Machine Learning Study of the Magnetic Ordering in 2D Materials
ACS APPLIED MATERIALS & INTERFACES(2022)
摘要
Magnetic materials have been applied in a large variety of technologies, from data storage to quantum devices. The development of two-dimensional (2D) materials has opened new arenas for magnetic compounds, even when classical theories discourage their examination. Here we propose a machinelearning-based strategy to predict and understand magnetic ordering in 2D materials. This strategy couples the prediction of the existence of magnetism in 2D materials using a random forest and the Shapley additive explanations method with material maps defined by atomic features predicting the magnetic ordering (ferromagnetic or antiferromagnetic). While the random forest model predicts magnetism with an accuracy of 86%, the material maps obtained by the sure independence screening and sparsifying method have an accuracy of similar to 90% in predicting the magnetic ordering. Our model indicates that 3d transition metals, halides, and structural clusters with regular transition-metal sublattices have a positive contribution in the total weight deciding the existence of magnetism in 2D compounds. This behavior is associated with the competition between crystal field and exchange splitting. The machine learning model also indicates that the atomic spin orbit coupling (SOC) is a determinant feature for the identification of the patterns separating ferro- from antiferromagnetic order. The proposed strategy is used to identify novel 2D magnetic compounds that, together with the fundamental trends in the chemical and structural space, pave novel routes for experimental exploration.
更多查看译文
关键词
two-dimensional materials, machine learning, high-throughput screening, magnetism, ferromagnetic, antiferromagnetic
AI 理解论文
溯源树
样例
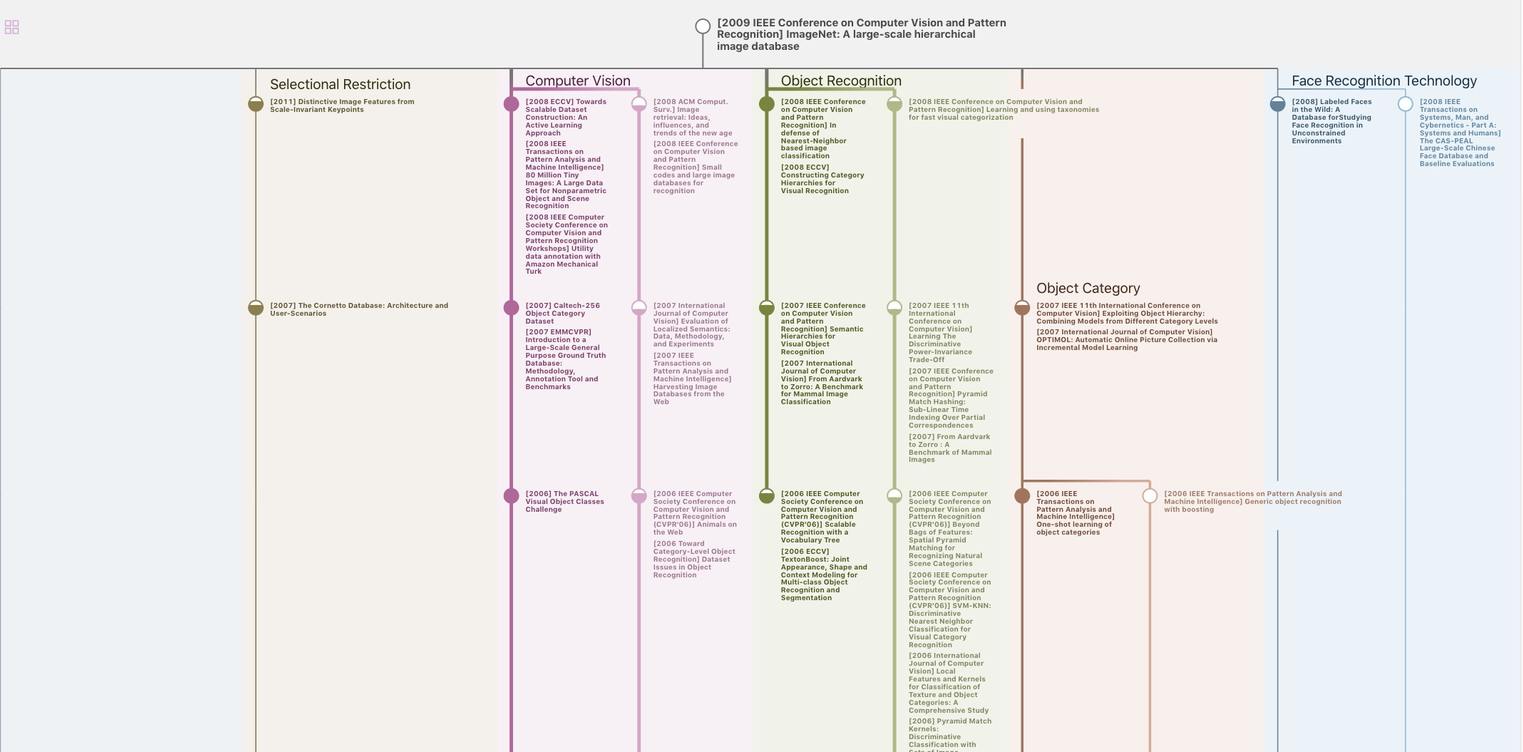
生成溯源树,研究论文发展脉络
Chat Paper
正在生成论文摘要