Generalized statistics: Applications to data inverse problems with outlier-resistance
arxiv(2023)
摘要
The conventional approach to data-driven inversion framework is based on Gaussian statistics that presents serious difficulties, especially in the presence of outliers in the measurements. In this work, we present maximum likelihood estimators associated with generalized Gaussian distributions in the context of Renyi, Tsallis and Kaniadakis statistics. In this regard, we analytically analyze the outlier-resistance of each proposal through the so-called influence function. In this way, we formulate inverse problems by constructing objective functions linked to the maximum likelihood estimators. To demonstrate the robustness of the generalized methodologies, we consider an important geophysical inverse problem with high noisy data with spikes. The results reveal that the best data inversion performance occurs when the entropic index from each generalized statistic is associated with objective functions proportional to the inverse of the error amplitude. We argue that in such a limit the three approaches are resistant to outliers and are also equivalent, which suggests a lower computational cost for the inversion process due to the reduction of numerical simulations to be performed and the fast convergence of the optimization process.
更多查看译文
关键词
data inverse problems,generalized statistics,outlier-resistance
AI 理解论文
溯源树
样例
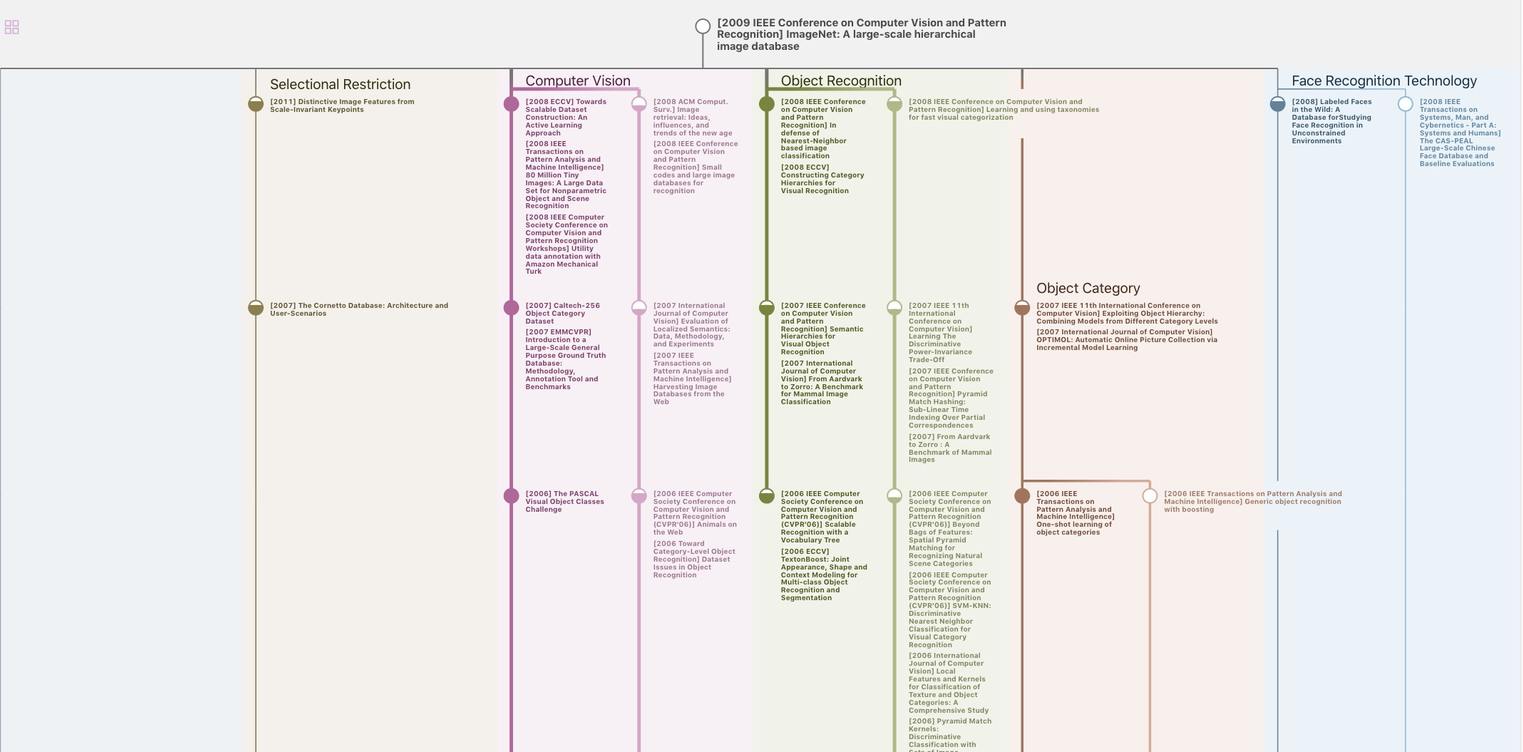
生成溯源树,研究论文发展脉络
Chat Paper
正在生成论文摘要