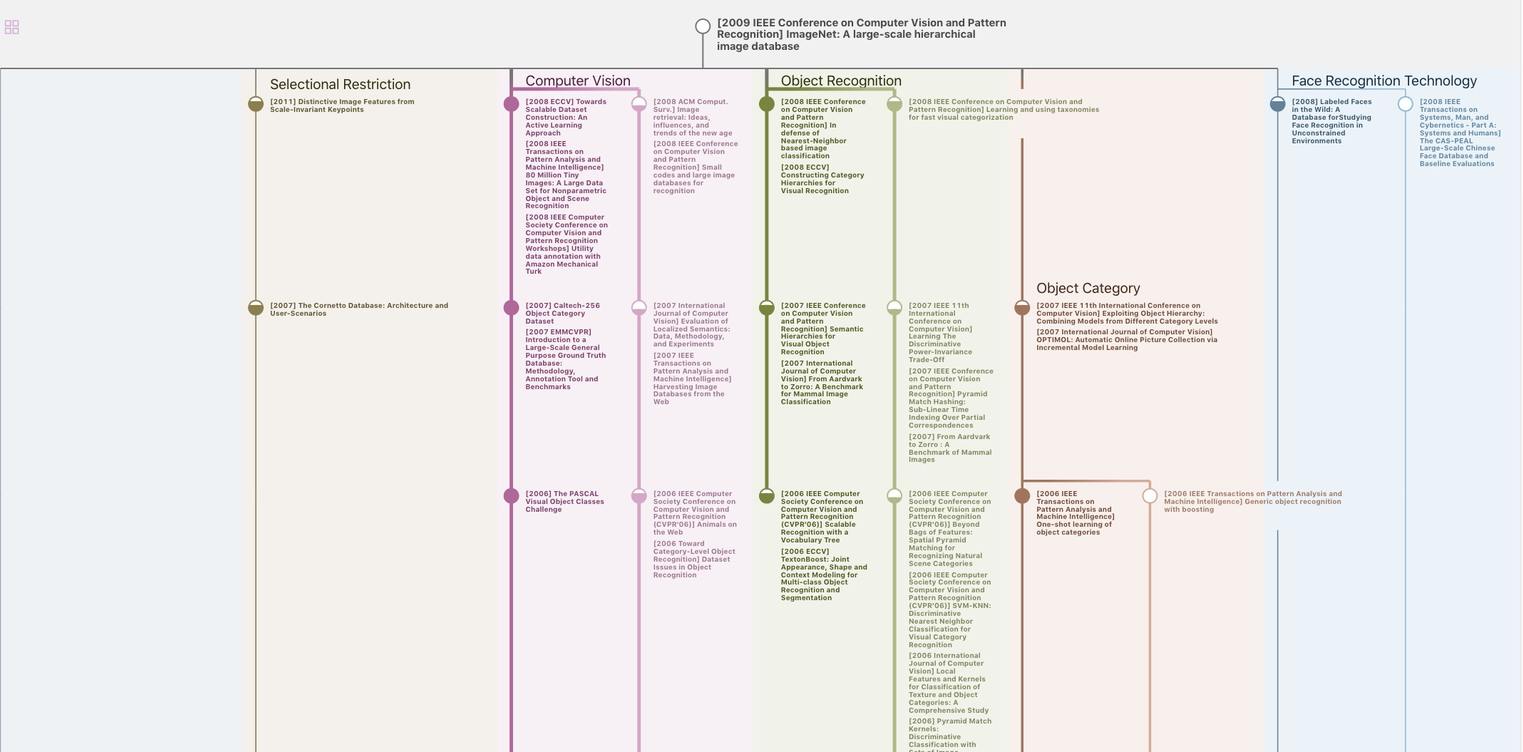
生成溯源树,研究论文发展脉络
Chat Paper
正在生成论文摘要