RiskNet: Neural Risk Assessment in Networks of Unreliable Resources
arxiv(2023)
摘要
We propose a graph neural network (GNN)-based method to predict the distribution of penalties induced by outages in communication networks, where connections are protected by resources shared between working and backup paths. The GNN-based algorithm is trained only with random graphs generated on the basis of the Barabási–Albert model. However, the results obtained show that we can accurately model the penalties in a wide range of existing topologies. We show that GNNs eliminate the need to simulate complex outage scenarios for the network topologies under study—in practice, the entire time of path placement evaluation based on the prediction is no longer than 4 ms on modern hardware. In this way, we gain up to 12 000 times in speed improvement compared to calculations based on simulations.
更多查看译文
关键词
Graph neural networks (GNNs),Message-passing neural networks (MPNN),Resilience management,Risk engineering,Shared protection,Value-at-risk ( VaR )
AI 理解论文
溯源树
样例
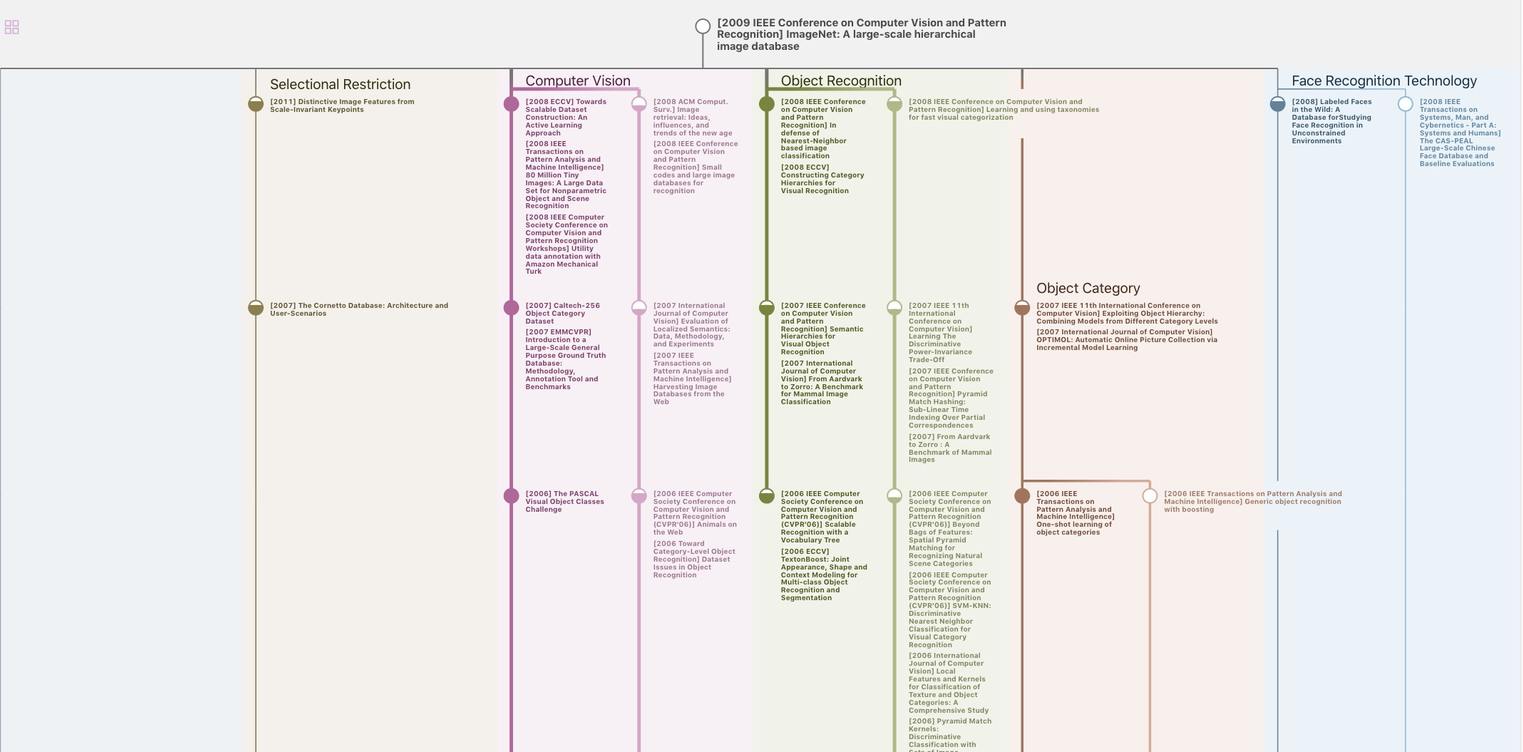
生成溯源树,研究论文发展脉络
Chat Paper
正在生成论文摘要