Deep machine learning potential for atomistic simulation of Fe-Si-O systems under Earth's outer core conditions
PHYSICAL REVIEW MATERIALS(2022)
摘要
Using artificial neural-network machine learning (ANN-ML) to generate interatomic potentials has been demonstrated to be a promising approach to address the longstanding challenge of accuracy vs efficiency in molecular dynamics (MD) simulations. Here, taking the Fe-Si-O system as a prototype, we show that accurate and transferable ANN-ML potentials can be developed for reliable MD simulations of materials at high-pressure and high-temperature conditions of the Earth's outer core. The ANN-ML potential for the Fe-Si-O system is trained by fitting the energies and forces of related binaries and ternary liquid structures at high pressures and temperatures obtained by first-principles calculations based on density functional theory (DFT). We show that the generated ANN-ML potential describes well the structure and dynamics of liquid phases of this complex system. In addition to binary systems (Fe189Si61, Fe189O61, and Si80O160) and ternary systems (Fe189Si38O23), whose snapshots are included in the training dataset, the reliability of the ANN-ML potential is validated in two other ternary systems (Fe189Si23O38 and Fe158Si14O28), whose snapshots are not included in the training dataset. The efficient ANN-ML potential with DFT accuracy provides a promising scheme for accurate atomistic simulations of structures and dynamics of the complex Fe-Si-O system in the Earth's outer core.
更多查看译文
关键词
atomistic simulation,deep machine,core,fe-si-o
AI 理解论文
溯源树
样例
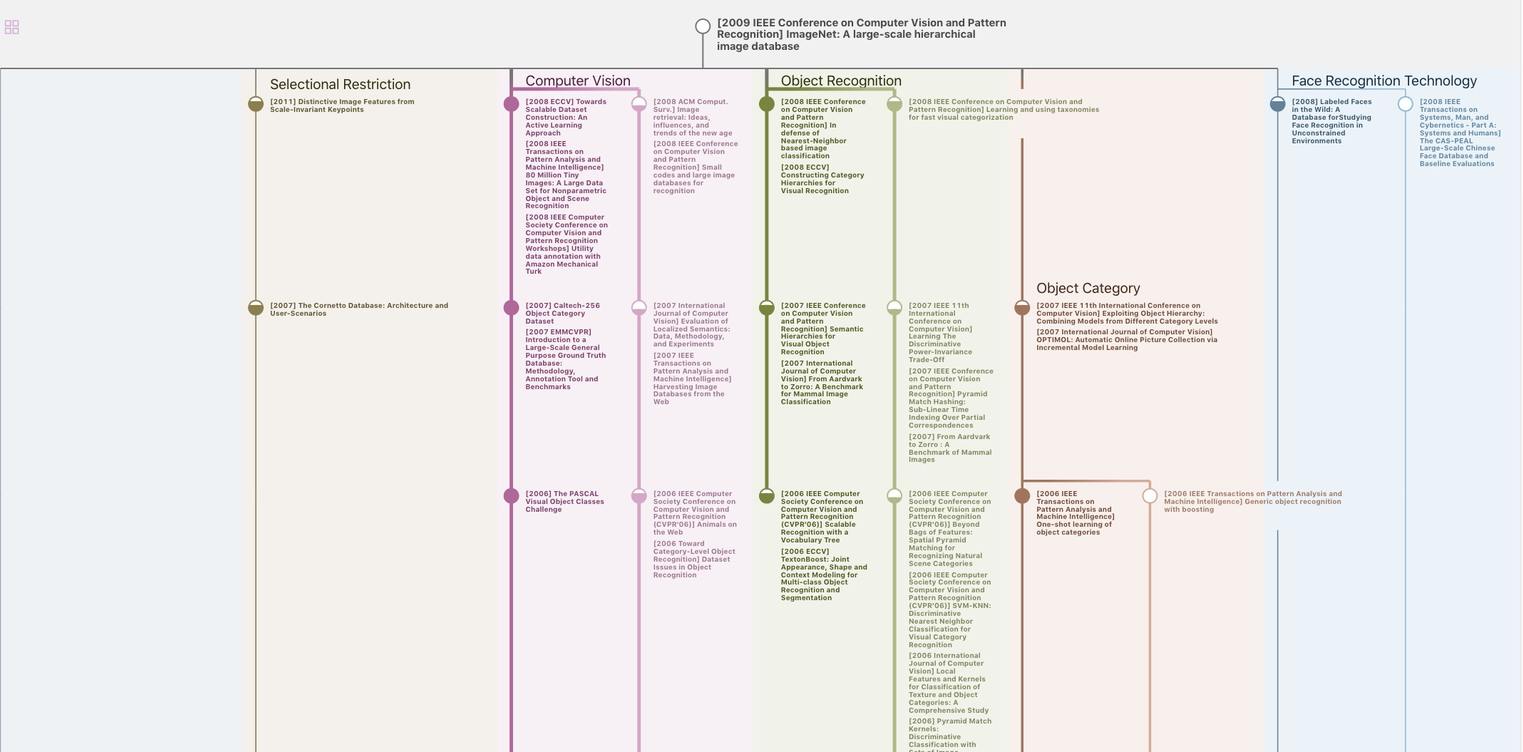
生成溯源树,研究论文发展脉络
Chat Paper
正在生成论文摘要