Accelerating Deep Reinforcement Learning for Digital Twin Network Optimization with Evolutionary Strategies
PROCEEDINGS OF THE IEEE/IFIP NETWORK OPERATIONS AND MANAGEMENT SYMPOSIUM 2022(2022)
摘要
The recent growth of emergent network applications (e.g., satellite networks, vehicular networks) is increasing the complexity of managing modern communication networks. As a result, the community proposed the Digital Twin Networks (DTN) as a key enabler of efficient network management. Network operators can leverage the DTN to perform different optimization tasks (e.g., Traffic Engineering, Network Planning). Deep Reinforcement Learning (DRL) showed a high performance when applied to solve network optimization problems. In the context of DTN, DRL can be leveraged to solve optimization problems without directly impacting the real-world network behavior. However, DRL scales poorly with the problem size and complexity. In this paper, we explore the use of Evolutionary Strategies (ES) to train DRL agents for solving a routing optimization problem. The experimental results show that ES achieved a training time speed-up of 128 and 6 for the NSFNET and GEANT2 topologies respectively.
更多查看译文
关键词
Deep Reinforcement Learning, Digital Twin Network, Optimization, Evolutionary Strategies
AI 理解论文
溯源树
样例
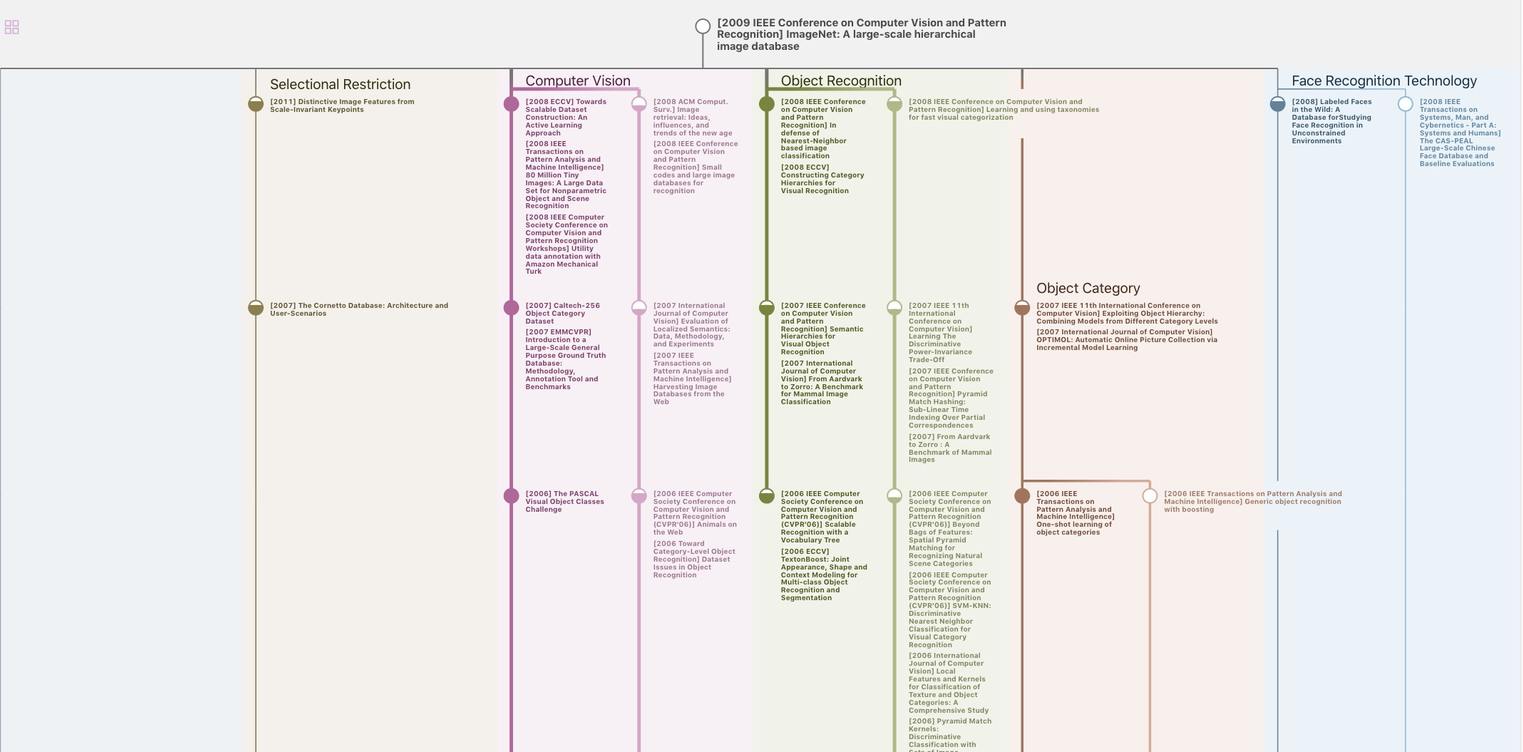
生成溯源树,研究论文发展脉络
Chat Paper
正在生成论文摘要