Machine-learning of nonlocal kernels for anomalous subsurface transport from breakthrough curves
NUMERICAL ALGEBRA CONTROL AND OPTIMIZATION(2024)
摘要
Anomalous behavior is ubiquitous in subsurface solute transport due to the presence of high degrees of heterogeneity at different scales in the media. Although fractional models have been extensively used to describe the anomalous transport in various subsurface applications, their application is hindered by computational challenges. Simpler nonlocal models characterized by integrable kernels and finite interaction length represent a computationally feasible alternative to fractional models; yet, the informed choice of their kernel functions still remains an open problem. We propose a general datadriven framework for the discovery of optimal kernels on the basis of very small and sparse data sets in the context of anomalous subsurface transport. Using spatially sparse breakthrough curves recovered from fine-scale particle-density simulations, we learn the best coarse-scale nonlocal model using a nonlocal operator regression technique. Predictions of the breakthrough curves obtained using the optimal nonlocal model show good agreement with fine-scale simulation results even at locations and time intervals different from the ones used to train the kernel, confirming the excellent generalization properties of the proposed algorithm. A comparison with trained classical models and with black-box deep neural networks confirms the superiority of the predictive capability of the proposed model.
更多查看译文
关键词
Anomalous transport,nonlocal diffusion,machine learning,homogenization,breakthrough curves
AI 理解论文
溯源树
样例
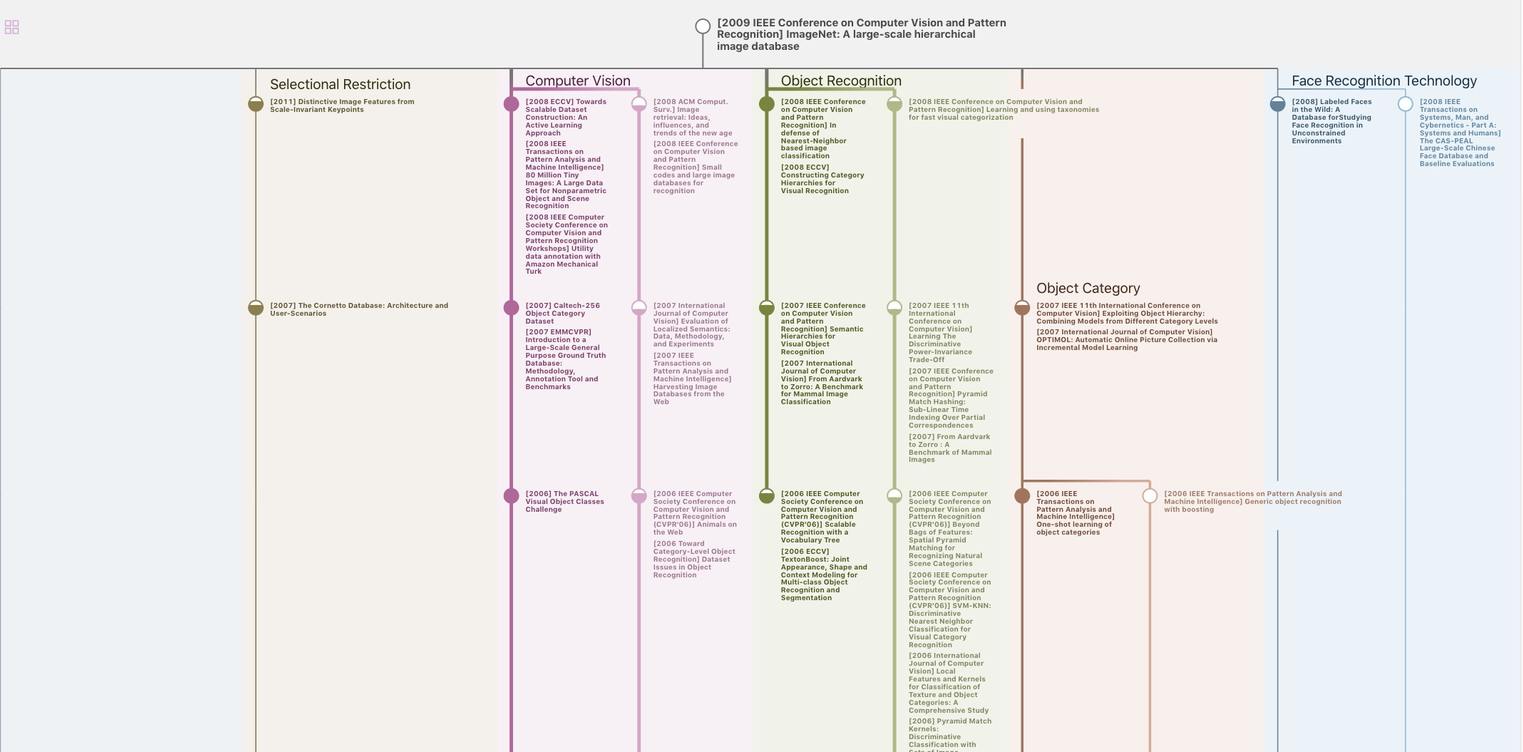
生成溯源树,研究论文发展脉络
Chat Paper
正在生成论文摘要