Covid-19 Impact and Implications on Traffic - Smart Predictive Analytics for Mobility Navigation.
COMSNETS(2022)
摘要
Traffic prediction and analysis is an essential task towards intelligent mobility, particularly for path planning and navigation. When the traffic flow starts after the COVID-19 pandemic is subsided, the mobility patterns changes and may become unpredictable or challenging. This problem may be crucial, particularly if many people hurry to single occupancy transport mode. Notably, the rapid development in machine learning with new methods and the emergence of new data sources make it possible to evaluate and predict traffic conditions in smart cities more quickly and precisely. The proposed work is modeled in two-fold manner to investigate the impact of COVID shift in regular urban traffic movements given the particular period of the pre, during, and post lockdown phases. Firstly, the investigation is carried out for time series analysis considering the three phases of lockdown. Secondly, the real-time spatial information is analyzed for different time zones in a day. Notably, this requires a detailed analysis of the heterogeneous and complex input traffic data. Machine learning and advanced deep learning methodologies such as regression models, RNN, variants of LSTM, and GRU is used for analysis in this proposed traffic modeling. Significantly, the least error scores with Root Mean Square Error (RMSE) loss of 1.82 is observed for the RNN and GRU models, and 0.058 with the Gradient Boosting regression analysis, respectively.
更多查看译文
关键词
Covid-19,Deep Learning,LSTM,Machine Learning,Regression Analysis,RNN,Smart Mobility,Traffic prediction
AI 理解论文
溯源树
样例
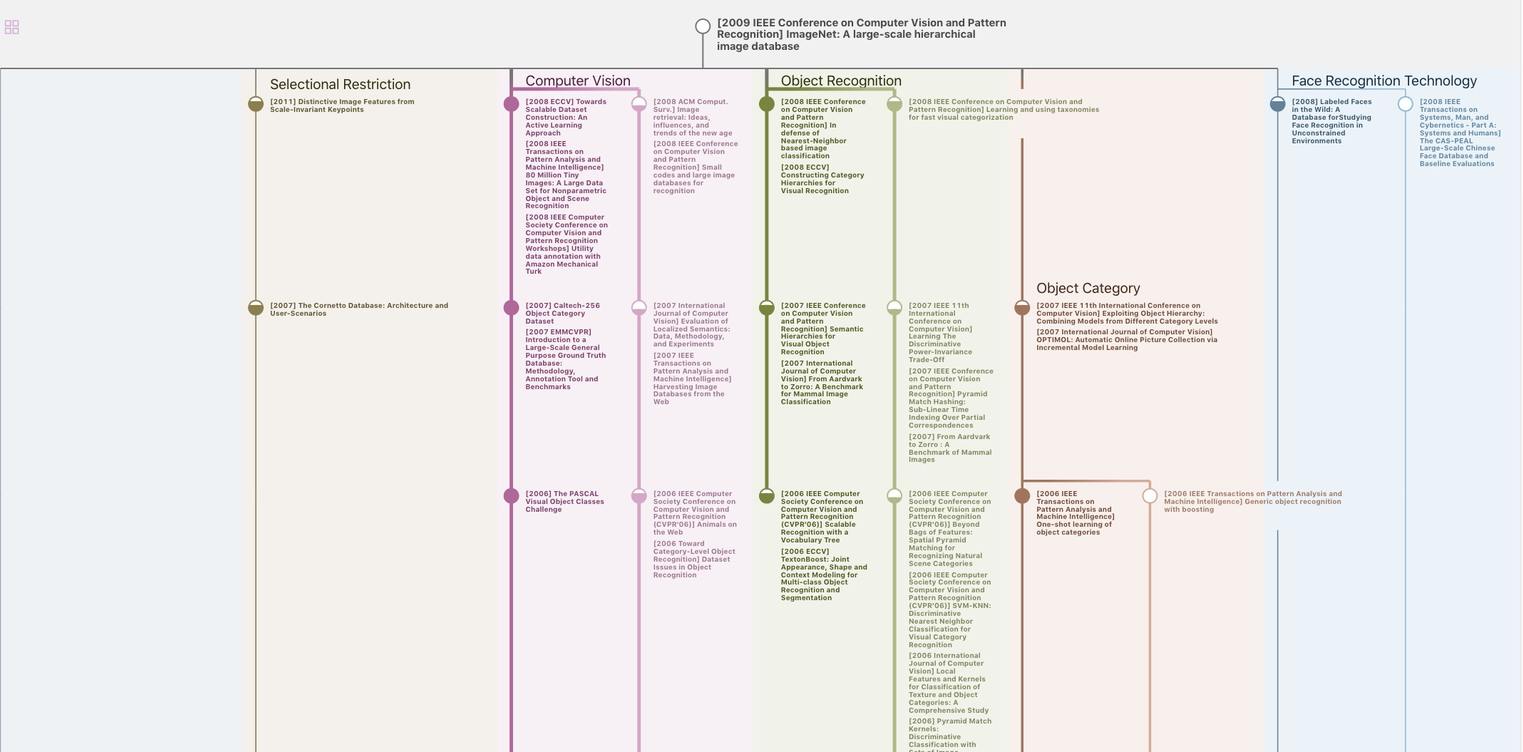
生成溯源树,研究论文发展脉络
Chat Paper
正在生成论文摘要