Tailoring Gradient Methods for Differentially Private Distributed Optimization
IEEE TRANSACTIONS ON AUTOMATIC CONTROL(2024)
摘要
Decentralized optimization is gaining increased traction due to its widespread applications in large-scale machine learning and multiagent systems. The same mechanism that enables its success, i.e., information sharing among participating agents, however, also leads to the disclosure of individual agents' private information, which is unacceptable when sensitive data are involved. As differential privacy is becoming a de facto standard for privacy preservation, recently results have emerged integrating differential privacy with distributed optimization. However, directly incorporating differential privacy design in existing distributed optimization approaches significantly compromises optimization accuracy. In this article, we propose to redesign and tailor gradient methods for differentially private distributed optimization, and propose two differential-privacy oriented gradient methods that can ensure both rigorous is an element of-differential privacy and optimality. The first algorithm is based on static-consensus-based gradient methods, and the second algorithm is based on dynamic-consensus (gradient-tracking) based distributed optimization methods and, hence, is applicable to general directed interaction graph topologies. Both algorithms can simultaneously ensure almost sure convergence to an optimal solution and a finite privacy budget, even when the number of iterations goes to infinity. To the best of authors' knowledge, this is the first time that both goals are achieved simultaneously. Numerical simulations using a distributed estimation problem and experimental results on a benchmark dataset confirm the effectiveness of the proposed approaches.
更多查看译文
关键词
Decentralized learning,decentralized optimization,differential privacy,gradient methods
AI 理解论文
溯源树
样例
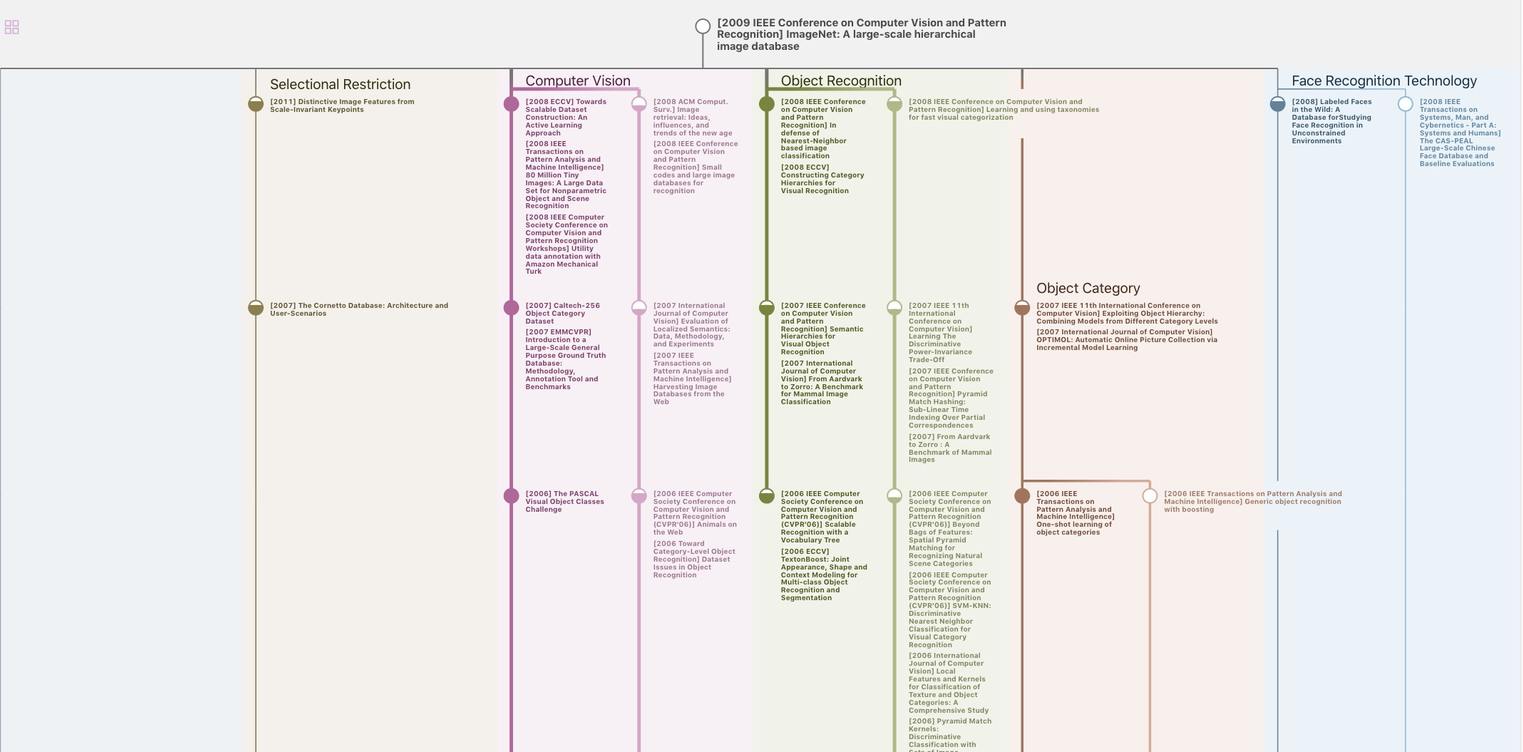
生成溯源树,研究论文发展脉络
Chat Paper
正在生成论文摘要