SEEM: A Sequence Entropy Energy-Based Model for Pedestrian Trajectory All-Then-One Prediction
IEEE Transactions on Pattern Analysis and Machine Intelligence(2023)
摘要
Predicting the future trajectories of pedestrians is of increasing importance for many applications such as autonomous driving and social robots. Nevertheless, current trajectory prediction models suffer from limitations such as lack of diversity in candidate trajectories, poor accuracy, and instability. In this paper, we propose a novel Sequence Entropy Energy-based Model named SEEM, which consists of a generator network and an energy network. Within SEEM we optimize the sequence entropy by taking advantage of the local variational inference of
$f$
-divergence estimation to maximize the mutual information across the generator in order to cover all modes of the trajectory distribution, thereby ensuring SEEM achieves full diversity in candidate trajectory generation. Then, we introduce a probability distribution clipping mechanism to draw samples towards regions of high probability in the trajectory latent space, while our energy network determines which trajectory is most representative of the ground truth. This dual approach is our so-called all-then-one strategy. Finally, a zero-centered potential energy regularization is proposed to ensure stability and convergence of the training process. Through experiments on both synthetic and public benchmark datasets, SEEM is shown to substantially outperform the current state-of-the-art approaches in terms of diversity, accuracy and stability of pedestrian trajectory prediction.
更多查看译文
关键词
Trajectory prediction,mutual information,variational inference,potential energy regularization
AI 理解论文
溯源树
样例
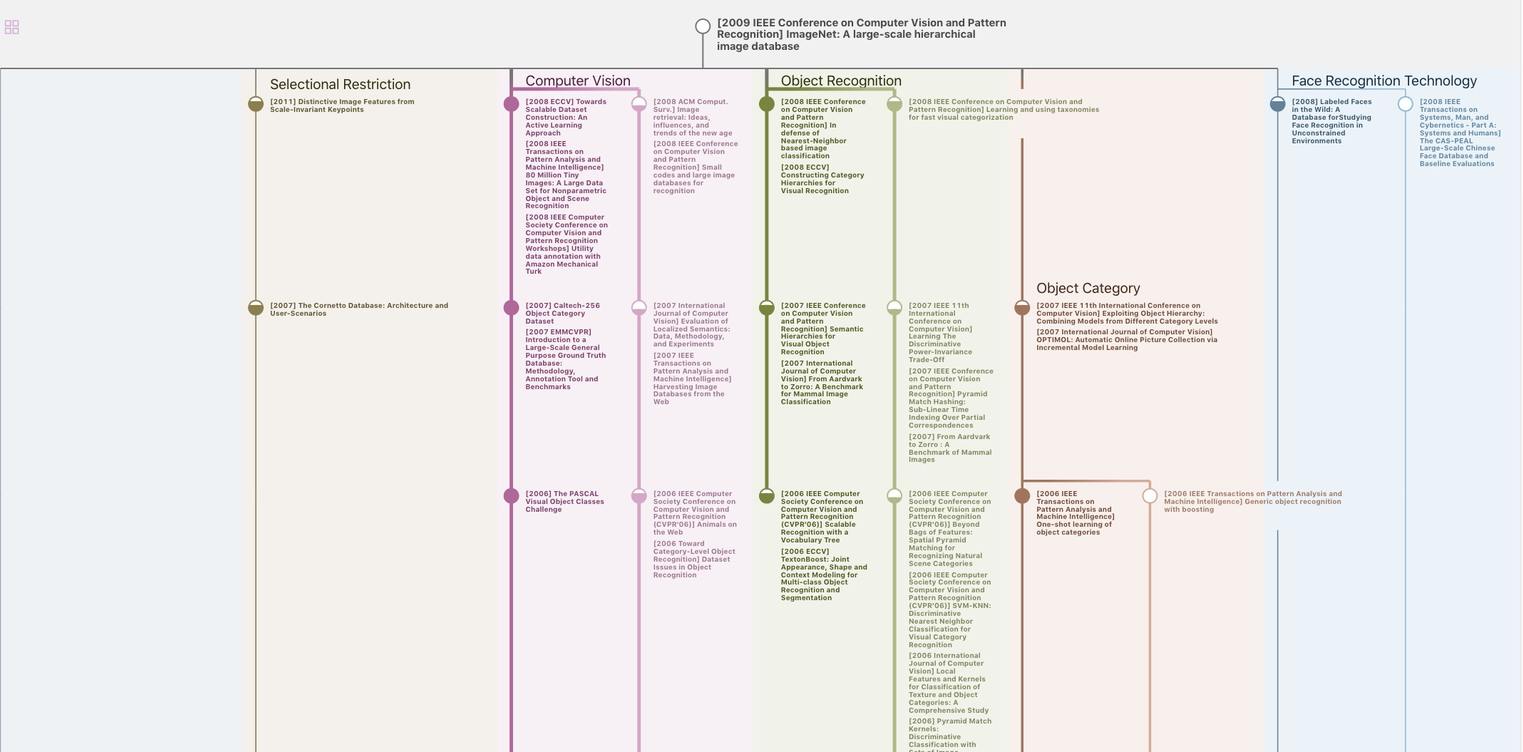
生成溯源树,研究论文发展脉络
Chat Paper
正在生成论文摘要