Diagnosing failures of fairness transfer across distribution shift in real-world medical settings
arxiv(2023)
摘要
Diagnosing and mitigating changes in model fairness under distribution shift is an important component of the safe deployment of machine learning in healthcare settings. Importantly, the success of any mitigation strategy strongly depends on the structure of the shift. Despite this, there has been little discussion of how to empirically assess the structure of a distribution shift that one is encountering in practice. In this work, we adopt a causal framing to motivate conditional independence tests as a key tool for characterizing distribution shifts. Using our approach in two medical applications, we show that this knowledge can help diagnose failures of fairness transfer, including cases where real-world shifts are more complex than is often assumed in the literature. Based on these results, we discuss potential remedies at each step of the machine learning pipeline.
更多查看译文
关键词
Healthcare,fairness,robustness,deep learning
AI 理解论文
溯源树
样例
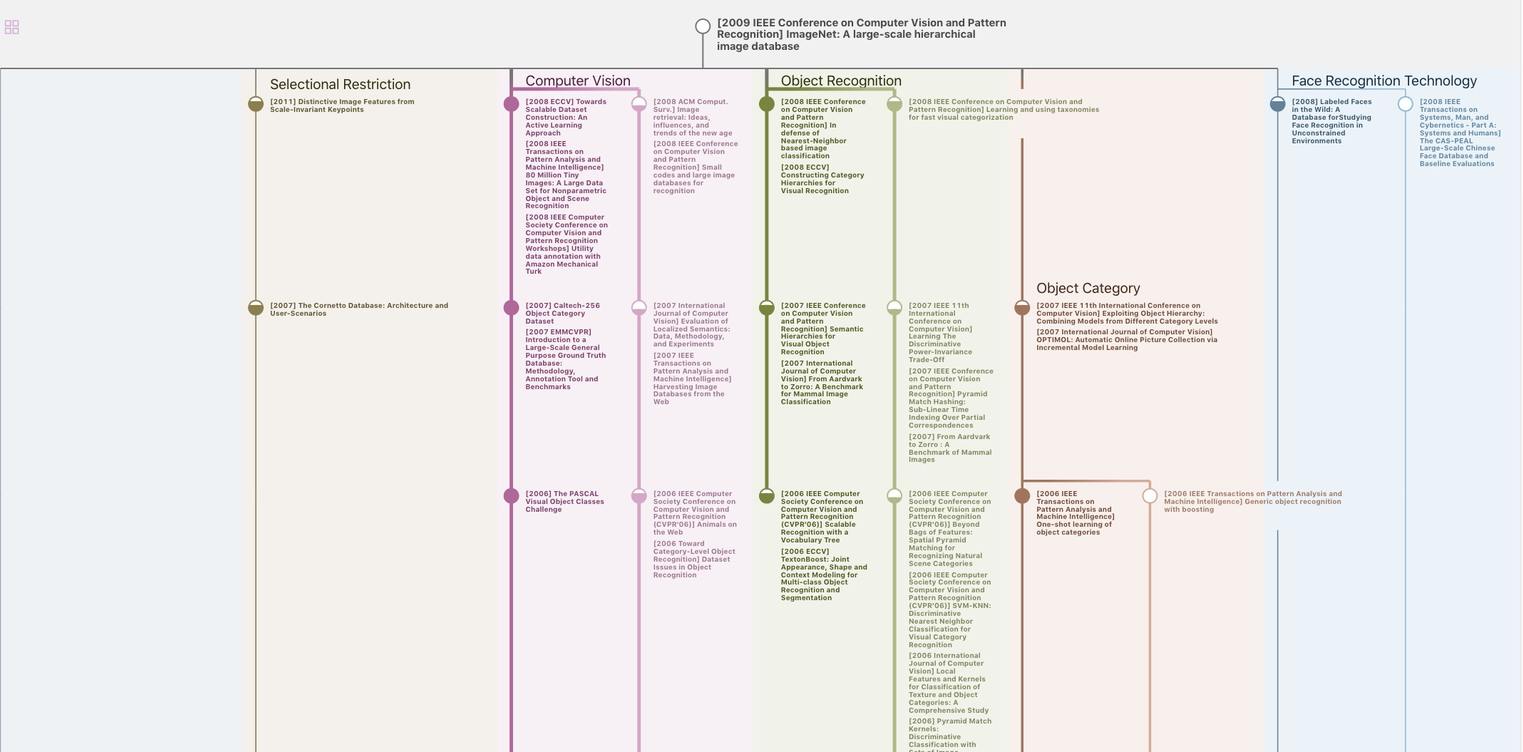
生成溯源树,研究论文发展脉络
Chat Paper
正在生成论文摘要