Comparison of an in-house hybrid DIR method to NiftyReg on CBCT and CT images for head and neck cancer
JOURNAL OF APPLIED CLINICAL MEDICAL PHYSICS(2022)
摘要
An in-house hybrid deformable image registration (DIR) method, which combines free-form deformation (FFD) and the viscous fluid registration method, is proposed. Its results on the planning computed tomography (CT) and the day 1 treatment cone-beam CT (CBCT) image from 68 head and neck cancer patients are compared with the results of NiftyReg, which uses B-spline FFD alone. Several similarity metrics, the target registration error (TRE) of annotated points, as well as the Dice similarity coefficient (DSC) and Hausdorff distance (HD) of the propagated organs at risk are employed to analyze their registration accuracy. According to quantitative analysis on mutual information, normalized cross-correlation, and the absolute pixel value differences, the results of the proposed DIR are more similar to the CBCT images than the NiftyReg results. Smaller TRE of the annotated points is observed in the proposed method, and the overall mean TRE for the proposed method and NiftyReg was 2.34 and 2.98 mm, respectively (p < 0.001). The mean DSC in the larynx, spinal cord, oral cavity, mandible, and parotid given by the proposed method ranged from 0.78 to 0.91, significantly higher than the NiftyReg results (ranging from 0.77 to 0.90), and the HD was significantly lower compared to NiftyReg. Furthermore, the proposed method did not suffer from unrealistic deformations as the NiftyReg did in the visual evaluation. Meanwhile, the execution time of the proposed method was much higher than NiftyReg (96.98 +/- 11.88 s vs. 4.60 +/- 0.49 s). In conclusion, the in-house hybrid method gave better accuracy and more stable performance than NiftyReg.
更多查看译文
关键词
cone-beam CT, deformable image registration, Dice similarity coefficient, free-form registration, head and neck cancer, similarity metric, viscous fluid registration
AI 理解论文
溯源树
样例
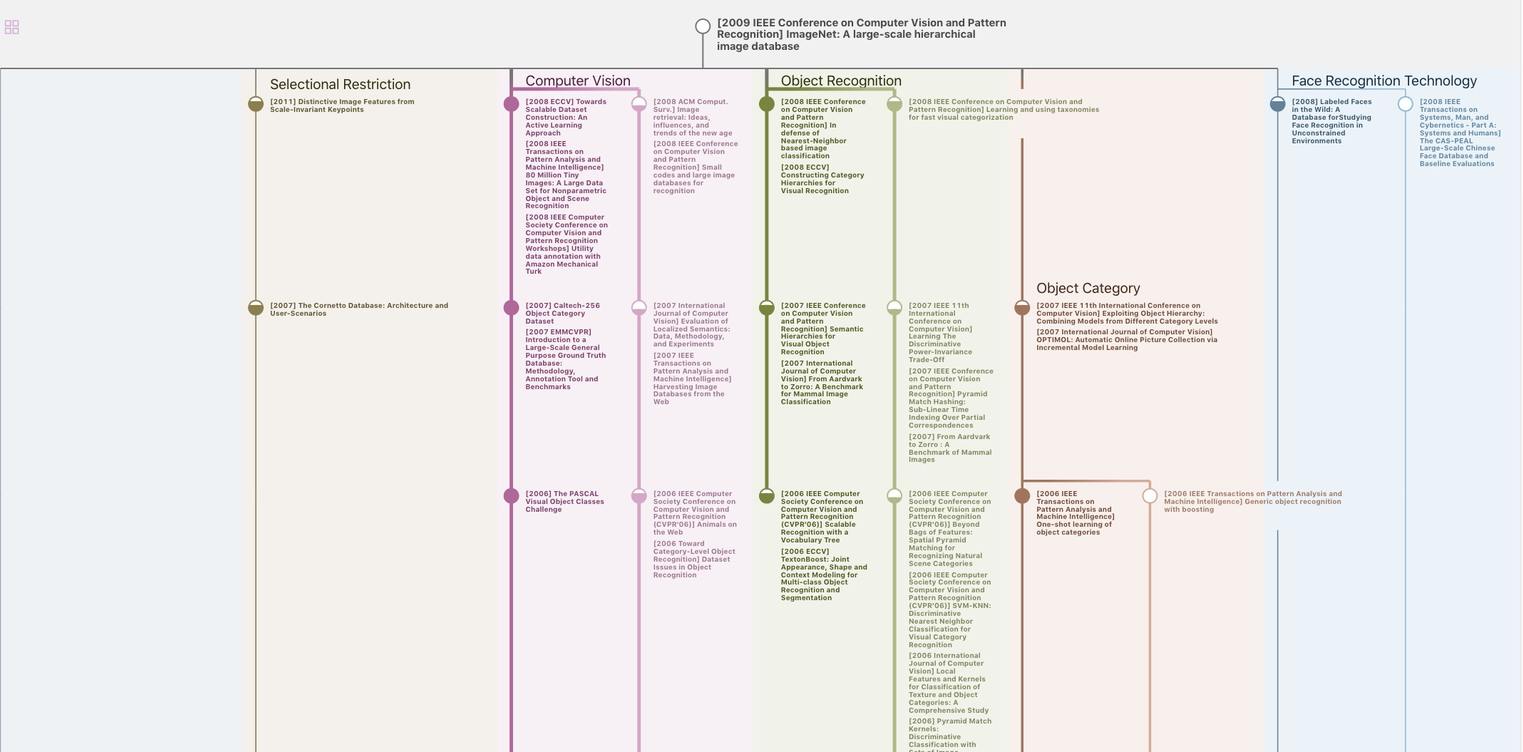
生成溯源树,研究论文发展脉络
Chat Paper
正在生成论文摘要