Stability Analysis for Delayed Neural Networks via a Generalized Reciprocally Convex Inequality
IEEE transactions on neural networks and learning systems(2023)
摘要
This article deals with the stability of neural networks (NNs) with time-varying delay. First, a generalized reciprocally convex inequality (RCI) is presented, providing a tight bound for reciprocally convex combinations. This inequality includes some existing ones as special case. Second, in order to cater for the use of the generalized RCI, a novel Lyapunov–Krasovskii functional (LKF) is constructed, which includes a generalized delay-product term. Third, based on the generalized RCI and the novel LKF, several stability criteria for the delayed NNs under study are put forward. Finally, two numerical examples are given to illustrate the effectiveness and advantages of the proposed stability criteria.
更多查看译文
关键词
Artificial neural networks,Stability criteria,Delays,Symmetric matrices,Neurons,Time-varying systems,Linear matrix inequalities,Delayed neural network (NN),Lyapunov-Krasovskii functional (LKF),reciprocally convex combination,stability criteria
AI 理解论文
溯源树
样例
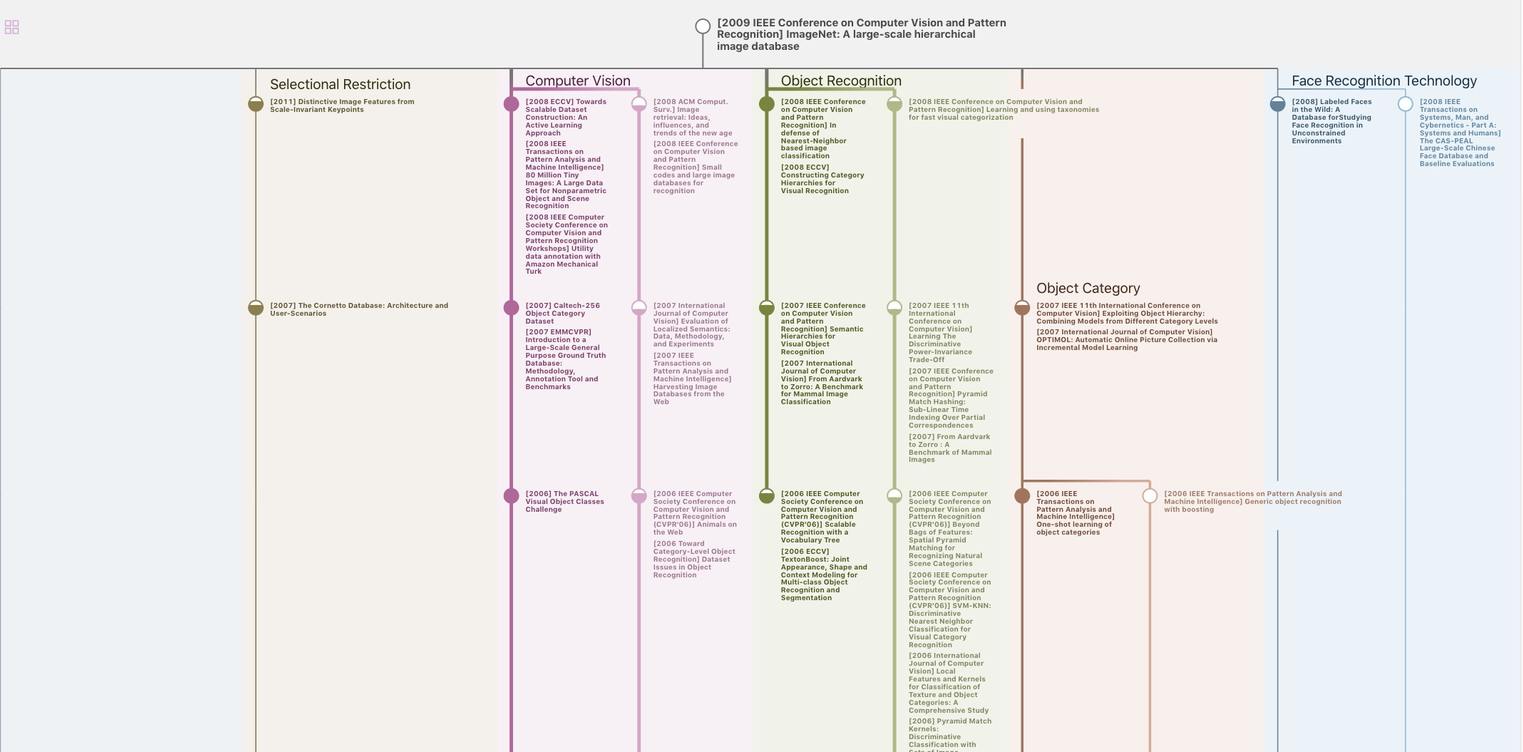
生成溯源树,研究论文发展脉络
Chat Paper
正在生成论文摘要