Architecture Matters in Continual Learning
ICLR 2023(2022)
摘要
A large body of research in continual learning is devoted to overcoming the catastrophic forgetting of neural networks by designing new algorithms that are robust to the distribution shifts. However, the majority of these works are strictly focused on the "algorithmic" part of continual learning for a "fixed neural network architecture", and the implications of using different architectures are mostly neglected. Even the few existing continual learning methods that modify the model assume a fixed architecture and aim to develop an algorithm that efficiently uses the model throughout the learning experience. However, in this work, we show that the choice of architecture can significantly impact the continual learning performance, and different architectures lead to different trade-offs between the ability to remember previous tasks and learning new ones. Moreover, we study the impact of various architectural decisions, and our findings entail best practices and recommendations that can improve the continual learning performance.
更多查看译文
关键词
Continual Learning,Catastrophic Forgetting,Neural Network Architecture
AI 理解论文
溯源树
样例
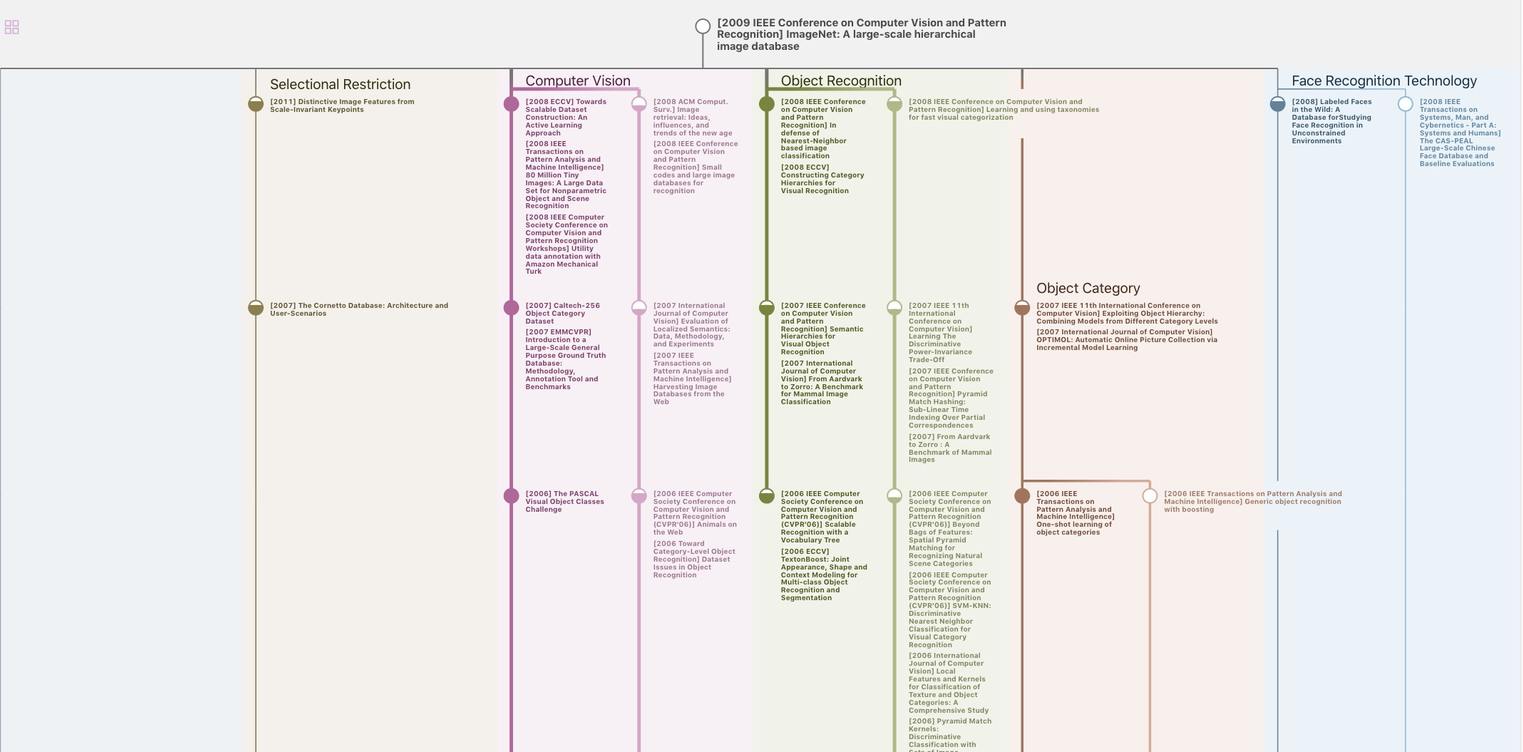
生成溯源树,研究论文发展脉络
Chat Paper
正在生成论文摘要