ExPoSe: Combining State-Based Exploration with Gradient-Based Online Search
arxiv(2023)
摘要
Online tree-based search algorithms iteratively simulate trajectories and update action-values for a set of states stored in a tree structure. It works reasonably well in practice but fails to effectively utilise the information gathered from similar states. Depending upon the smoothness of the action-value function, one approach to overcoming this issue is through online learning, where information is interpolated among similar states; Policy Gradient Search provides a practical algorithm to achieve this. However, Policy Gradient Search lacks an explicit exploration mechanism, which is a key feature of tree-based online search algorithms. In this paper, we propose an efficient and effective online search algorithm called Exploratory Policy Gradient Search (ExPoSe), which leverages information sharing among states by updating the search policy parameters directly, while incorporating a well-defined exploration mechanism during the online search process. We evaluate ExPoSe on a range of decision-making problems, including Atari games, Sokoban, and Hamiltonian cycle search in sparse graphs. The results demonstrate that ExPoSe consistently outperforms other popular online search algorithms across all domains. The ExPoSe source code is available at \textit{\url{https://github.com/dixantmittal/ExPoSe}}.
更多查看译文
关键词
exploration,search,state-based,gradient-based
AI 理解论文
溯源树
样例
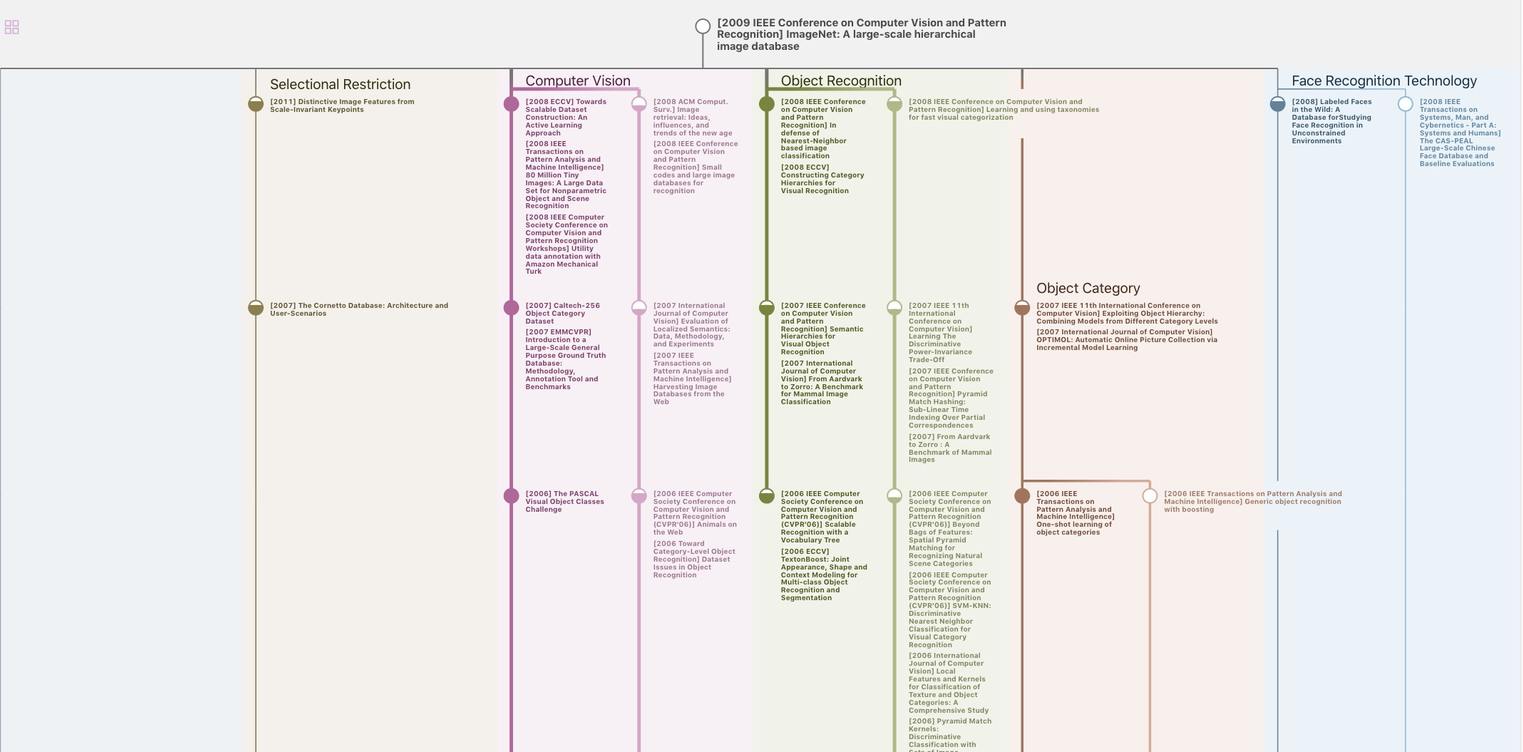
生成溯源树,研究论文发展脉络
Chat Paper
正在生成论文摘要