Decoupling Local and Global Representations of Time Series
INTERNATIONAL CONFERENCE ON ARTIFICIAL INTELLIGENCE AND STATISTICS, VOL 151(2022)
摘要
Real-world time series data are often generated from several sources of variation. Learning representations that capture the factors contributing to this variability enables a better understanding of the data via its underlying generative process and improves performance on downstream machine learning tasks. This paper proposes a novel generative approach for learning representations for the global and local factors of variation in time series. The local representation of each sample models non-stationarity over time with a stochastic process prior, and the global representation of the sample encodes the time-independent characteristics. To encourage decoupling between the representations, we introduce counterfactual regularization that minimizes the mutual information between the two variables. In experiments, we demonstrate successful recovery of the true local and global variability factors on simulated data, and show that representations learned using our method yield superior performance on downstream tasks on real-world datasets. We believe that the proposed way of defining representations is beneficial for data modelling and yields better insights into the complexity of real-world data.(1)
更多查看译文
关键词
global representations,series,local
AI 理解论文
溯源树
样例
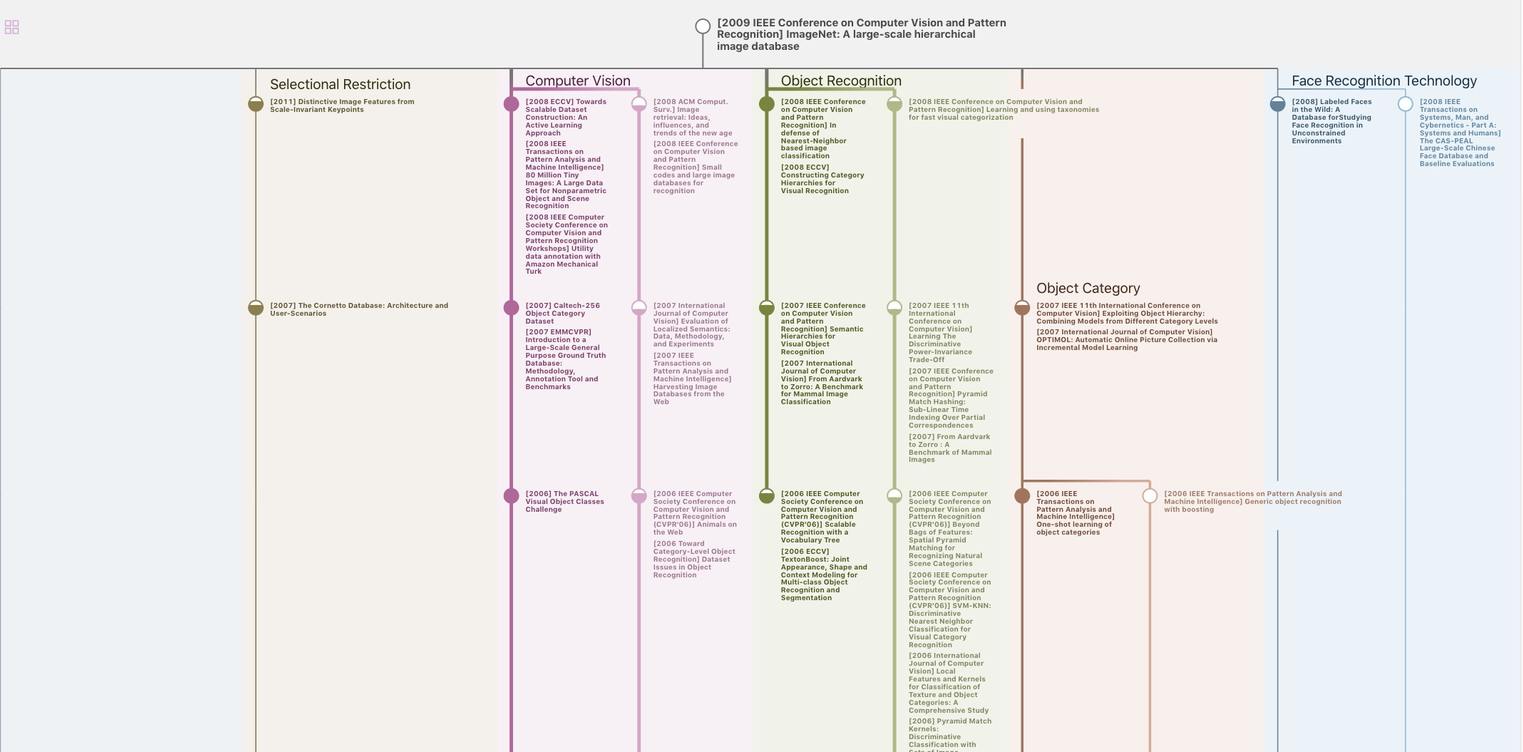
生成溯源树,研究论文发展脉络
Chat Paper
正在生成论文摘要