Autonomous Multi-Step and Multi-Objective Optimization Facilitated by Real-Time Process Analytics
ADVANCED SCIENCE(2022)
摘要
Autonomous flow reactors are becoming increasingly utilized in the synthesis of organic compounds, yet the complexity of the chemical reactions and analytical methods remains limited. The development of a modular platform which uses rapid flow NMR and FTIR measurements, combined with chemometric modeling, is presented for efficient and timely analysis of reaction outcomes. This platform is tested with a four variable single-step reaction (nucleophilic aromatic substitution), to determine the most effective optimization methodology. The self-optimization approach with minimal background knowledge proves to provide the optimal reaction parameters within the shortest operational time. The chosen approach is then applied to a seven variable two-step optimization problem (imine formation and cyclization), for the synthesis of the active pharmaceutical ingredient edaravone. Despite the exponentially increased complexity of this optimization problem, the platform achieves excellent results in a relatively small number of iterations, leading to >95% solution yield of the intermediate and up to 5.42 kg L-1 h(-1) space-time yield for this pharmaceutically relevant product.
更多查看译文
关键词
chemometrics, data-rich experimentation, flow chemistry, machine learning, organic synthesis, process analytical technology, self-optimization
AI 理解论文
溯源树
样例
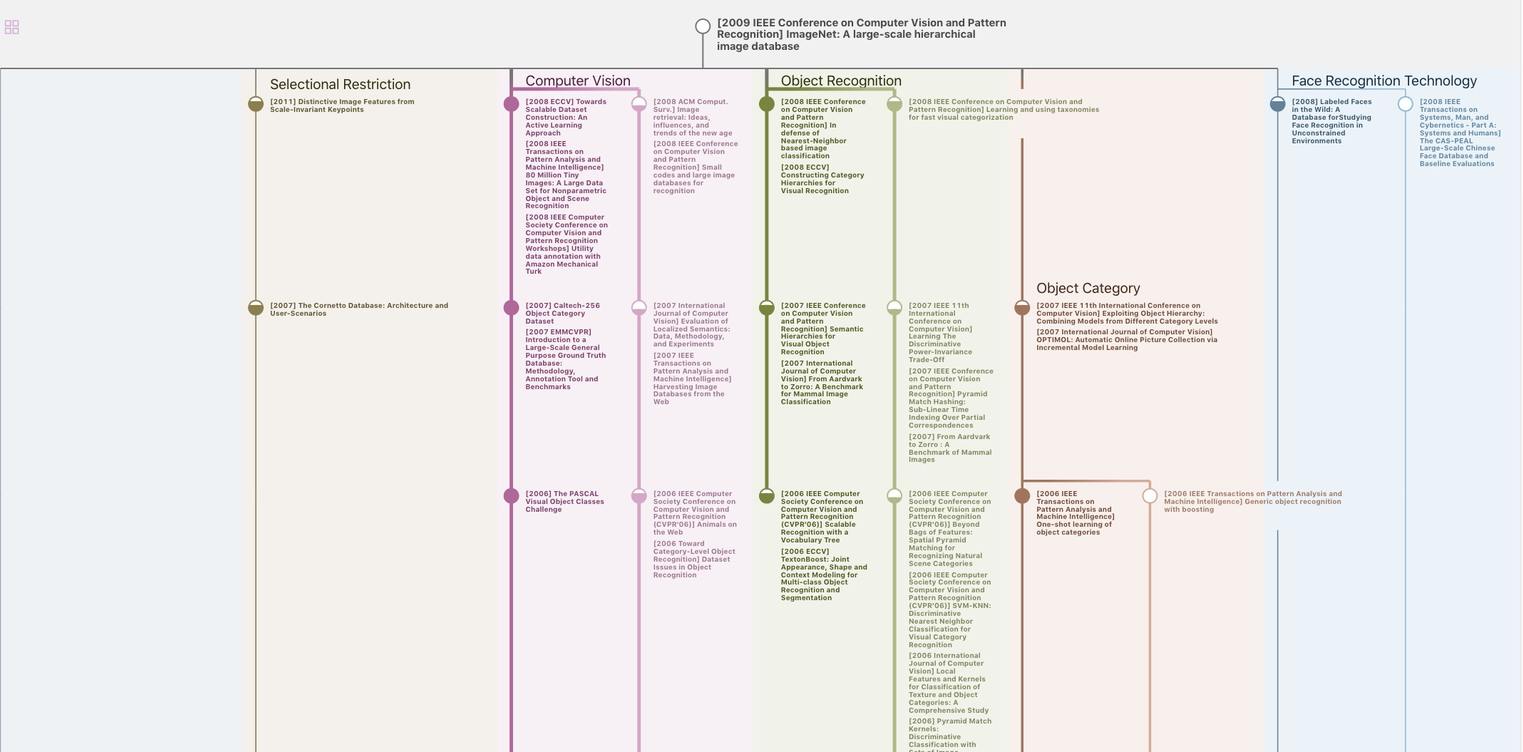
生成溯源树,研究论文发展脉络
Chat Paper
正在生成论文摘要