Ontology-enhanced Prompt-tuning for Few-shot Learning
International World Wide Web Conference(2022)
Abstract
ABSTRACT Few-shot Learning (FSL) is aimed to make predictions based on a limited number of samples. Structured data such as knowledge graphs and ontology libraries has been leveraged to benefit the few-shot setting in various tasks. However, the priors adopted by the existing methods suffer from challenging knowledge missing, knowledge noise, and knowledge heterogeneity, which hinder the performance for few-shot learning. In this study, we explore knowledge injection for FSL with pre-trained language models and propose ontology-enhanced prompt-tuning (OntoPrompt). Specifically, we develop the ontology transformation based on the external knowledge graph to address the knowledge missing issue, which fulfills and converts structure knowledge to text. We further introduce span-sensitive knowledge injection via a visible matrix to select informative knowledge to handle the knowledge noise issue. To bridge the gap between knowledge and text, we propose a collective training algorithm to optimize representations jointly. We evaluate our proposed OntoPrompt in three tasks, including relation extraction, event extraction, and knowledge graph completion, with eight datasets. Experimental results demonstrate that our approach can obtain better few-shot performance than baselines.
MoreTranslated text
Key words
Few-shot Learning, Ontology, Prompt-tuning, Relation Extraction, Event Extraction, Knowledge Graph Completion
AI Read Science
Must-Reading Tree
Example
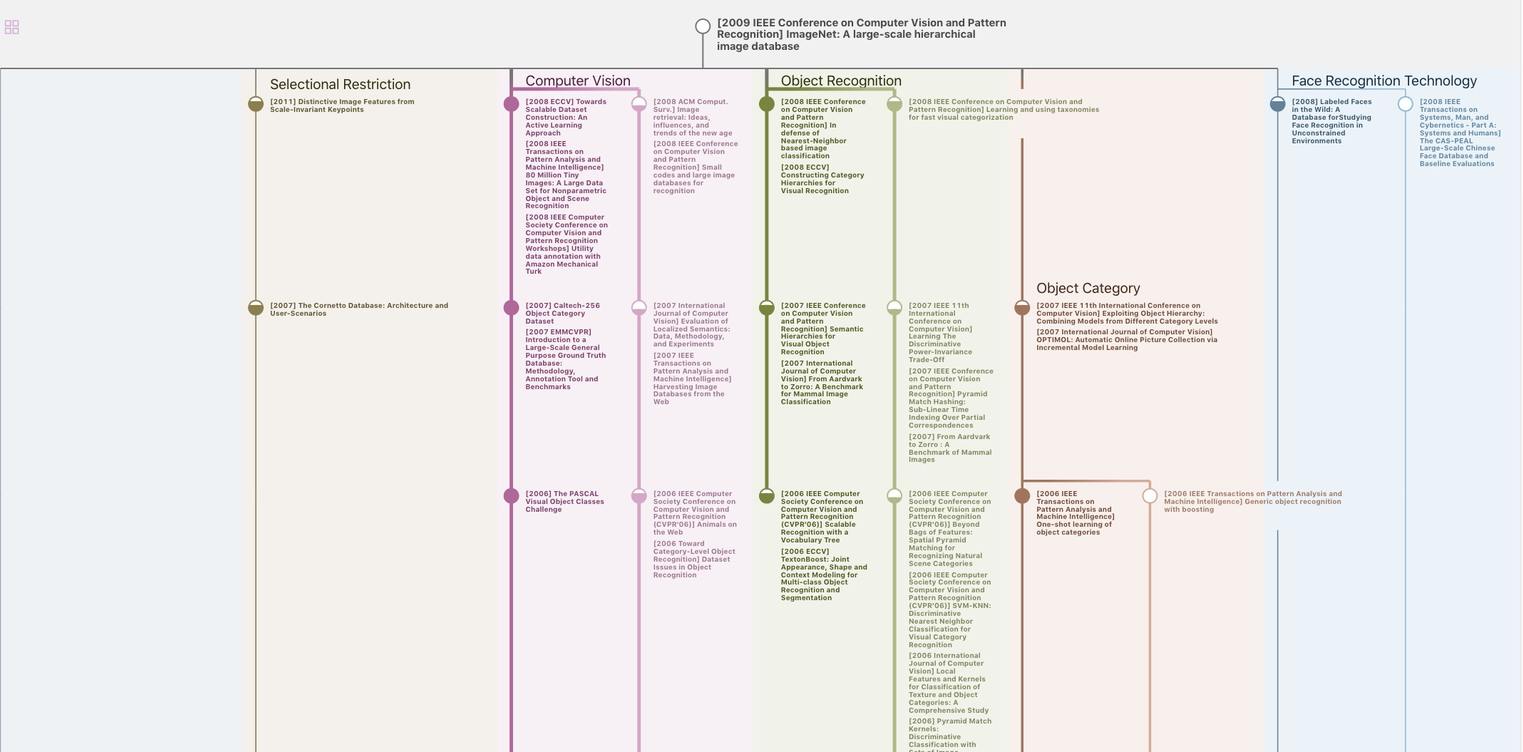
Generate MRT to find the research sequence of this paper
Chat Paper
Summary is being generated by the instructions you defined