Explainable AI through the Learning of Arguments
arxiv(2022)
摘要
Learning arguments is highly relevant to the field of explainable artificial intelligence. It is a family of symbolic machine learning techniques that is particularly human-interpretable. These techniques learn a set of arguments as an intermediate representation. Arguments are small rules with exceptions that can be chained to larger arguments for making predictions or decisions. We investigate the learning of arguments, specifically the learning of arguments from a 'case model' proposed by Verheij [34]. The case model in Verheij's approach are cases or scenarios in a legal setting. The number of cases in a case model are relatively low. Here, we investigate whether Verheij's approach can be used for learning arguments from other types of data sets with a much larger number of instances. We compare the learning of arguments from a case model with the HeRO algorithm [15] and learning a decision tree.
更多查看译文
关键词
explainable explainable,arguments,learning
AI 理解论文
溯源树
样例
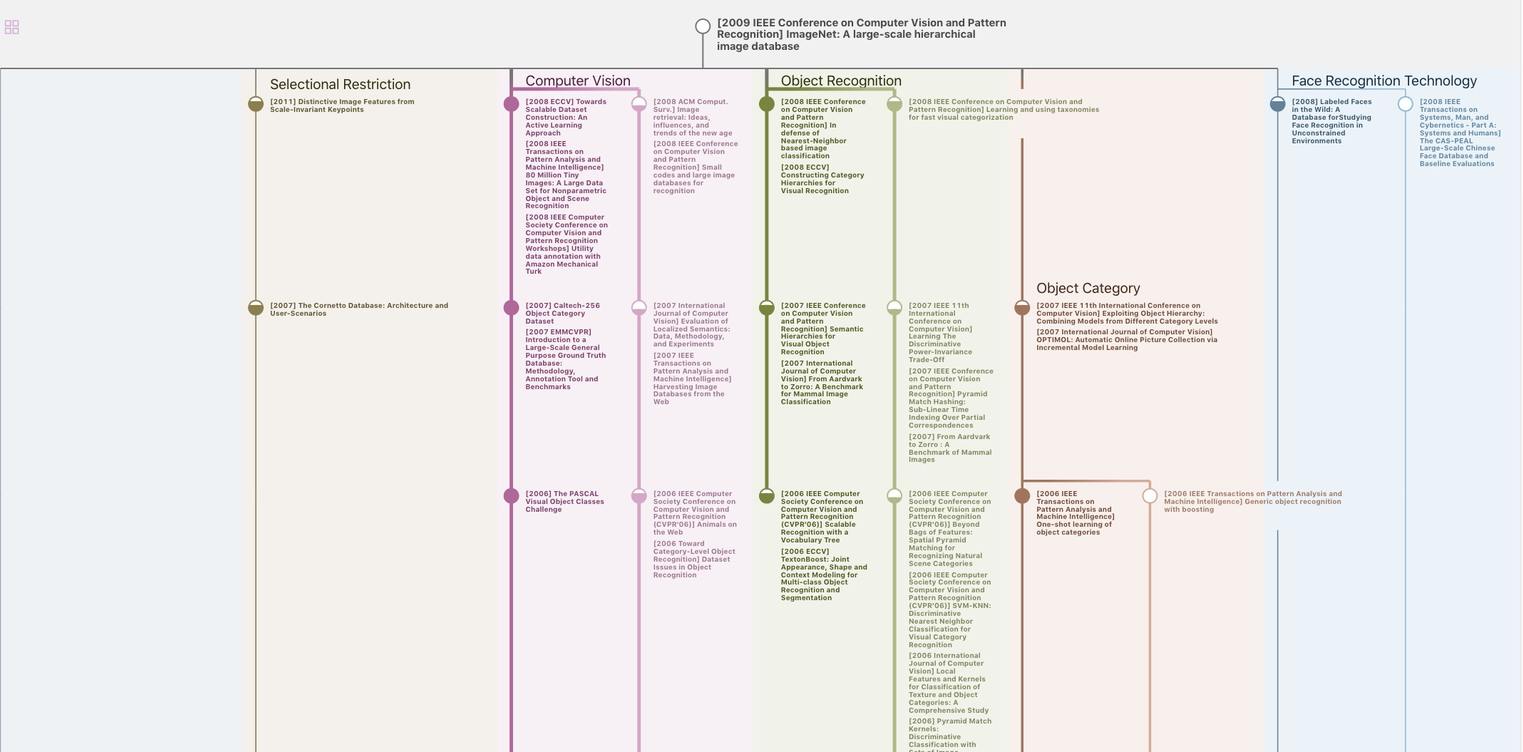
生成溯源树,研究论文发展脉络
Chat Paper
正在生成论文摘要