Residual-guided In-loop Filter Using Convolution Neural Network
ACM Transactions on Multimedia Computing, Communications, and Applications(2021)
摘要
AbstractThe block-based coding structure in the hybrid video coding framework inevitably introduces compression artifacts such as blocking, ringing, and so on. To compensate for those artifacts, extensive filtering techniques were proposed in the loop of video codecs, which are capable of boosting the subjective and objective qualities of reconstructed videos. Recently, neural network-based filters were presented with the power of deep learning from a large magnitude of data. Though the coding efficiency has been improved from traditional methods in High-Efficiency Video Coding (HEVC), the rich features and information generated by the compression pipeline have not been fully utilized in the design of neural networks. Therefore, in this article, we propose the Residual-Reconstruction-based Convolutional Neural Network (RRNet) to further improve the coding efficiency to its full extent, where the compression features induced from bitstream in form of prediction residual are fed into the network as an additional input to the reconstructed frame. In essence, the residual signal can provide valuable information about block partitions and can aid reconstruction of edge and texture regions in a picture. Thus, more adaptive parameters can be trained to handle different texture characteristics. The experimental results show that our proposed RRNet approach presents significant BD-rate savings compared to HEVC and the state-of-the-art CNN-based schemes, indicating that residual signal plays a significant role in enhancing video frame reconstruction.
更多查看译文
关键词
Convolutional neural network, high efficiency video coding, in-loop filter, reconstruction, residual
AI 理解论文
溯源树
样例
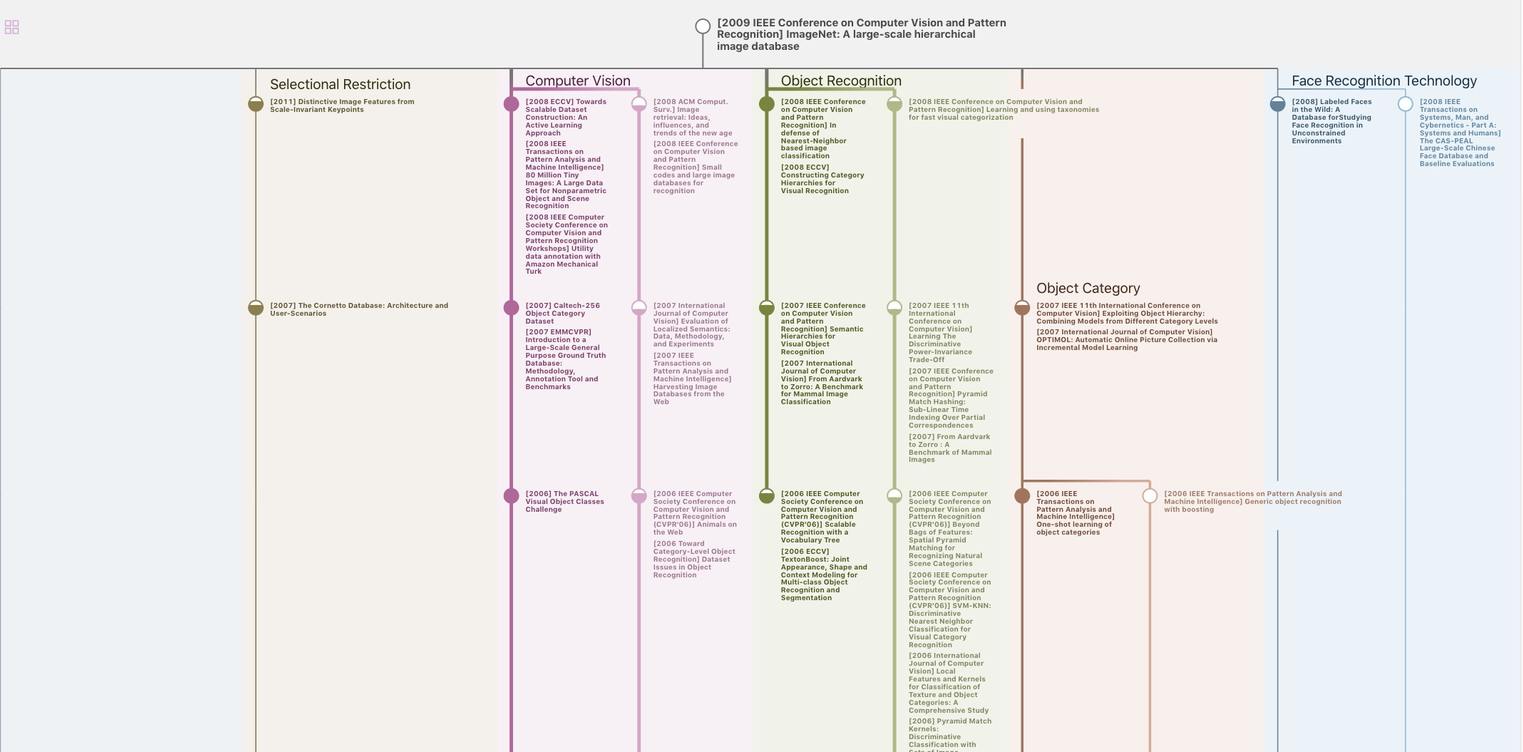
生成溯源树,研究论文发展脉络
Chat Paper
正在生成论文摘要