Source data selection for out-of-domain generalization
arxiv(2022)
摘要
Models that perform out-of-domain generalization borrow knowledge from heterogeneous source data and apply it to a related but distinct target task. Transfer learning has proven effective for accomplishing this generalization in many applications. However, poor selection of a source dataset can lead to poor performance on the target, a phenomenon called negative transfer. In order to take full advantage of available source data, this work studies source data selection with respect to a target task. We propose two source selection methods that are based on the multi-bandit theory and random search, respectively. We conduct a thorough empirical evaluation on both simulated and real data. Our proposals can be also viewed as diagnostics for the existence of a reweighted source subsamples that perform better than the random selection of available samples.
更多查看译文
关键词
source data selection,out-of-domain
AI 理解论文
溯源树
样例
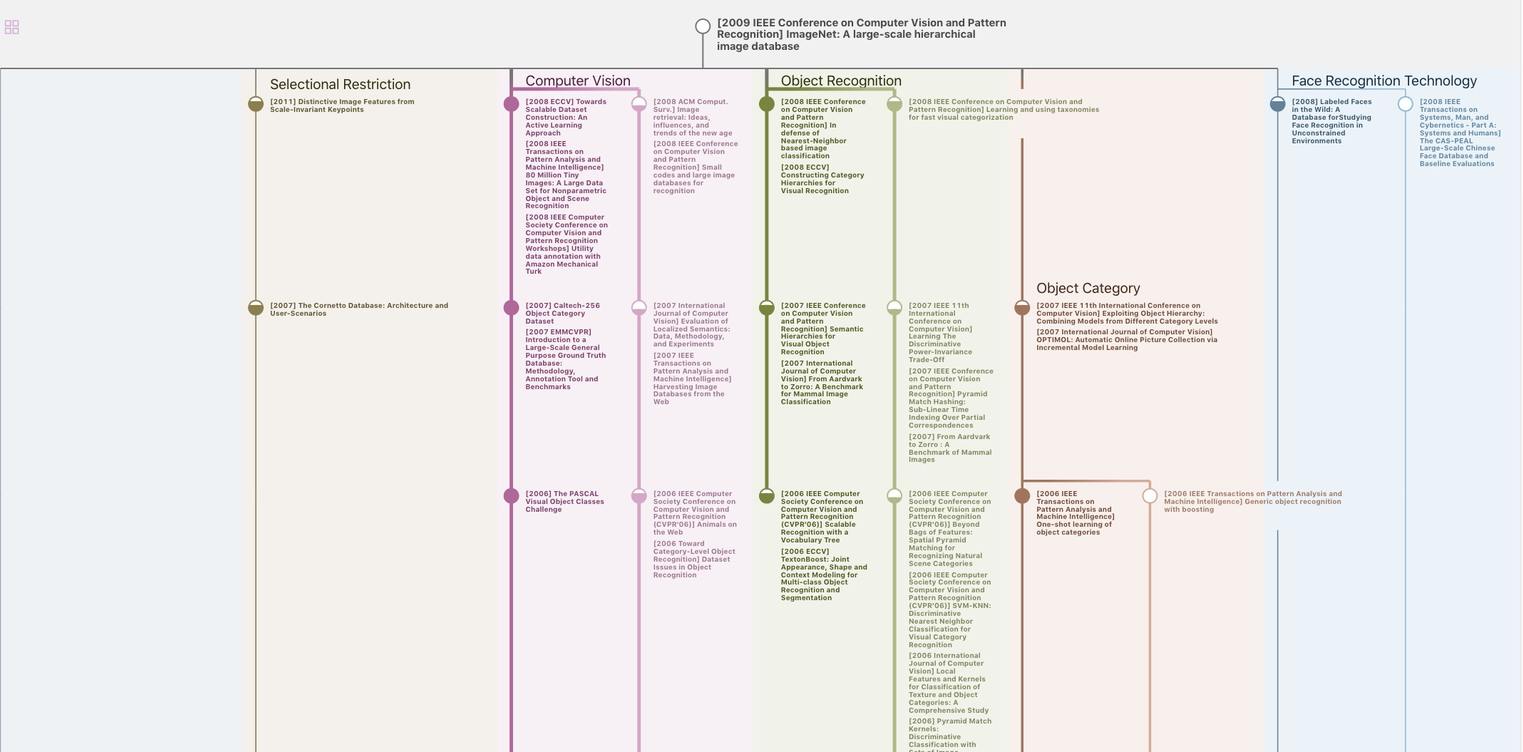
生成溯源树,研究论文发展脉络
Chat Paper
正在生成论文摘要