Federated Reinforcement Learning for Collective Navigation of Robotic Swarms
IEEE TRANSACTIONS ON COGNITIVE AND DEVELOPMENTAL SYSTEMS(2023)
摘要
The recent advancement of deep reinforcement learning (DRL) contributed to robotics by allowing automatic controller design. The automatic controller design is a crucial approach for designing swarm robotic systems, which require more complex controllers than a single robot system to lead a desired collective behavior. Although the DRL-based controller design method showed its effectiveness for swarm robotic systems, the reliance on the central training server is a critical problem in real-world environments where robot-server communication is unstable or limited. We propose a novel federated learning (FL)-based DRL training strategy federated learning DDPG (FLDDPG) for use in swarm robotic applications. Through the comparison with baseline strategies under a limited communication bandwidth scenario, it is shown that the FLDDPG method resulted in higher robustness and generalization ability into a different environment and real robots, while the baseline strategies suffer from the limitation of communication bandwidth. This result suggests that the proposed method can benefit swarm robotic systems operating in environments with limited communication bandwidth, e.g., in high radiation, underwater, or subterranean environments.
更多查看译文
关键词
deep reinforcement learning,federated learning,swarm robotics,Collective navigation
AI 理解论文
溯源树
样例
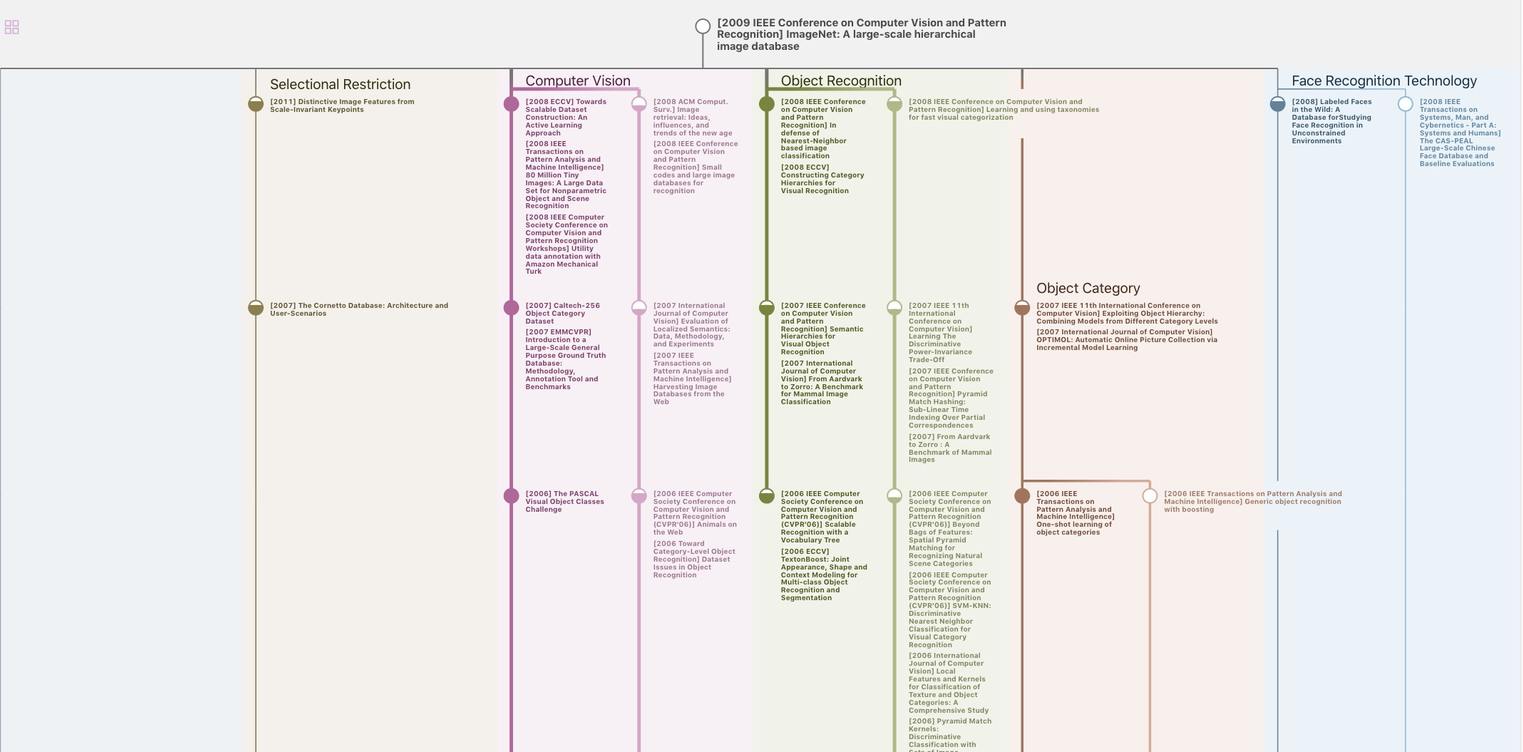
生成溯源树,研究论文发展脉络
Chat Paper
正在生成论文摘要