Long-Horizon Return Predictability from Realized Volatility in Pure-Jump Point Processes
arxiv(2022)
摘要
We develop and justify methodology to consistently test for long-horizon return predictability based on realized variance. To accomplish this, we propose a parametric transaction-level model for the continuous-time log price process based on a pure jump point process. The model determines the returns and realized variance at any level of aggregation with properties shown to be consistent with the stylized facts in the empirical finance literature. Under our model, the long-memory parameter propagates unchanged from the transaction-level drift to the calendar-time returns and the realized variance, leading endogenously to a balanced predictive regression equation. We propose an asymptotic framework using power-law aggregation in the predictive regression. Within this framework, we propose a hypothesis test for long horizon return predictability which is asymptotically correctly sized and consistent.
更多查看译文
关键词
realized volatility,long-horizon,pure-jump
AI 理解论文
溯源树
样例
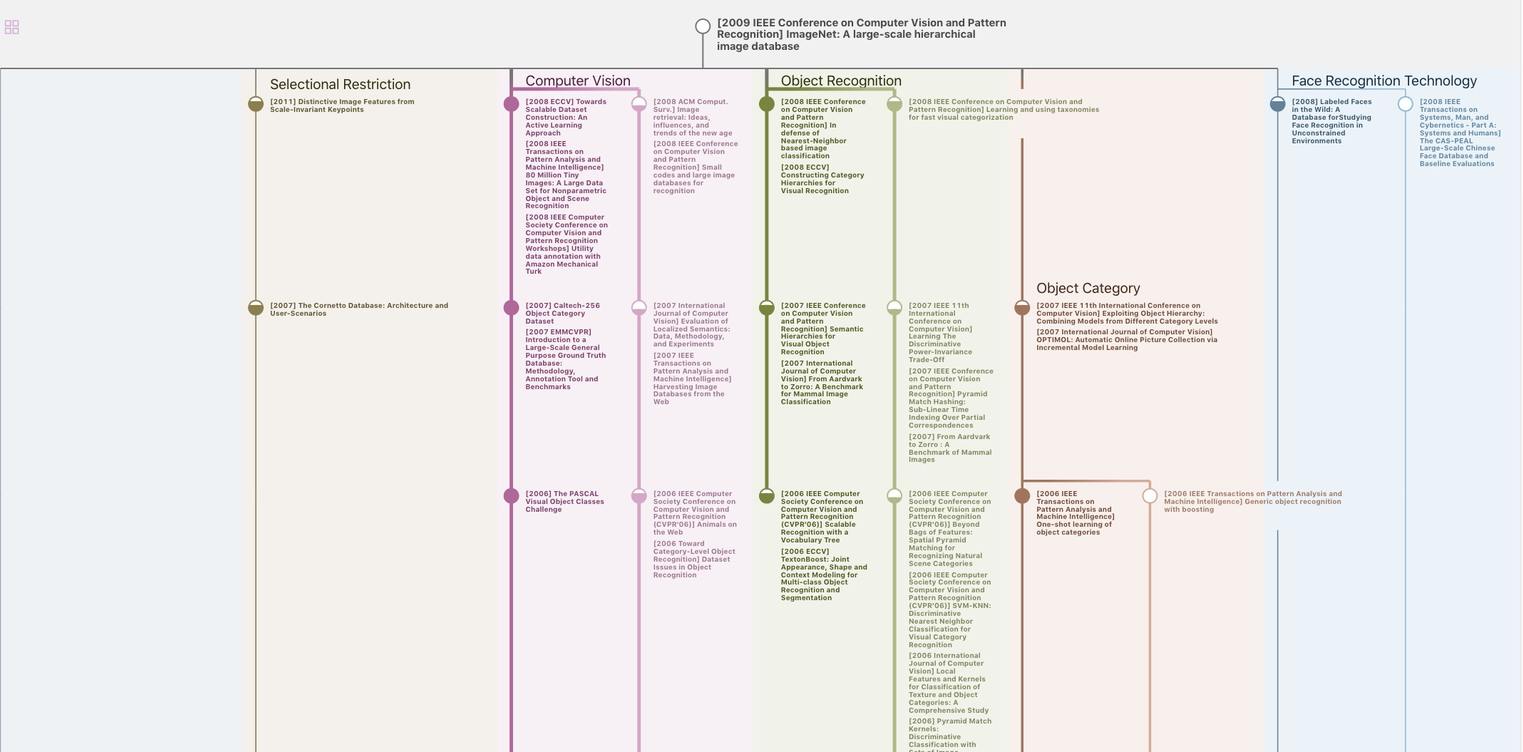
生成溯源树,研究论文发展脉络
Chat Paper
正在生成论文摘要