Development of a deep learning-based patient-specific target contour prediction model for markerless tumor positioning
MEDICAL PHYSICS(2022)
摘要
Purpose For pancreatic cancer patients, image guided radiation therapy and real-time tumor tracking (RTTT) techniques can deliver radiation to the target accurately. Currently, for the radiation therapy machine with kV X-ray imaging systems, internal markers must be implemented to facilitate tumor tracking. The purpose of this study was to develop a markerless deep learning-based pancreatic tumor positioning procedure for real-time tumor tracking with a kV X-ray imaging system. Methods and materials Fourteen pancreatic cancer patients treated with intensity-modulated radiation therapy from six fixed gantry angles with a gimbal-head radiotherapy system were included in this study. For a gimbal-head radiotherapy system, the three-dimensional (3D) intrafraction target position can be determined using an orthogonal kV X-ray imaging system. All patients underwent four-dimensional computed tomography (4DCT) simulations for treatment planning, which were divided into 10 respiratory phases. After a patient's 4DCT was acquired, for each X-ray tube angle, 10 digitally reconstructed radiograph (DRR) images were obtained. Then, a data augmentation procedure was conducted. The data augmentation procedure first rotated the CT volume around the superior-inferior and anterior-posterior directions from -3 degrees to 3 degrees in 1.5 degrees intervals. Then, the Super-SloMo model was adapted to interpolate 10 frames between respiratory phases. In total, the data augmentation procedure expanded the data scale 250-fold. In this study, for each patient, 12 datasets containing the DRR images from each specific X-ray tube angle based on the radiation therapy plan were obtained. The augmented dataset was randomly divided into training and testing datasets. The training dataset contained 2000 DRR images with clinical target volume (CTV) contours labeled for fine-tuning the pre-trained target contour prediction model. After the fine-tuning, the patient and X-ray tube angle-specific CTV contour prediction model was acquired. The testing dataset contained the remaining 500 images to evaluate the performance of the CTV contour prediction model. The dice similarity coefficient (DSC) between the area enclosed by the CTV contour and predicted contour was calculated to evaluate the model's contour prediction performance. The 3D position of the CTV was calculated based on the centroid of the contour in the orthogonal DRR images, and the 3D error of the prediction position was calculated to evaluate the CTV positioning performance. For each patient, the DSC results from 12 X-ray tube angles and 3D error from 6 gantry angles were calculated, representing the novelty of this study. Results The mean and standard deviation (SD) of all patients' DSCs were 0.98 and 0.015, respectively. The mean and SD of the 3D error were 0.29 mm and 0.14 mm, respectively. The global maximum 3D error was 1.66 mm, and the global minimum DSC was 0.81. The mean calculation time for CTV contour prediction was 55 ms per image. This fulfills the requirement of RTTT. Conclusions Regarding the positioning accuracy and calculation efficiency, the presented procedure can provide a solution for markerless real-time tumor tracking for pancreatic cancer patients.
更多查看译文
关键词
deep learning, markerless tumor positioning, pancreatic cancer, real time tumor tracking, target contour prediction
AI 理解论文
溯源树
样例
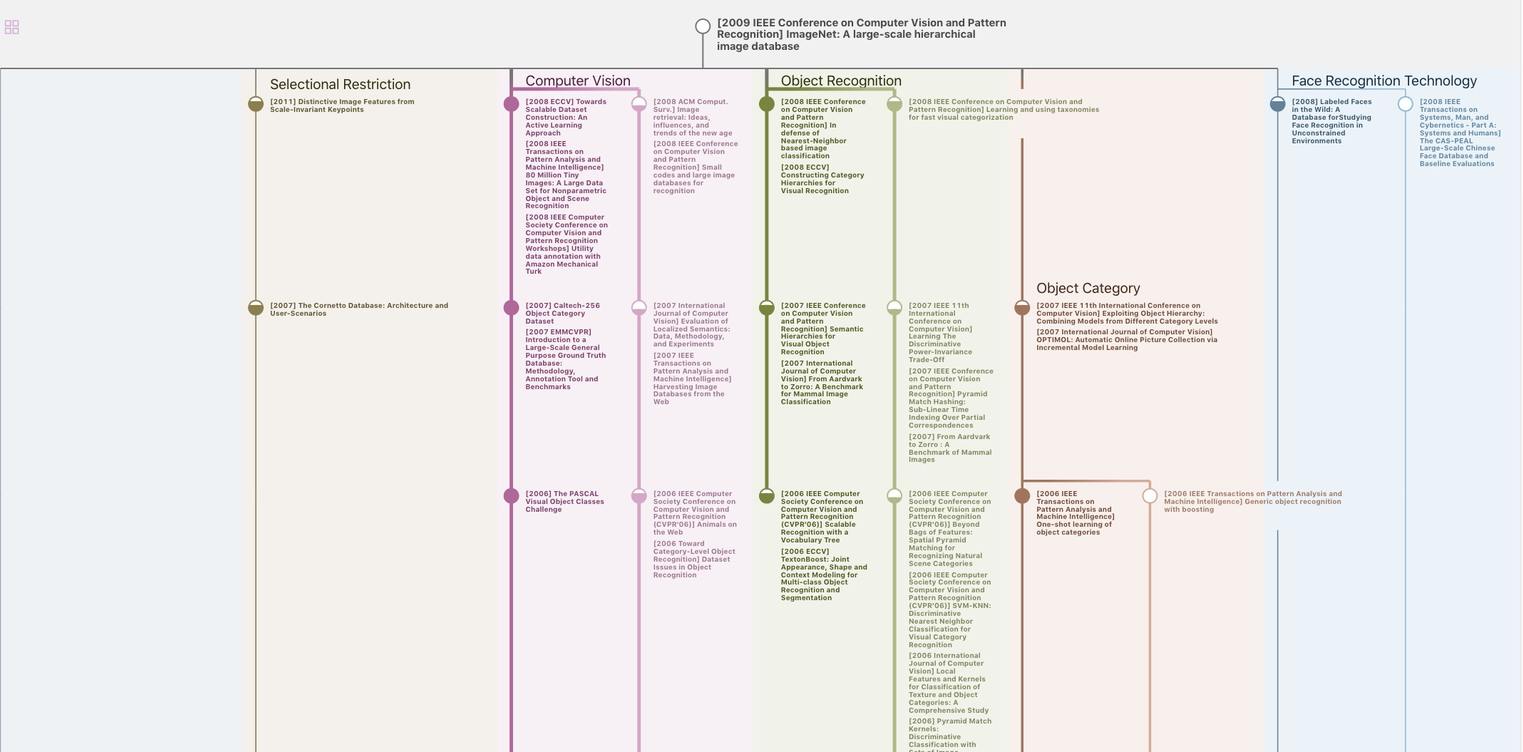
生成溯源树,研究论文发展脉络
Chat Paper
正在生成论文摘要