A Note on "Assessing Generalization of SGD via Disagreement"
arxiv(2022)
摘要
Several recent works find empirically that the average test error of deep neural networks can be estimated via the prediction disagreement of models, which does not require labels. In particular, Jiang et al. (2022) show for the disagreement between two separately trained networks that this `Generalization Disagreement Equality' follows from the well-calibrated nature of deep ensembles under the notion of a proposed `class-aggregated calibration.' In this reproduction, we show that the suggested theory might be impractical because a deep ensemble's calibration can deteriorate as prediction disagreement increases, which is precisely when the coupling of test error and disagreement is of interest, while labels are needed to estimate the calibration on new datasets. Further, we simplify the theoretical statements and proofs, showing them to be straightforward within a probabilistic context, unlike the original hypothesis space view employed by Jiang et al. (2022).
更多查看译文
关键词
sgd,generalization,disagreement
AI 理解论文
溯源树
样例
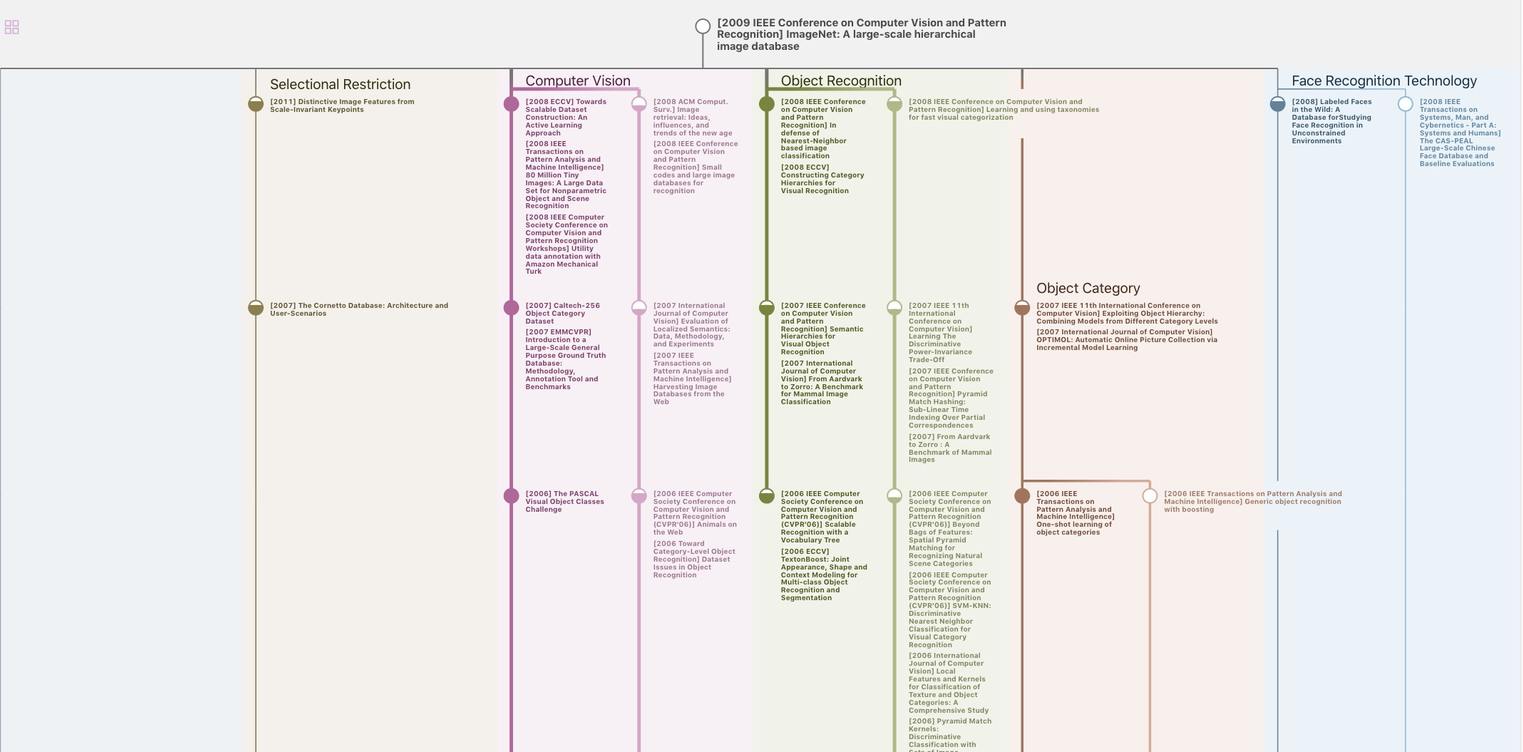
生成溯源树,研究论文发展脉络
Chat Paper
正在生成论文摘要