Is the Performance of My Deep Network Too Good to Be True? A Direct Approach to Estimating the Bayes Error in Binary Classification
ICLR 2023(2022)
摘要
There is a fundamental limitation in the prediction performance that a machine learning model can achieve due to the inevitable uncertainty of the prediction target. In classification problems, this can be characterized by the Bayes error, which is the best achievable error with any classifier. The Bayes error can be used as a criterion to evaluate classifiers with state-of-the-art performance and can be used to detect test set overfitting. We propose a simple and direct Bayes error estimator, where we just take the mean of the labels that show \emph{uncertainty} of the classes. Our flexible approach enables us to perform Bayes error estimation even for weakly supervised data. In contrast to others, our method is model-free and even instance-free. Moreover, it has no hyperparameters and gives a more accurate estimate of the Bayes error than classifier-based baselines. Experiments using our method suggest that a recently proposed classifier, the Vision Transformer, may have already reached the Bayes error for certain benchmark datasets.
更多查看译文
关键词
Bayes error,best achievable error,irreducible error
AI 理解论文
溯源树
样例
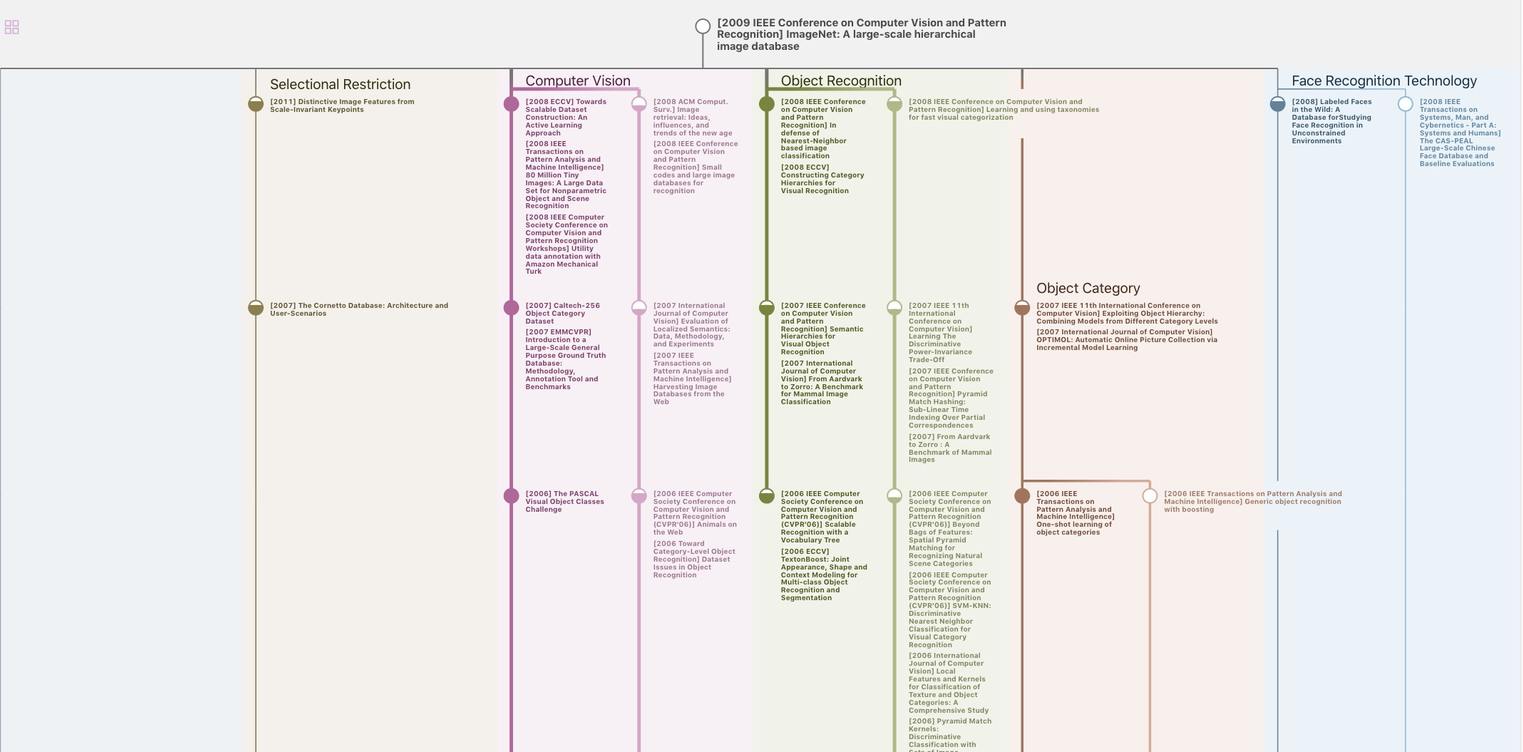
生成溯源树,研究论文发展脉络
Chat Paper
正在生成论文摘要