DDNet: 3D densely connected convolutional networks with feature pyramids for nasopharyngeal carcinoma segmentation
IET IMAGE PROCESSING(2022)
摘要
Radiation therapy is the standard treatment for early stage Nasopharyngeal cancer (NPC). Thus, accurate delineation of target volumes at risk in NPC is important. While manual delineation is time-consuming and labour-intensive process and also leads to significant inter- and intra-practitioner variability. Thus, computer-aided segmentation algorithm is required. However, segmentation task is not trivial due to large variations (e.g., shape and size) of nasopharynx structure across subjects. Moreover, extreme foreground and background class imbalance in NPC segmentation remains challenge. In this paper, we propose a threedimensional densely connected convolutional neural network with multi-scale feature pyramids for NPC segmentation. We adapt the densely connected convolutional block into a new structure via adding feature pyramids. The concatenated pyramid feature carries multi-scale and hierarchical semantic information which is effective for segmenting different size of tumors and perceiving hierarchical context information. To address the foreground and background imbalance problem, we propose an enhanced version of focal loss. It prevents the large number of negative voxels far from boundaries from overwhelming the segmentation algorithm. We validated the proposed method on 120 clinical subjects. Experimental results demonstrate that our approach out-performed state-of-the-art methods and human experts.
更多查看译文
关键词
Probability theory, stochastic processes, and statistics,Optical, image and video signal processing,Radiation therapy,Computer vision and image processing techniques,Other topics in statistics,Other topics in statistics
AI 理解论文
溯源树
样例
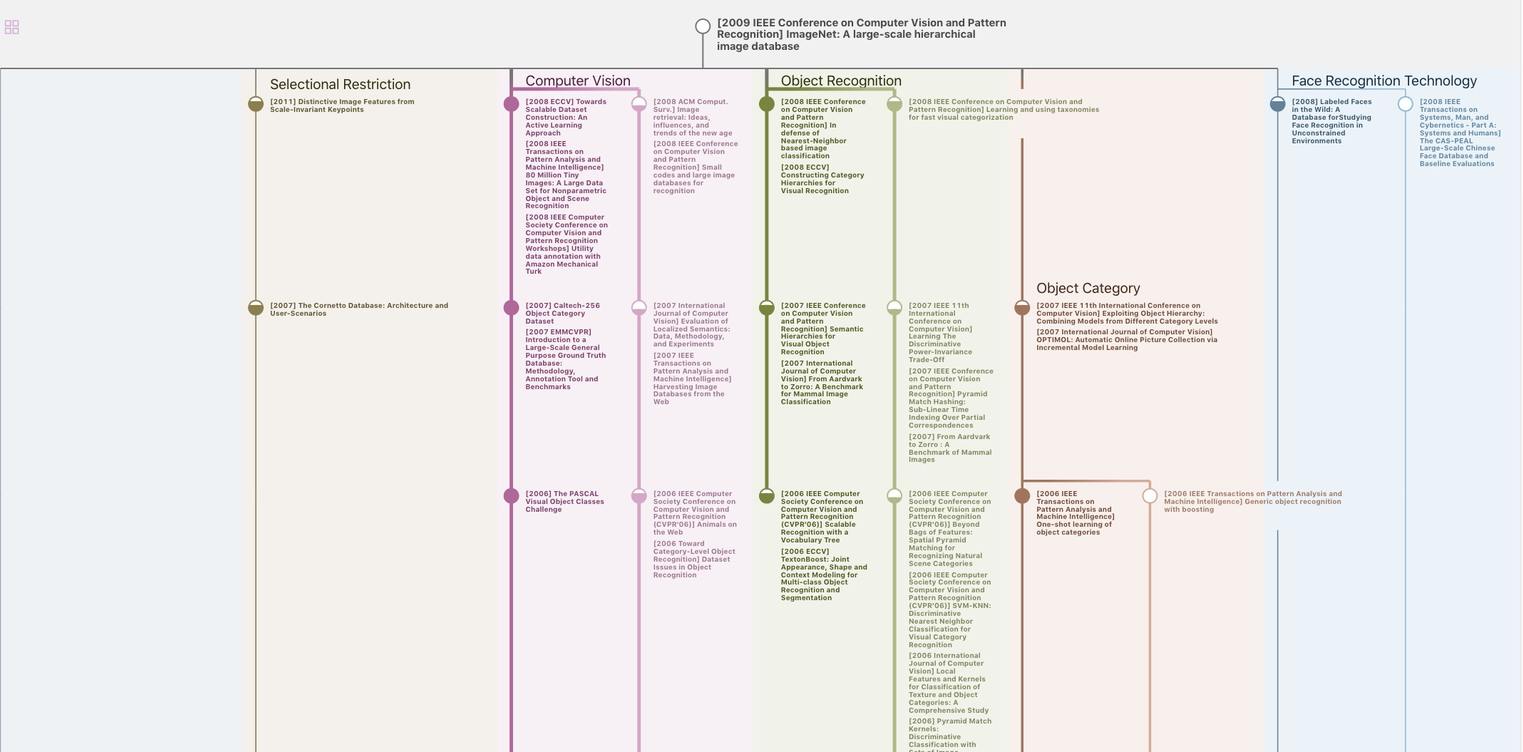
生成溯源树,研究论文发展脉络
Chat Paper
正在生成论文摘要