Neural Collaborative Filtering Bandits via Meta Learning
ICLR 2023(2022)
摘要
Contextual multi-armed bandits provide powerful tools to solve the exploitation-exploration dilemma in decision making, with direct applications in the personalized recommendation. In fact, collaborative effects among users carry the significant potential to improve the recommendation. In this paper, we introduce and study the problem by exploring `Neural Collaborative Filtering Bandits', where the rewards can be non-linear functions and groups are formed dynamically given different specific contents. To solve this problem, inspired by meta-learning, we propose Meta-Ban (meta-bandits), where a meta-learner is designed to represent and rapidly adapt to dynamic groups, along with a UCB-based exploration strategy. Furthermore, we analyze that Meta-Ban can achieve the regret bound of $\mathcal{O}(\sqrt{T \log T})$, improving a multiplicative factor $\sqrt{\log T}$ over state-of-the-art related works. In the end, we conduct extensive experiments showing that Meta-Ban significantly outperforms six strong baselines.
更多查看译文
关键词
Neural Contextual Bandit,Meta Learning
AI 理解论文
溯源树
样例
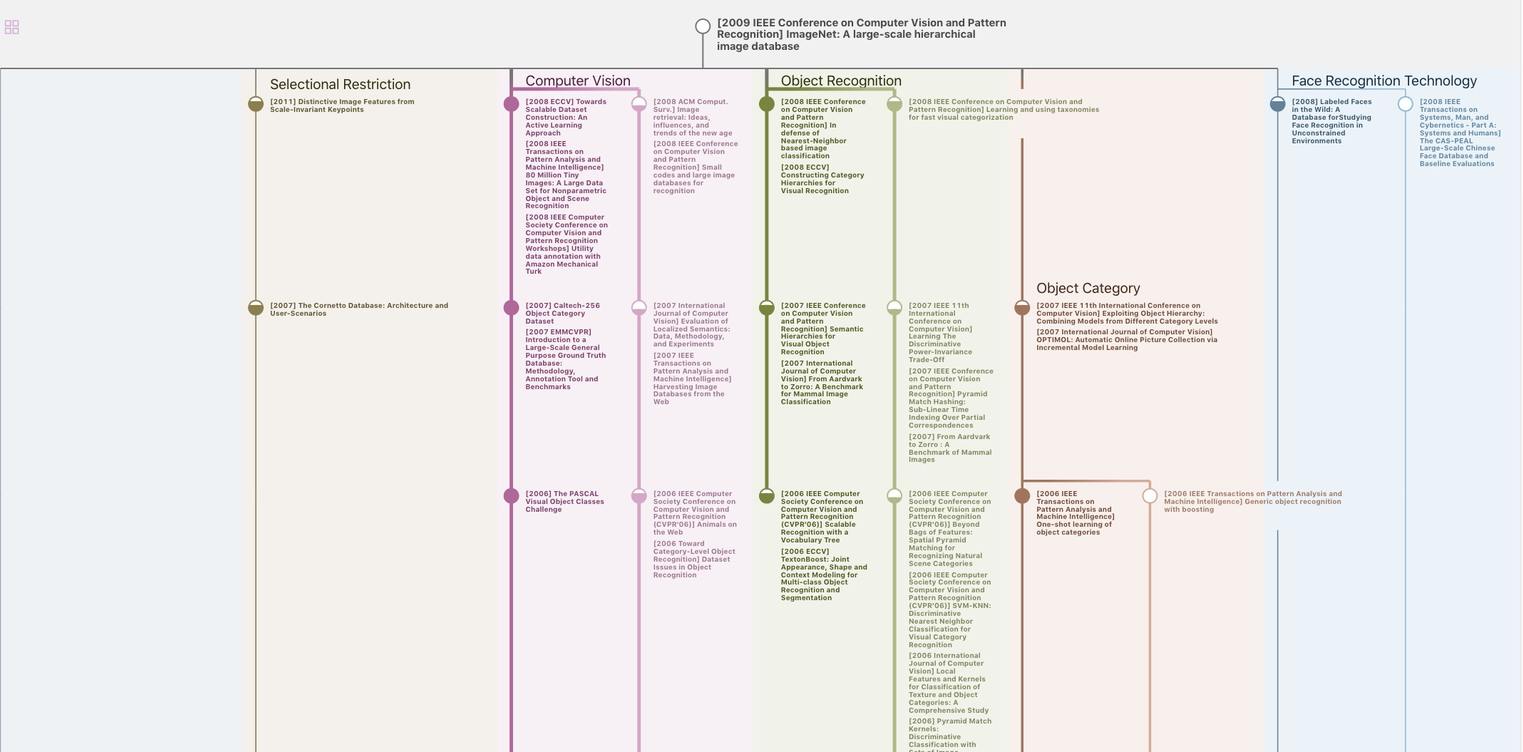
生成溯源树,研究论文发展脉络
Chat Paper
正在生成论文摘要