Comparing trained and untrained probabilistic ensemble forecasts of COVID-19 cases and deaths in the United States.
International journal of forecasting(2022)
摘要
The U.S. COVID-19 Forecast Hub aggregates forecasts of the short-term burden of COVID-19 in the United States from many contributing teams. We study methods for building an ensemble that combines forecasts from these teams. These experiments have informed the ensemble methods used by the Hub. To be most useful to policy makers, ensemble forecasts must have stable performance in the presence of two key characteristics of the component forecasts: (1) occasional misalignment with the reported data, and (2) instability in the relative performance of component forecasters over time. Our results indicate that in the presence of these challenges, an untrained and robust approach to ensembling using an equally weighted median of all component forecasts is a good choice to support public health decision makers. In settings where some contributing forecasters have a stable record of good performance, trained ensembles that give those forecasters higher weight can also be helpful.
更多查看译文
关键词
COVID-19,Ensemble,Epidemiology,Health forecasting,Quantile combination
AI 理解论文
溯源树
样例
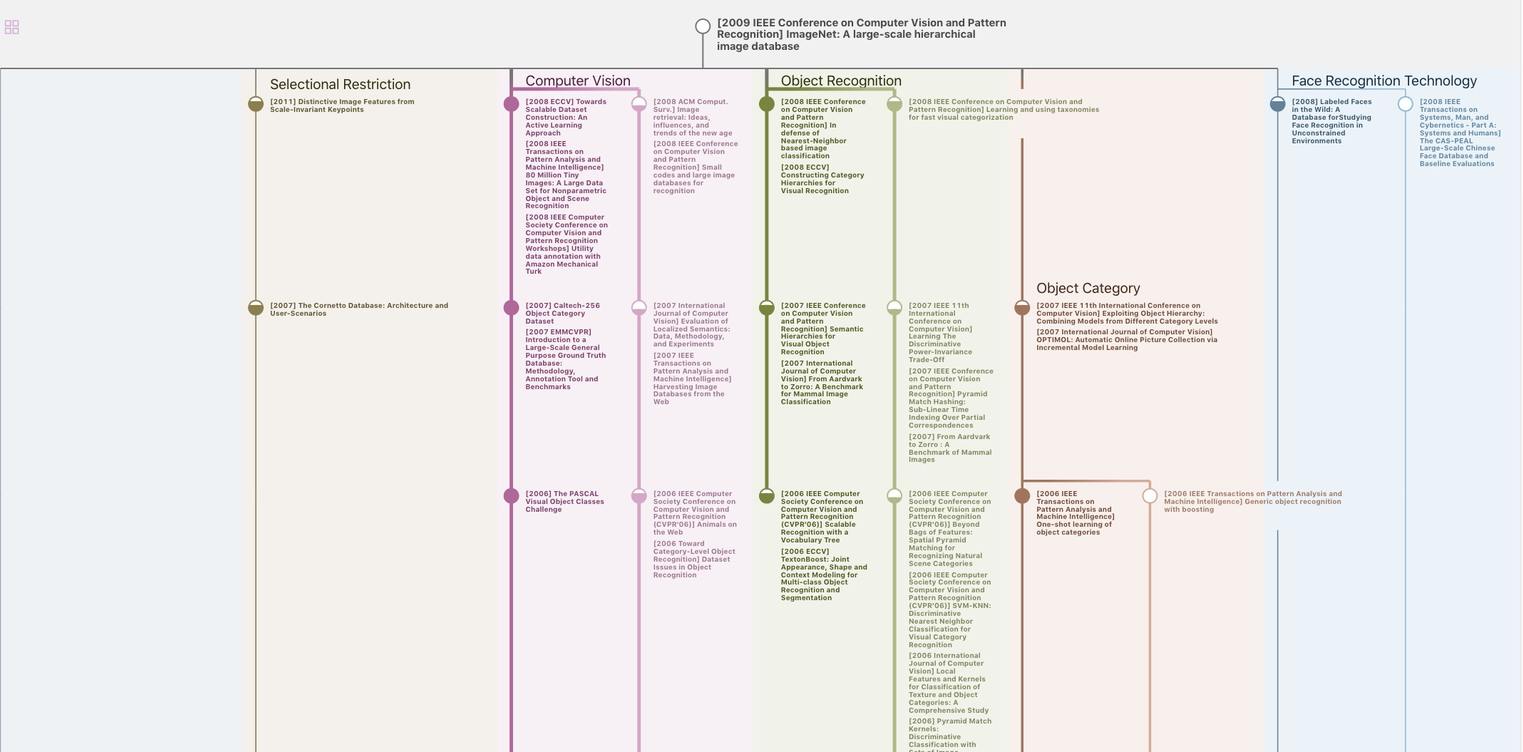
生成溯源树,研究论文发展脉络
Chat Paper
正在生成论文摘要