Synergistic combination of a topologically invariant imaging signature and a biomarker for the accurate prediction of symptomatic radiation pneumonitis before stereotactic ablative radiotherapy for lung cancer: A retrospective analysis
PLOS ONE(2022)
摘要
Objectives We aimed to explore the synergistic combination of a topologically invariant Betti number (BN)-based signature and a biomarker for the accurate prediction of symptomatic (grade >= 2) radiation-induced pneumonitis (RP+) before stereotactic ablative radiotherapy (SABR) for lung cancer. Methods A total of 272 SABR cases with early-stage non-small cell lung cancer were chosen for this study. The occurrence of RP+ was predicted using a support vector machine (SVM) model trained with the combined features of the BN-based signature extracted from planning computed tomography (pCT) images and a pretreatment biomarker, serum Krebs von den Lungen-6 (BN+KL-6 model). In all, 242 (20 RP+ and 222 RP-(grade 1)) and 30 cases (8 RP+ and 22 RP-) were used for training and testing the model, respectively. The BN-based features were extracted from BN maps that characterize topologically invariant heterogeneous traits of potential RP+ lung regions on pCT images by applying histogram- and texture-based feature calculations to the maps. The SVM models were built to predict RP+ patients with a BN signature that was constructed based on the least absolute shrinkage and selection operator logistic regression model. The evaluation of the prediction models was performed based on the area under the receiver operating characteristic curves (AUCs) and accuracy in the test. The performance of the BN+KL-6 model was compared to the performance based on the BN, conventional original pCT, and wavelet decomposition (WD) models. Results The test AUCs obtained for the BN+KL-6, BN, pCT, and WD models were 0.825, 0.807, 0.642, and 0.545, respectively. The accuracies of the BN+KL-6, BN, pCT, and WD models were found to be 0.724, 0.708, 0.591, and 0.534, respectively. Conclusion This study demonstrated the comprehensive performance of the BN+KL-6 model for the prediction of potential RP+ patients before SABR for lung cancer.
更多查看译文
AI 理解论文
溯源树
样例
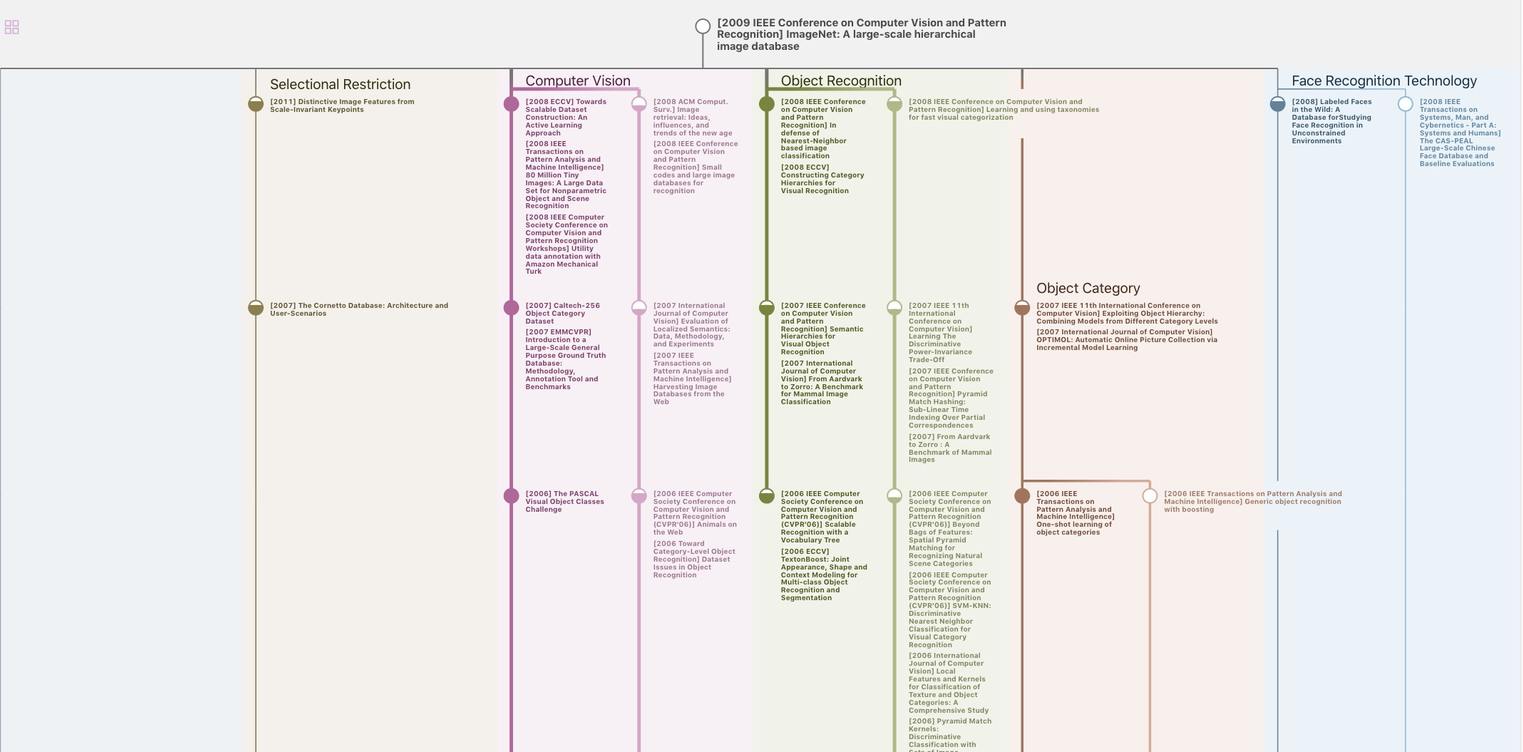
生成溯源树,研究论文发展脉络
Chat Paper
正在生成论文摘要