Information effects on lay tradeoffs between national regulatory costs and benefits.
Risk analysis : an official publication of the Society for Risk Analysis(2022)
摘要
A novel stated-preference "macro-risk" approach introduced to estimate the life-prolonging benefits of proposed environmental, health, and safety regulations may answer questions unasked or wrongly answered by conventional revealed-preference (e.g., "wage premiums" for high occupational risks) and stated-preference methods (e.g., willingness to pay for tiny reductions in one's own premature death risk). This new approach asks laypeople to appraise directly their preferred tradeoffs between national regulatory costs and lives prolonged nationwide (regulatory benefits). However, this method may suffer from incomplete lay understanding of national-scale consequences (e.g., billions of dollars in regulatory costs; hundreds of lives prolonged) or tradeoffs (e.g., what are lives prolonged worth?). Here we (1) tested effects of numerical contextual examples to ground each hypothetical regulatory tradeoff, and (2) explored why some people implicitly offer "implausible" values (< $10,000 or > $1 billion) for the social benefit of prolonging one life. In Study 1 (n = 356), after testing their separate effects, we combined three contextual-information aids: (1) comparing hypothetical regulatory costs and benefits to real-life higher and lower values; (2) reframing large numbers into smaller, more familiar terms; and (3) framing regulatory costs as having diffuse versus concentrated impacts. Information increased social benefits values on average (from $4.5 million to $13.8 million). Study 2 (n = 402) found that the most common explanations for "implausible" values included inattention, strong attitudes about regulation, and problems translating values into responses. We discuss implications for this novel stated-preferences method, and for comparing it to micro-risk methods.
更多查看译文
关键词
calibration,cost-benefit analysis,national tradeoffs,stated preferences,valuation
AI 理解论文
溯源树
样例
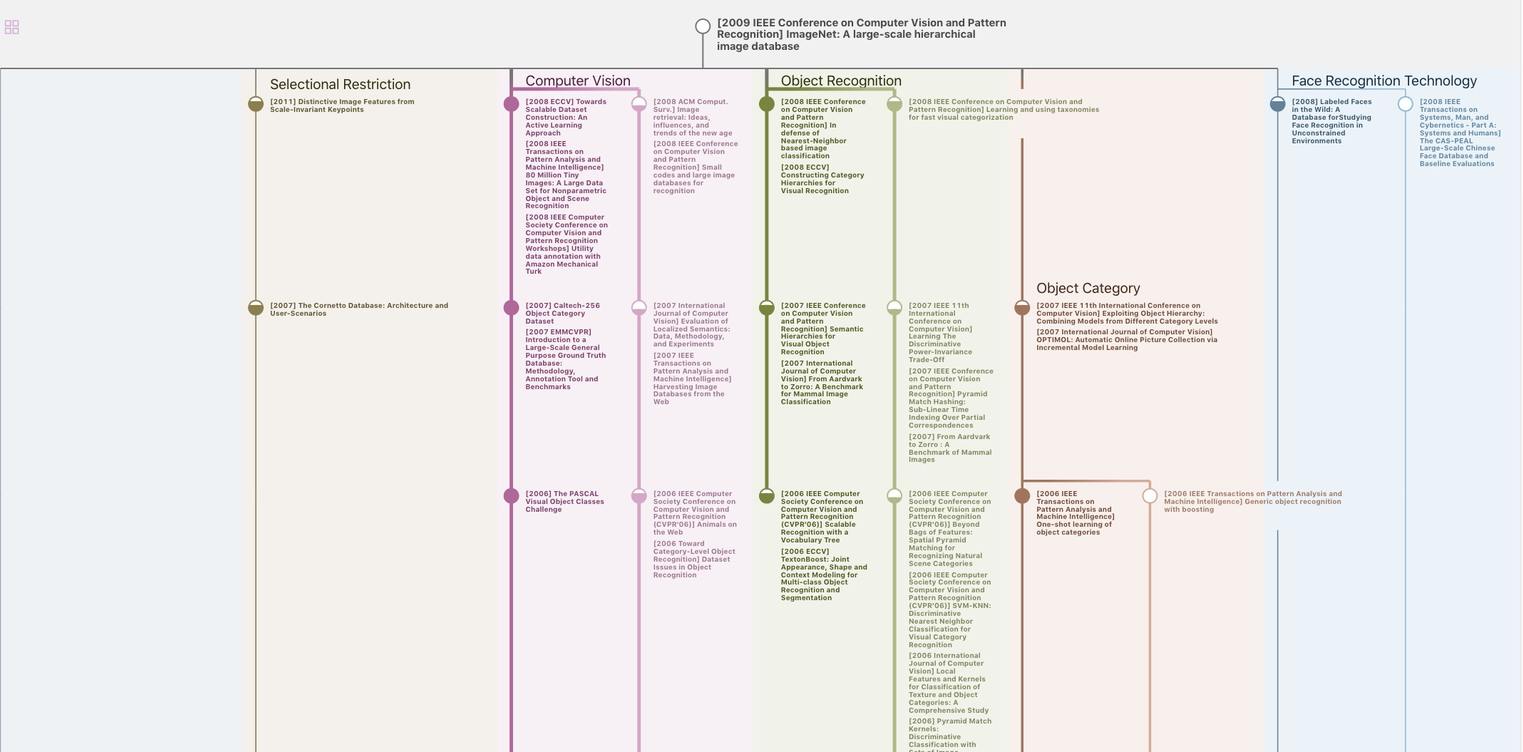
生成溯源树,研究论文发展脉络
Chat Paper
正在生成论文摘要