Filtering In Neural Implicit Functions
arxiv(2022)
摘要
Neural implicit functions are highly effective for data representation. However, the implicit functions learned by neural networks usually include unexpected noisy artifacts or lose fine details if the input data has many scales of detail or contains both low-frequency and high-frequency bandwidths. Removing artifacts while preserving fine-scale contents is challenging and usually comes out with over-smoothing or noisy issues. To solve this dilemma, we propose a new framework (FINN) that integrates a filtering module into the MLPs to perform data reconstruction while adapting regions containing different frequencies. The filtering module has a smoothing operator acting on intermediate results of the network that encourages the results to be smooth and a recovering operator bringing high frequencies to regions overly smooth. The two counteractive operators play consecutively in all MLP layers to adaptively influence the reconstruction. We demonstrate the advantage of FINN on several tasks and showcase significant improvement compared to state-of-the-art methods. In addition, FINN also yields better performance in both convergence speed and network stability.
更多查看译文
AI 理解论文
溯源树
样例
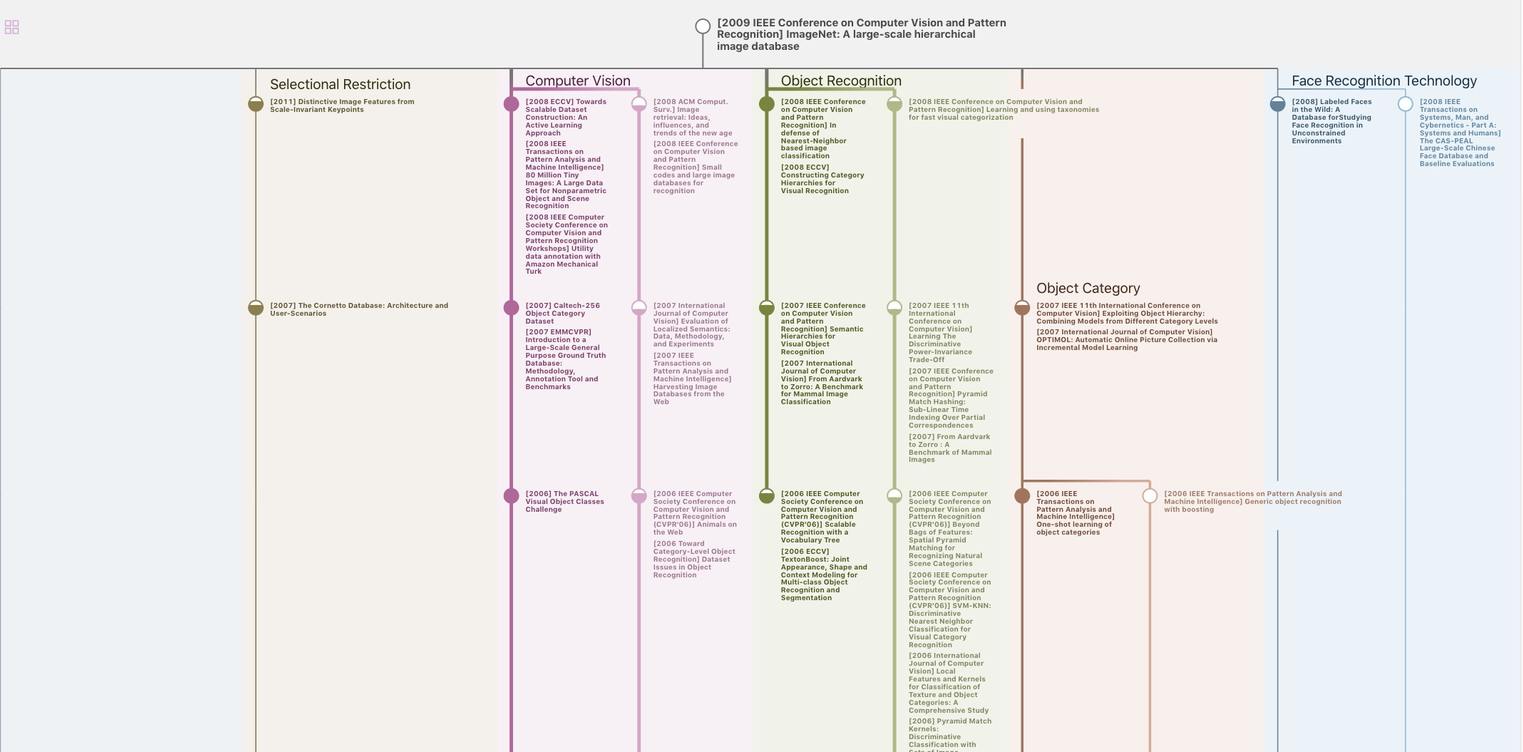
生成溯源树,研究论文发展脉络
Chat Paper
正在生成论文摘要