GALAXY: Graph-based Active Learning at the Extreme.
International Conference on Machine Learning(2022)
摘要
Active learning is a label-efficient approach to train highly effective models while interactively selecting only small subsets of unlabelled data for labelling and training. In “open world" settings, the classes of interest can make up a small fraction of the overall dataset – most of the data may be viewed as an out-of-distribution or irrelevant class. This leads to extreme class-imbalance, and our theory and methods focus on this core issue. We propose a new strategy for active learning called GALAXY (Graph-based Active Learning At the eXtrEme), which blends ideas from graph-based active learning and deep learning. GALAXY automatically and adaptively selects more class-balanced examples for labeling than most other methods for active learning. Our theory shows that GALAXY performs a refined form of uncertainty sampling that gathers a much more class-balanced dataset than vanilla uncertainty sampling. Experimentally, we demonstrate GALAXY’s superiority over existing state-of-art deep active learning algorithms in unbalanced vision classification settings generated from popular datasets.
更多查看译文
关键词
active learning,galaxy,graph-based
AI 理解论文
溯源树
样例
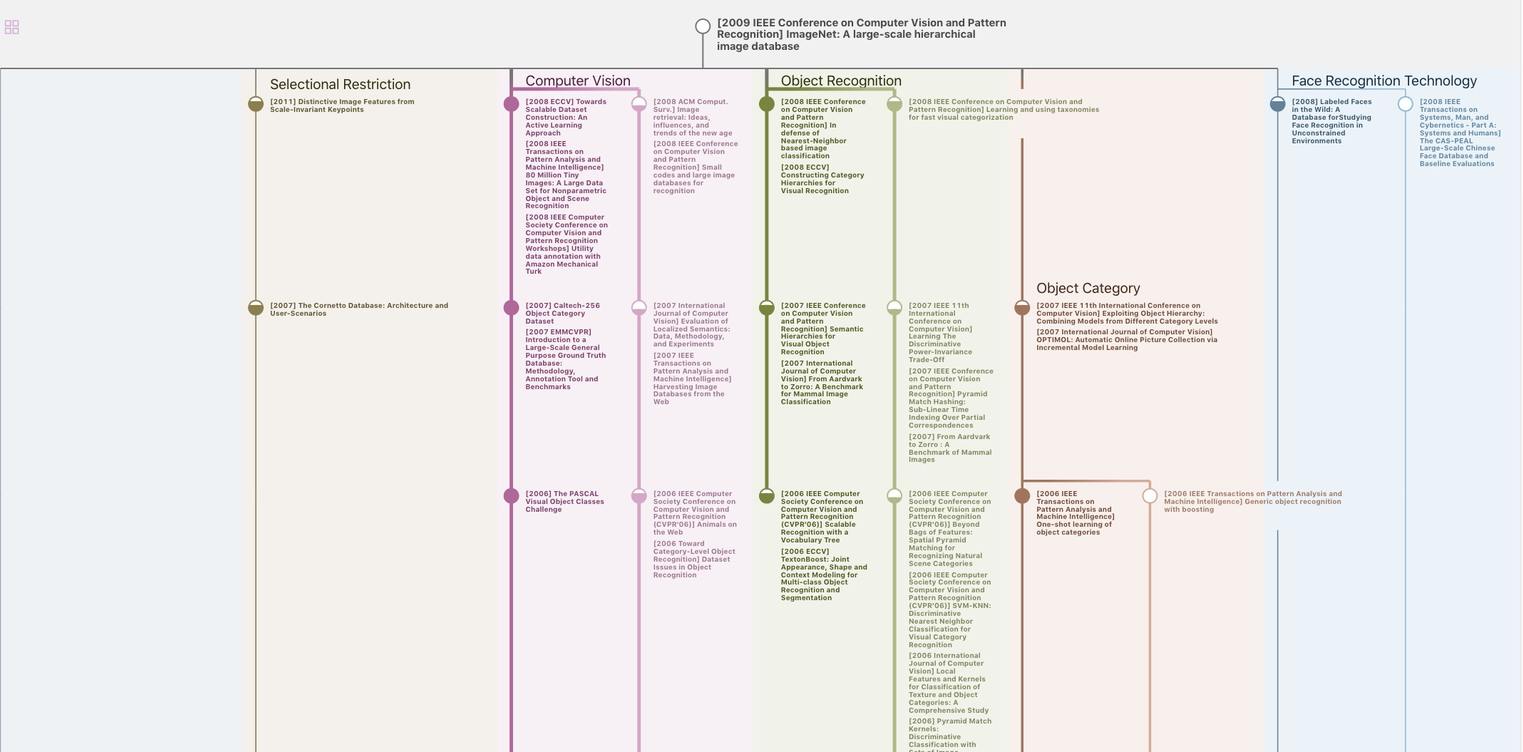
生成溯源树,研究论文发展脉络
Chat Paper
正在生成论文摘要