Poster Abstract: Model-Free Reinforcement Learning for Symbolic Automata-encoded Objectives
arxiv(2022)
摘要
ABSTRACTIn this work, we propose the use of symbolic automata as formal specifications for reinforcement learning agents. The use of symbolic automata serves as a generalization of both bounded-time temporal logic-based specifications and deterministic finite automata, allowing us to describe input alphabets over metric spaces. Furthermore, our use of symbolic automata allows us to define non-sparse potential-based rewards which empirically shape the reward surface, leading to better convergence during RL. We also show that our potential-based rewarding strategy still allows us to obtain the policy that maximizes the satisfaction of the given specification.
更多查看译文
AI 理解论文
溯源树
样例
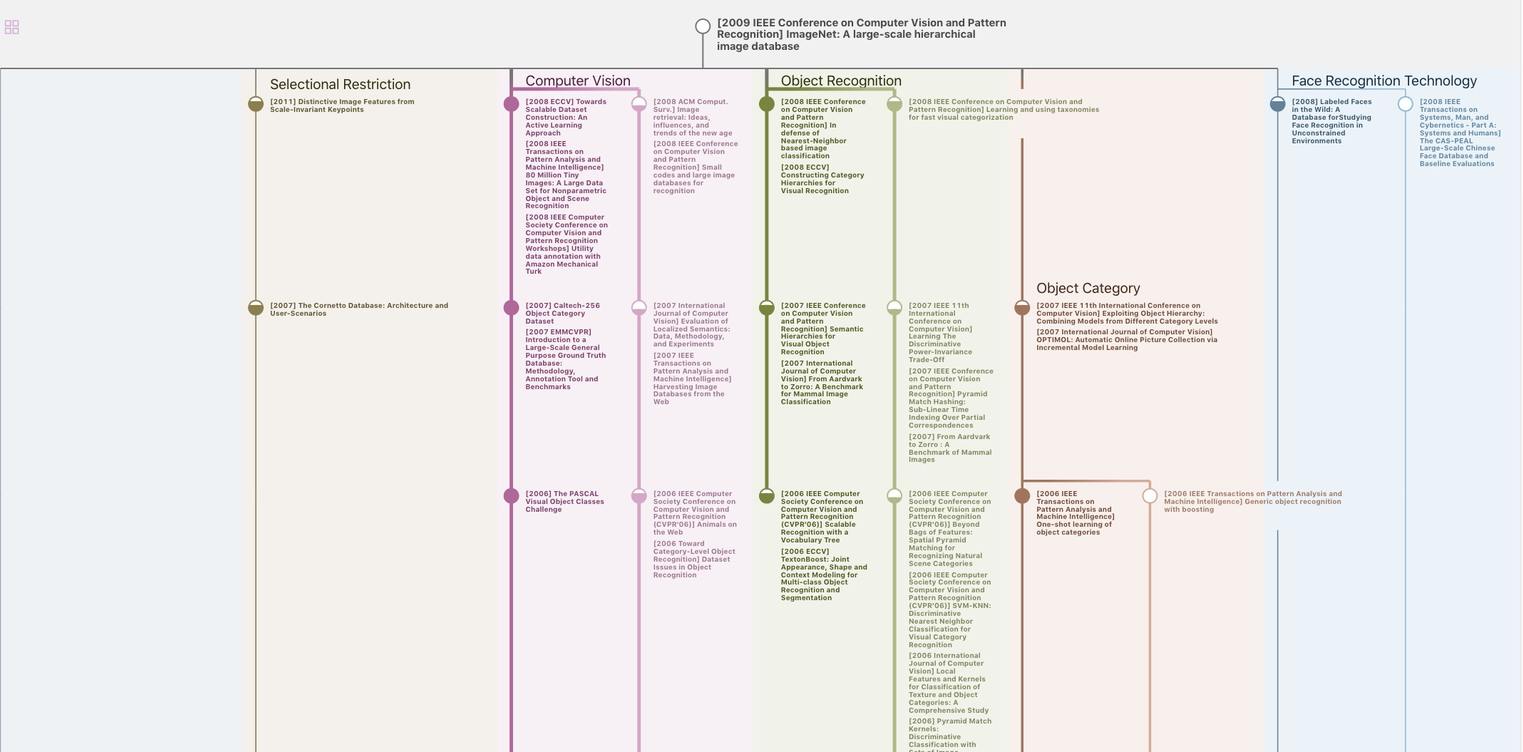
生成溯源树,研究论文发展脉络
Chat Paper
正在生成论文摘要