COIN++: Neural Compression Across Modalities
arxiv(2022)
摘要
Neural compression algorithms are typically based on autoencoders that require specialized encoder and decoder architectures for different data modalities. In this paper, we propose COIN++, a neural compression framework that seamlessly handles a wide range of data modalities. Our approach is based on converting data to implicit neural representations, i.e. neural functions that map coordinates (such as pixel locations) to features (such as RGB values). Then, instead of storing the weights of the implicit neural representation directly, we store modulations applied to a meta-learned base network as a compressed code for the data. We further quantize and entropy code these modulations, leading to large compression gains while reducing encoding time by two orders of magnitude compared to baselines. We empirically demonstrate the feasibility of our method by compressing various data modalities, from images and audio to medical and climate data.
更多查看译文
关键词
neural compression across modalities
AI 理解论文
溯源树
样例
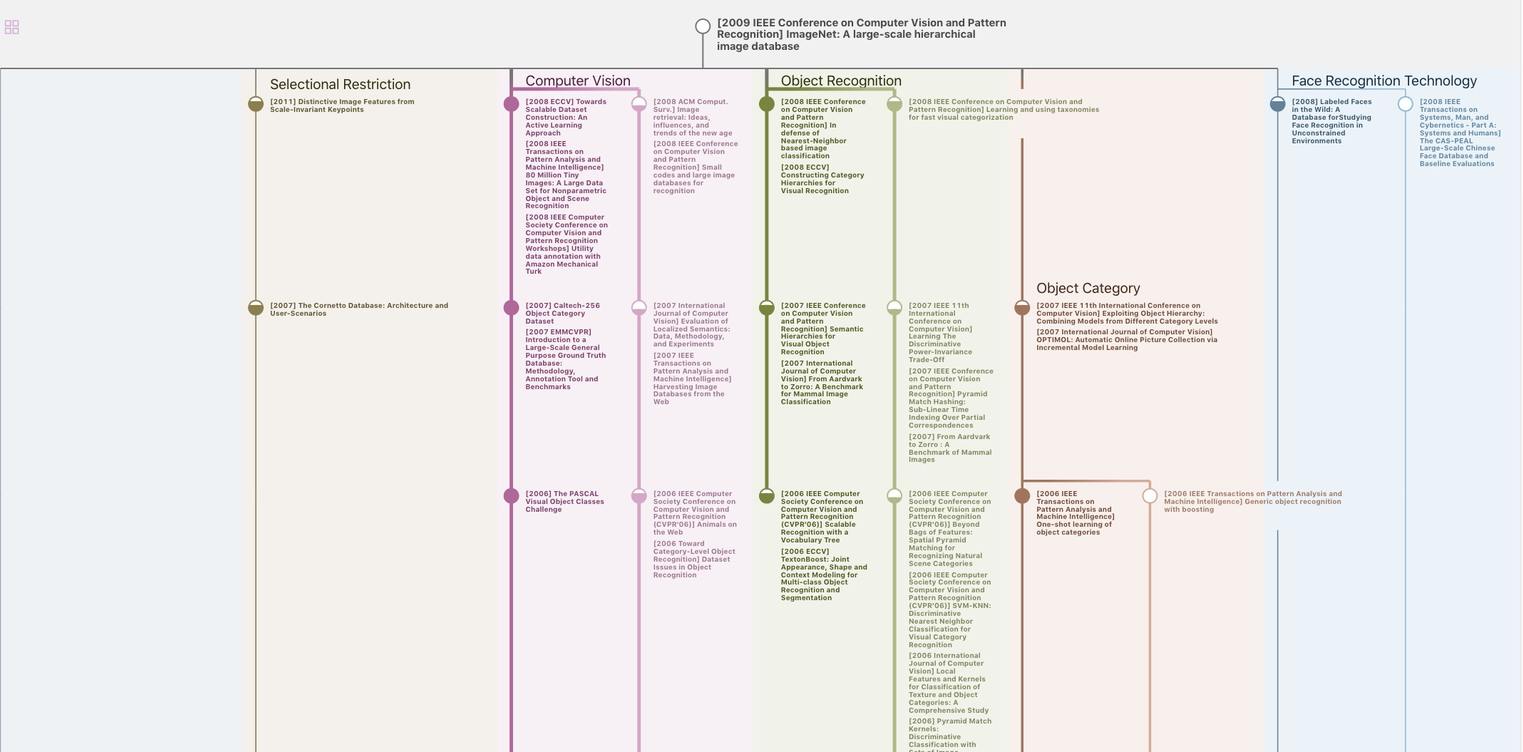
生成溯源树,研究论文发展脉络
Chat Paper
正在生成论文摘要